基于粒子群改进BP神经网络的组合预测模型及其应用
崔吉峰1, 2,乞建勋1,杨尚东3
( 华北电力大学 工商管理学院,北京,102206;
2. 国网运行有限公司,北京,100005;
3. 国网北京经济技术研究院,北京,100761)
摘 要:针对应用广泛的传统人工智能预测BP(Back propagation)神经网络自身局限以及其在处理中长期复杂预测问题中需要样本数量大、泛化能力弱等不足,提出利用粒子群算法优化BP神经网络的学习算法,在此基础上,利用灰色预测方法和自回归移动平均模型(ARIMA)时序预测对历史数据进行初步预测,对中长期预测中数据趋势项和随机项进行模拟;将初步预测的结果作为改进BP神经网络的输入,在此基础上进行训练和预测,构建基于改进BP网络的组合预测模型。以我国1978—2007年能源需求数据为样本,进行实例分析。结果表明:组合预测模型预测精度较BP神经网络、灰色预测方法和ARIMA预测方法分别提高4.8%,6.1%和5.3%,验证了组合预测方法在中长期预测问题处理中的有效性。
关键词:BP神经网络;粒子群算法;ARIMA模型;灰色理论;组合预测
中图分类号:TP18 文献标识码:A 文章编号:1672-7207(2009)01-0190-05
Combined forecasting model based on BP improved by
PSO and its application
CUI Ji-feng1, 2, QI Jian-xun1, YANG Shang-dong3
( School of Business Administration, North China Electric Power University, Beijing 102206, China;
2. State Grid Operation Company Ltd., Beijing 100005, China;
3. State Power Economic Research Institute, Beijing 100761, China)
Abstract: To solve the limitations of BP neural network in dealing with complex and long-term prediction, which needs many samples to calculate and its generalization ability is poor, the learning algorithm of BP neural network optimized by PSO (Particle swarm algorithm) was proposed. On the basis of this algorithm, the grey prediction and the ARIMA (Autoregressive integrated moving average model) time series forecasting methods were used to make a preliminary forecast for historical data. The results of initial forecasts were put as the input of improved BP neural network to be forecasted and trained. An improved BP network-based combining forecasting model was built. China’s energy demands data in 1978—2007 were taken as the sample to be analyzed. The results show that the learning algorithm of BP neural network optimized by PSO has better effects in simulation of the trend data and the random data in