文章编号: 1004-0609(2005)01-0105-05
基于分子动力学结合神经网络的Au表面能计算方法
汤 伟, 朱定一, 陈丽娟, 关翔锋
(福州大学 材料科学与工程学院, 福州350002)
摘 要: 利用嵌入原子模型, 采用分子动力学方法计算了贵金属Au低指数晶面及部分简单高指数晶面的表面能。 同时, 采用Levenberg-Marquardt 算法, 建立了Au表面能的BP神经网络模型; 结合分子动力学模型的计算数据, 通过大量数据的自学习训练, 完成神经网络模型对Au高指数晶面表面能的预测。 计算结果表明: 该方法具有较高的预测精度, 能正确预言低指数晶面表面能的排序, Au各晶面的表面能随其晶面与(111)密排面夹角的增大呈现先增大后减小的特点。
关键词: 表面能; 嵌入原子势; 人工神经网络; Levenberg-Marquardt算法 中图分类号: TG146.31; TP183
文献标识码: A
Calculation of Au surface energy by molecular dynamics combined with neural networks
TANG Wei, ZHU Ding-yi, CHEN Li-juan, GUAN Xiang-feng
(School of Materials Science and Engineering, Fuzhou University, Fuzhou 350002, China)
Abstract: Via embedded-atom model and molecular dynamics simulation, the surface energies of three low-index and some high-index planes were calculated for precious metal Au, and the error back-propagation network (BP) developed by Levenberg-Marquardt algorithm was adopted. Combining the data calculated with the molecular dynamics model, a great deal of data were trained many times and compared with the calculated data, and the prediction of high-index surface energy was performed. The results show that the method has high predicting accuracy. The order of the three low-index planes was predicted exactly. The surface energies on the other planes show a tendency that first increasing and then decreasing with angle between the planes and (111) plane increasing.
Key words: surface energy; embedded-atom potential; artificial neural network; Levenberg-Marquardt algorithm
表面能是表征材料表面特性的重要参量, 在表面、 强度与断裂、 相变等很多材料问题的研究中有广泛应用。 由于晶体在结构上的各向异性, 不同的晶体表面具有不同的表面能, 其物理属性也有很大差异。 Au在电子工业和尖端科学技术领域是极其重要的功能材料, 在电子工业中90%用于表面镀层。 镀金可提高材料的耐腐蚀性, 镀金的另一个作用是对红外有强烈的反射作用, 广泛用于红外探测仪反导弹装置上, Au已成为现代卫星通讯仪器中的关键材料[1]。 近几年, Au的表面催化作用得到广泛的应用[2]。 因此研究Au的表面能具有重要意义。 目前很难通过实验精确测定固态材料的表面能, 即使通过实验能获得一些简单结构晶体的表面能, 但误差一般都比较大。 现在已能够由第一原理方法在超级计算机上直接计算一些简单结构晶体的表面能, 但需要花费大量机时, 同时也存在一定的误差[3]。 若想通过该方法计算实际条件下各种复杂结构晶体的表面能, 目前计算机的运算速度和存储能力仍远远不够。 在这种情况下, 利用近年来迅速发展的各种多体理论, 并结合分子动力学方法进行计算机模拟计算材料的表面能, 可能是目前确定固态材料表面能的有效方法, 它既有一定的准确性, 又对计算机要求不太高, 本文作者采用嵌入原子模型对贵金属Au的低指数及部分高指数晶面的表面能进行分子动力学模拟计算, 但由于复杂高指数晶面表面能用分子动力学方法计算困难, 目前鲜有报道, 本文作者探索性地采用人工神经网络方法, 结合分子动力学模拟的计算数据, 通过大量数据的自学习训练, 完成神经网络模型对高指数晶面表面能的预测, 并与实验结果进行比较。