medium- and long- term forecasting. The prediction accuracy of combining forecasting model increases by 4.8%, 6.1% and 5.3% respectively compared with those of BP neural network, gray forecasting methods and ARIMA forecasting, which proves the effectiveness of combining forecasting method in the medium-term and long-term forecasting.
Key words: BP neural network; particle swarm optimization; auto regression-moving average; grey model; combining forecasting
随着计算机科学的进展,越来越多的研究者开始探讨利用人工智能预测方法进行中长期负荷预测。中长期负荷预测问题,大多因为预测周期长和影响因素复杂,一直未能得到有效解决[1]。传统预测方法如趋势预测、灰色预测、时间序列预测方法,对于中长期预测的周期性因素有较强的处理能力[2]。人工智能预测方法如BP神经网络、支持向量机[3-4],对于复杂因素具有较强的拟合能力,但是,对于不同结构的数据样本,预测的差异性较大,预测效果的稳定性有待提高[5-6]。在此,本文作者提出粒子群算法优化BP(Back propagation)神经网络的学习算法。在此基础上,利用灰色预测方法和ARIMA(Autoregressive integrated moving average model)时序预测对历史数据进行初步预测。其中,灰色预测有较强的趋势项模拟能力,ARIMA时序模型用于非平稳数据建模能够较好地处理随即扰动因素。将灰色预测和ARIMA时序预测的结果作为改进BP神经网络的输入,从而构建组合预测模型,对中长期预测中数据趋势项和随机项进行模拟,将初步预测结果作为改进BP神经网络的输入,在此基础上进行训练和预测,构建基于改进BP网络的组合预测模型。此外,将人工神经网络预测组合模型应用于中长期能源需求问题,结合我国1978—2007年能源需求以及GDP、人口等相关因素的实际数据进行实证研究。
1 灰色预测模型
灰色系统理论[7-8]是基于小样本的灰信息(信息不完全、不充分与非惟一)系统理论,对于因素空间难以穷尽、运行机制尚不明确又缺乏建立确定关系的信息系统等问题,该理论及方法提供了一种新的解决方法。它由灰信息论、灰集合论和灰方法论3部分组成。GM(1, 1)模型法是该理论中的重要方法之一。建立GM(1, 1)模型的实质是对原始序列进行1次累加,使生成序列呈现一定规律,然后,建立一阶线性微分方程模型,求得拟合曲线对系统进行预测。灰色GM(1, 1)预测模型在计算过程中是以计算矩阵为主,它与MATLAB结合可以有效地解决灰色系统理论在矩阵计算中的问题,能够适用于数据样本较小的预测问题。
GM(1,1)模型是最常用的一种灰色模型,它是由一个只包含单变量的一阶微分方程构成的模型。作为电力负荷预测的一种有效的模型,使用灰色理论进行预测。
设有变量为
的原始数据序列
,用1-AGO生成一阶累加生成序列
。其中,
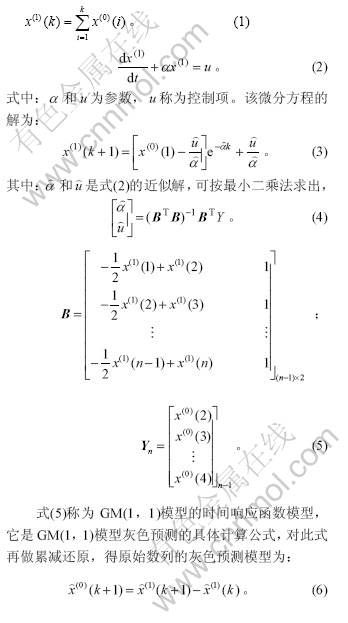
2 时间序列预测
时间序列分析方法[9]就是从具有先后顺序的信息中提取有用信息,它是数理统计的一个重要分支。时间序列分析方法的实质是通过对历史数据的处理,寻找前后数据之间的关系,建立关联模型,然后,通过历史数据和所建立的关联模型预测时间序列的未来值。时间序列分析有多种模型:自回归模型(Autoregressive model,AR)、移动平均模型(Moving average model,MA)、自回归移动平均模型(Auto-regressive moving average model,ARMA),这3种模型都是对平稳时间序列而言。在实际问题中,许多时间序列并不近似为平稳时间序列,所以不能直接用3种基本模型建模,可以通过某种处理产生一个平稳的新的时间序列,再进行建模。ARIMA(p,d,q)中:p代表自回归阶数;d代表对含有长期趋势、季节变动、循环变动的非平稳时间数列进行差分处理的次数;q代表滑动平均的阶数。本研究就是应用ARIMA模型(自回归移动平均模型)进行预测分析。
ARIMA建模基本思路为:将预测对象随时间移而形成的数据序列视为一个随机序列,用一定的数学模型来近似描述这个序列,模型通过识别后就可以从时间序列的过去值和现在值来预测未来值。
3 粒子群算法改进BP神经网络
3.1 基本原理
BP网络是一种按误差逆传播算法训练的多层前馈网络。它能学习和存贮大量的输入-输出模式映射关系,而无需事前揭示描述这种映射关系的数学方程。它的学习规则是使用最速下降法,通过反向传播不断调整网络的权值和阈值,使网络的误差平方和最小。BP神经网络模型拓扑结构包括输入层(input)、隐层(hide layer)和输出层(output layer)[10-11]。
3.2 粒子群优化算法
PSO算法是一种基于群体的优化方法,算法中种群称作粒子群,个体称为粒子。它的基本概念源于对海鸥等海鸟捕食方式的模拟。一群海鸟随机地搜寻食物,它们不知道食物在哪里,但知道当前离食物最近的位置,从而选择搜寻目前离食物最近的鸟的周围区域,以尽快地找到食物[12-13]。
PSO算法中的粒子寻优基本公式如下:
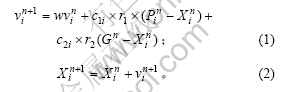
式中:w为惯性因子;r1和r2为(0,1)区间服从均匀分布的随机数;c1i和c2i为学习因子;n为迭代次数;
为迭代n次时粒子i的空间位置;
为迭代n次时粒子i的速度;
和
分别为微粒从初始到当前迭代次数搜索产生的个体极值和全局极值。
3.3 优化的神经网络学习算法
3.3.1 算法设计
将神经元之间所有的连接权值编码成实数向量,用来表示种群中的个体,随机生成这些向量的群体,按照算法原步骤迭代。迭代中新生成的个体向量还原成神经网络权值,计算所有样本通过神经网络产生的均方差,若其小于系统指定的误差,则训练过程停止,否则继续进行,直到达到最大迭代次数为止。
BP网络的激活函数都选定为Sigmoid函数,然后,用PSO算法搜索出最优位置,使如下均方误差指标达到最小:

其中:N为样本个数;c为网络神经元输出个数;tk, p为第p个样本的第k个理想输出值;Yk, p为第p个样本的第k个实际输出值。
3.3.2 算法实现步骤
a. 确定预定的误差要求、最大允许迭代步数、搜索范围、最大速度,并根据网络规模确定粒子数。
b. 根据微粒群规模,随机产生一定数目的个体Xi及其速度vi组成种群,其中不同的个体代表神经网络的一组不同权值。
c. 计算各个粒子的适应值。
d. 比较适应度,确定每个粒子的局部最优值以及每一代粒子的全部最优值G_best。
e. 检验是否符合结束条件。若符合,则停止迭代,输出神经网络的最终权值和阈值。
f. 更新每个粒子的位置以及速度,并继续迭代。
4 改进神经网络组合预测模型
三层BP神经网络如图1所示。其中:N1为输入层节点数;N2为中间层节点数;N3为输出层节点数。
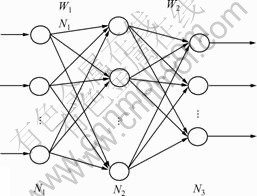
图1 三层BP神经网络
Fig.1 Three layers BP neuro network
节点的传递函数通常选取Sigmoid型函数
,其中,
为阈值。算法中的误差准则是调整权值,使得偏差
最小。
神经网络组合预测的基本方法与步骤如下。
步骤1 以能源年度需求的历史数据建立GM(1, 1)等维数为m的等维信息模型,其灰色预测值为
,以ARIMA模型建立预测模型,预测值为
。
步骤2 将ARIMA的预测值
和灰色预测值
作为用于组合预测的BP网络的输入,其对应的实际值Y(k)作为该网络的输出构成匹配对
,然后,将这些匹配对分为2部分:A部分用于网络的训练,B部分用于测试该网络的 性能。
步骤3 用粒子群算法对A部分样本进行组合预测网络BP的学习,然后,进行1步预测,从而得到能源需求的最终预测值
,如图2所示。
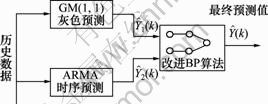
图2 改进BP神经网络组合预测模型