1 嵌入原子模型及参数的确定
EAM理论[4]是在密度泛函理论基础上,运用有效介质或准原子近似推导出来的,它包括两个主要的假设:一是原子电子密度分布的球面平均假设;二是基体电子密度是原子的电子密度的线性迭加。 根据EAM理论,一个原子集团的总能量可表示为
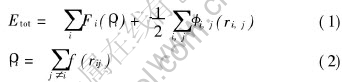
式中 Fi(ρi)为嵌入能; i, j(ri, j)为对势项, 根据需要可以取不同的形式; ρi为除第i个原子以外的所有其他原子的核外电子在第i个原子处产生的电子云密度之和; f(rij)为原子电子密度分布函数; rij为两原子间的间距。
要得到一个嵌入原子模型, 须确定上述函数的形式, 根据Johnson[5]提出模拟计算方法:
F(ρ)=-Ec(1-lnx)x-6ey(3)
f(r)=feexp[-β(r/re-1)](4)
(r)=eexp[-γ(r/re-1)](5)
式中 x=(ρ/ρe)α/β; y=(ρ/ρe)γ/β; α=3(ΩB/Ec)1/2; fe=Ec/Ω; e=Ec/6; 下标e表示平衡状态时的值。
通过拟合晶格常数a、 原子体积Ω、 内聚能Ec、 单空位形成能EUF、 体积模量B和剪切模量G等物性参数和通过拟合获得的模型参数列于表1。
2 人工神经网络原理及模型
2.1 人工神经网络原理
表1 贵金属Au的物理参数和模型参数[5]
Table 1 Physical parameters and model parameters of precious metal Au
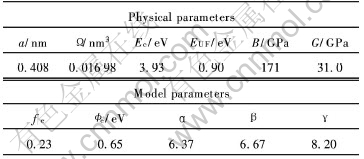
人工神经网络(ANN)是目前国际上迅速发展的前沿交叉学科, 其本质是建立一种映射关系, 在工程应用中属于典型的数据挖掘技术。 人工神经网络以样本数据为基础, 按照某种算法经过有限次迭代获得一个反映样本数据内在规律的数学模型, 因此具有适合于研究复杂非线性系统和不确定过程的优点, 具有广泛的应用前景[6-8]。 由于误差反向传播神经网络(BP网络)具有较强的联想记忆和泛化能力, 本文选用BP网络。 BP网络通过输入数据正向传播和输出误差反向传播两个过程, 修正各层联接权值和阈值,直至输出达到或逼近所要求的响应为止。 具有一个输入层、 一个隐含层、 一个输出层的3层BP神经网络如图1所示(图中, P代表输入层矩阵; w1、 w2分别代表隐含层和输出层的权值矩阵; b1、 b2分别代表隐含层和输出层的偏差向量(阈值); a1、 a2分别代表隐含层和输出层的输出矩阵; n1、 n2分别代表隐含层和输出层神经元的输出矩阵)。 经典BP算法是采用最速下降静态寻优的一阶算法,其在目标函数的极值点附近收敛速度慢, 而经典二阶算法(如高斯-牛顿法)在远离目标函数的极值点时收敛速度很慢[8]。 本研究采用一种拟二阶算法——Levenberg-Marquardt算法[9], 其基本迭代公式为
Δw=(JTJ+μJ)-1JTe(6)
式中 J为误差函数对权值向量微分的Jacobian矩阵; e为误差向量, μ是某个可以进行自适应调整的非负数。 该修正方法依赖于μ的幅值的改变, 光滑地在两种极端之间变化: 即二阶的高斯-牛顿法(μ →0)和经典的梯度法(μ→∞), 充分发挥了两种算法的优势[10]。
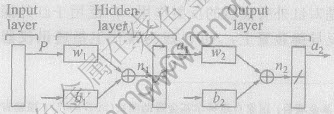
图1 三层BP神经网络
Fig.1 Three layers BP neural network
2.2 表面能预测神经网络模型
2.2.1 BP神经网络模型的建立
将各晶面与3个低指数晶面((100)、 (110)和(111))的夹角作为神经网络训练的输入矢量, 根据立方晶系两个晶面(h1k1l1)和(h2k2l2)间的夹角公式:

可求得各晶面与(100)、 (110)和(111)3个低指数晶面的夹角, 将所对应的根据分子动力学模拟计算的表面能值作为输出矢量, 其结果均列入表2中。
由于BP神经网络选用Sigmoid函数作为网络神经元的传递函数。 根据S函数的特征, 为了保证网络神经元的非线性作用, 对原始数据要进行标准化处理, 标准化处理有助于提高网络的学习速度。 为了将样本输入和输出标准化, 使它们的均值为0, 而标准差为1, 作者对样本进行如下标准化处理:

式中 xp为样本数据, 平均值
, 标准
。
Hecht-Nielson[11]证明了对于任何在闭区间内的一个连续函数都可以用一个单隐层的BP网络来逼近, 一个三层的BP网络可以完成任意的n维到m维的映射。 网络结构选用具有一个隐含层的BP网络, 隐含层采用S型激活函数, 输出层采用线性激活函数。
2.2.2 BP网络神经元数目的选择
隐含层神经元的数目是决定神经网络有效性和准确性的关键因素之一, 一般根据问题的复杂程度和目标的精度选择最适合的神经元数目。 因此, 首先要设定隐含层节点数和误差限, 在训练神经网络的过程中不断调节隐含层节点数目、 训练次数和训练误差限, 直至找到最佳预测结果。 通过多次训练和调节, 综合考虑网络的学习速度和泛化能力,最终确定6个节点的隐含层, 网络的拓扑结构为3-6-1。
3 表面能的计算与讨论
将上述网络模型在MatLab6.1中编制成可运行程序, 并将标准化后的夹角数据作为输入矢量, 表面能作为输出矢量分别代入网络模型进行训练。 用训练所得到的权值, 对表面能进行了预测, 同时将BP神经网络预测相对误差值列入表2中。 由表2可知, 所训练的网络对表面能预测的误差范围是比较稳定和合理的。 图2所示为表面能的神经网络预测结果与MD计算结果的比较, 并给出预测表面能和计算表面能的线性相关曲线。 从图可以看出, 预测值和MD计算值有较高的线性相关性, 线性相关系数R=0.99965。 由此可知, 该预测模型具有高的预测精度。 平均相对误差ε[TX-]可以用以下公式计算:

式中 di为目标输出值; oi为预测输出值, n为输出数据数目。 经计算可得:
, 表明表面能的计算值和预测值非常接近, 这说明上面建立的模型具有较高的精度。 需要指出的是, 由于本文作者对数据进行了标准化处理, 程序所控制的目标误差是对归一化后的数据而言, 而上面计算的相对误差是对原始数据而言的。 对于一个神经网络, 关键在于网络结构、 权值和阈值。 一旦网络训练完成, 这些数据就可以得到, 将它们保存起来, 编写成预测函数, 再次进行训练, 就无需再对神经网络进行训练, 预测的速度大大加快。
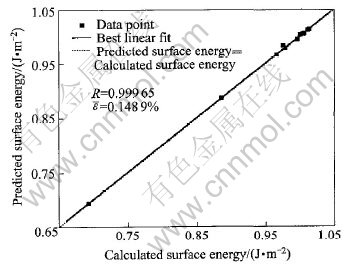
图2 表面能的神经网络预测结果与MD计算结果的比较
Fig.2 Comparison of ANN-predicted and calculated surface energy
利用上述模型, 作者对部分未知表面的晶面能进行了预测, 其结果列于表2中。
分析训练结束后的权值矩阵可以发现, 与输入矢量θ3相关的权值明显高于其他两个输入矢量, 表明各晶面的表面能大小主要与密排面(111)的夹角有关。 表面能γ随各晶面与(111)面夹角θ的变化如图3所示。 可以看出, 面心立方的(111)密排面的表面能最小, 和预期结果一致。 其他各晶面表面能随其与(111)面夹角的增大, 在角度较小时, 呈现明显的增大趋势, 并在θ≈40°附近时达到最大值, θ继续增大, 表面能快速下降。 对本文计算获得的各晶面表面能值研究发现, 表面能值随θ增大快速下降的区域, 是同属于〈001〉晶带轴的高密勒指数晶面(如(410)和(510)等)。 本文计算获得的Au各晶面表面能随其与(111)晶面夹角θ的变化的关系与张建民等[12]采用改进嵌入原子法计算Cu晶体的表面能得到的表面能面密度随各晶面与(111)晶面夹角θ的变化趋势基本一致。 可以预知具有面心立方特征的金属各晶面表面能随其与(111)晶面夹角θ的变化趋势基本上呈现先增大后减小的特点。 对比图3和表2还可以发现, 当不同晶面和密排面夹角相同时, 就必须考虑与其他二个低指数晶面的夹角关系。
表2 Au不同晶面与3个低指数晶面的夹角及各晶面的表面能
Table 2 Angle between planes and three low-index planes and surface energies
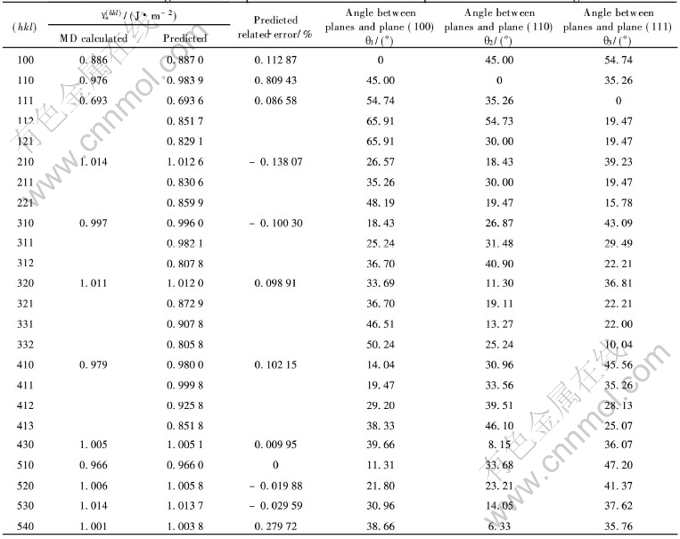
经计算可得, 预测的所有晶面表面能的平均值为0.9202J/m2, 同实验测得的Au多晶体的表面能
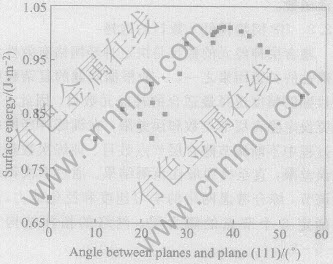
图3 表面能随各晶面与(111)晶面夹角的变化
Fig.3 Variation of surface energy with angle between planes and plane (111)
面密度平均值1.50J/m2[13] 、 Skriver的第一性原理计算值[3]和Foiles的EAM计算值[14]比较发现, EAM理论模型计算表面能及本方法预测的表面能均存在结果偏小的问题。 这主要是: 1)实验测定表面能1.50J/m2是所有晶面的表面能的平均值, 而本文计算的晶面较少; 2)对通过分子动力学方法计算的几个晶面没有考虑到表面原子的松驰。 因此仅考虑3个输入变量将会使预测结果存在一定的误差。 而Skriver第一性原理计算获得的最密排面(111)晶面的表面能值为1.61J/m2, 比实验获得的平均表面能还要高, 同样显示了Skriver方法的不足。 目前尚没有一种模拟计算方法可以完全解决计算晶面表面能同实验数据存在误差的问题。 由表2中表面能预测数据及图2可知所建立的联合模型可以正确预言低指数晶面表面能ES的排序: ES(111)〈ES(100)〈ES(110), 即表面的开放度越大, 其表面能越大。 (111)是最密排面, 该面的表面能最低。 这表明, 表面原子排列越紧密, 表面能越低。 根据固体表面物理学的有关理论[15], 表面原子排列越紧密时, 原子间的相互作用越强, 从而使材料表面能降低, 这与计算结果是一致的。