Fig.2 Combination forecasting model of improving BP neural network
5 改进BP神经网络组合预测方法在中长期能源需求预测中的应用
采用所提出的改进BP神经网络组合预测模型,利用我国1978—2007年全国用电量实际数据为样本进行实证分析。其中:1978—2003年数据用于训练学习,2003—2007年数据用于测试网络性能。
采用历史数据样本为GDP数据、能源需求数据、城市化率数据,分别用灰色预测和ARIMA模型进行初步预测,以初步预测值作为改进BP网络输入,进行学习训练和预测检验。
将所提出的方法分别与普通BP组合预测(未经PSO优化的普通BP网络作为组合预测实施载体)、灰色预测、ARIMA时序预测模型的预测相对误差进行比较,以验证本研究提出预测方法的有效性,比较结果如图3所示。
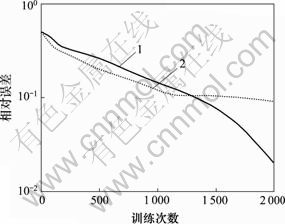
1—PSO改进BP模型;2—BP模型
图3 不同算法下相对误差与训练次数的关系
Fig.3 Relationships between relative error and training times in different algorithms
从图3可见,随着迭代次数的增加,采用PSO改进BP算法所得结果的相对误差与采用BP算法所得结果的相对误差小,同时其学习效果好,说明PSO算法改进BP网络训练机制的有效性。
采用PSO改进BP算法与BP神经网络、灰色预测方法和ARIMA预测方法所得的能源需求比较结果如图4所示。可见,采用PSO改进BP算法所得到的能源需求预测曲线与采用其他3种方法相比更逼近真实年度能源需求曲线,说明PSO改进BP算法能够更好地适应中长期能源需求预测,获得的预测效果更好。
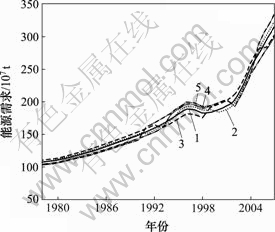
1—实际需求;2—PSO改进BP模型计算值;
3—BP模型计算值;4—灰色预测值;5—时序预测值
图4 不同方法的预测结果
Fig.4 Forecasting curves with different models
PSO改进BP算法与BP神经网络、灰色预测方法和ARIMA预测方法的预测精度比较结果如表1所示。
表1 本研究提出方法预测精度与其他方法的比较
Table 1 Comparison of forecasting accuracy with different models
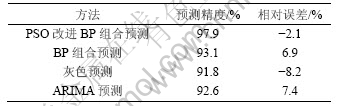
利用BP组合预测得到的预测结果的相对误差为6.9%;单独采用灰色预测模型得到的预测相对误差达到了-8.2%,是采用的几种模型中预测误差最大的;采用ARIMA预测模型得到的预测精度为92.6%,相对误差为7.4%;根据本研究采用的经过PSO改进的BP神经网络模型组合预测得到的预测精度达到了97.9%,相对误差仅为-2.1%。可见,采用PSO改进BP算法得到的预测精度与BP神经网络、灰色预测方法和ARIMA预测方法的预测精度相比分别提高了4.8%,6.1%和5.3%,由相对误差的比较可以看出这3种模型的预测精度均比较低,而采用本研究提出的PSO改进BP组合模型的预测精度得到了大大的提高。
6 结 论
a. 所提出的基于改进BP神经网络组合预测方法,利用灰色预测和ARIMA以及人工神经网络预测方法各自的优势,能够更好地协调处理好中长期预测问题中趋势性、周期性和随机性因素的影响,与单一方法相比更适合中长期预测。
b. 所提出的PSO改进BP神经网络能够有效地提高BP学习方法的全局性,克服原有学习策略下容易陷入局部极值的缺陷,学习能力提高;同时,算法的稳定性增强。改进后的BP神经网络作为组合预测的实施载体,能够更好地发挥组合预测的改进效果。
c. PSO改进BP神经网络预测方法的实证预测结果表明,该方法是有效的。
参考文献:
[1] Vila J P, Wagner V, Neveu P. Bayesian nonlinear model selection and neural network: A conjugate prior approach[J]. IEEE Trans on Neural Networks, 2000, 11(2): 265-278.
[2] Rahman M. Analysis and evaluation of five short-term load forecasting[J]. Techniques IEEE Transactions on Power Systems, 1989, 4(4): 1484-1491.
[3] 李媛媛, 牛东晓. 基于最优可信度的月度负荷综合最优灰色神经网络预测模型[J]. 电网技术, 2005, 29(5): 16-19.
LI Yuan-yuan, NIU Dong-xiao. An optimum credibility based integrated optimum gray network model of monthly power load forecasting[J]. Power System Technology, 2005, 29(5): 16-19.
[4] Conejo A J, Plazas M A, Espinola R, et al. Day-ahead electricity price forecasting using the wavelet transform and ARIMA models[J]. IEEE Trans Power Syst, 2005, 20(2): 1035-1042.
[5] 姚李孝, 姚金雄, 李宝庆, 等. 基于竞争分类的神经网络短期电力负荷预测[J]. 电网技术, 2004, 28(10): 45-48.
YAO Li-xiao, YAO Jin-xiong, LI Bao-qing, et al. Short-time load forecasting using neural network based on competitive learning classification[J]. Power System Technology, 2004, 28(10): 45-48.
[6] 鲍正江, 胡海兵. 一种基于神经网络的电力负荷预测方法[J]. 浙江电力, 2004, 23(4): 10-13.
BAO Zheng-jiang, HU Hai-bing. An electric load forecast method based on the neural network[J]. Zhejiang Electric Power, 2004, 23(4): 10-13.
[7] Torre S D L, Conejo A J, Contreras J. Simulating oligopolistic pool-based electricity markets: A multiperiod approach[J]. IEEE Trans Power Syst, 2003, 18(4): 1547-1555.
[8] Tien T L. A research on the deterministic grey dynamic model with multiple inputs DGDMMI(1,1,1)[J]. Applied Mathematics and Computation, 2003, 139(1): 401-416.
[9] Robinson T A. Electricity pool prices: A case study in nonlinear time-series modeling[J]. Applied Economics, 2000, 32(5): 527-532.
[10] 阎平凡, 张长水. 人工神经网络与模拟进化计算[M]. 北京: 清华大学出版社, 2006.
YAN Ping-fan, ZHANG Chang-shui. Artificial neural networks and evolutionary computing[M]. Beijing: Tsinghua University Press, 2006.
[11] 姚李孝, 宋玲芳, 李庆宇, 等. 基于模糊聚类分析与BP网络的电力系统短期负荷预测[J]. 电网技术, 2005, 29(1): 20-23.
YAO Li-xiao, SONG Ling-fang, LI Qing-yu, et al. Power system short-term load forecasting based on fuzzy clustering analysis and BP neural network[J]. Power System Technology, 2005, 29(1): 20-23.
[12] El-Gallad A, El-Hawary M, Sallam A, et al. Swarm-intelligently trained neural network for power transformer protection[C]// Proceedings of Conference on Electrical and Computer Engineering. Canadian, 2001: 265-269.
[13] 刘 玲, 严登俊, 龚灯才, 等. 基于粒子群模糊神经网络的短期电力负荷预测[J]. 电力系统及其自动化学报, 2006, 18(3): 47-50.
LIU Ling, YAN Deng-jun, GONG Deng-cai, et al. New method for short term load forecasting based on particle swarm optimization and fuzzy neural network[J]. Proceedings of the Chinese Society of Universities, 2006, 18(3): 47-50.
收稿日期:2008-10-15;修回日期:2008-12-21
基金项目:国家自然科学基金资助项目(70671040)
通信作者:杨尚东(1981-),男,湖南宁远人,博士,从事经济技术评价与决策优化、电力企业管理理论等研究;电话:010-80798623;E-mail: sd-yang@sgcc.com.cn