4 结论
1) 利用神经网络对晶面夹角和表面能样本集的学习, 采用改进的BP网络算法——Levenberg-Marquardt算法建立了表面能人工神经网络模型; 计算表明该神经网络模型具有较高的精度和很好的泛化能力。 该方法可以直接用于其他面心立方金属表面能的计算, 也可以类推到其他的晶体结构。
2) Au晶体其他晶面的表面能随其与(111)面夹角的增大呈现出先增大后减小的趋势, 并在θ≈40°附近时达到最大值。 表面能值随θ增大快速下降的区域, 是同属于〈001>晶带轴的高密勒指数晶面(如(410)和(510)等)。
3) 分子动力学计算的表面能数据的整体精度及所考虑影响因素的全面性, 决定了对Au各晶面表面能的预测精度。
REFERENCES
[1]阮德水, 李卫萍. 金的化学[J]. 高等函授学报(自然科学版), 2000, 13(1): 25-29.
RUAN De-shui, LI Wei-ping. Gold chemistry[J]. Journal of Higher Correspondence Education(Natural Science), 2000, 13(1): 25-29.
[2]董守安. 纳米技术中的金元素[J]. 贵金属, 2003, 24 (1): 54-61.
DONG Shou-an.The role of gold in the nanotechnology[J]. Precious Metals, 2003, 24 (1): 54-61.
[3]Skriver H L, Rosengaard N M. Surface energy and work function of elemental metals[J]. Phys Rev B, 1992, 46(11):7157-7168.
[4]Daw M S, Baskes M I. Embedded-atom method: derivation and application to impurities, surface, and other defects in metals[J]. Phys Rev B, 1984, 29(12): 6443-6453.
[5]Johnson R A. Alloy models with the embedded-atom method[J]. Phys Rev B, 1989, 39(17): 12554-12559.
[6]QI Le-hua. Research on prediction of the processing parameters of liquid extrusion by BP network[J].Journal of Materials Processing Technology, 1999, 95: 232-237.
[7]Basheer L A. Artificial neural network: fundamentals, computing, design, and application[J]. Journal of Microbiological Methods, 2000, 43: 3-31.
[8]Joines J A, White M W. Improved generalization using robust cost functions[A]. IEEE/INNS Int Joint Conference of Neural Networks[C]. New York: IEEE Press, 1992. 911-918.
[9]Hagan M T, Menhaj M. Training feedforward networks with Marquardt algorithm[J]. IEEE Transaction on Neural Networks, 1994, 5(6): 989-993.
[10]张智星, 孙春在, 水谷英二, 等. 神经-模糊和软计算[M]. 西安: 西安交通大学出版社, 1998. 156-215.
ZHANG Zhi-xing, SUN Chun-zai, Mizutani E, et al. Neuro-Fuzzy and Soft Computing[M]. Xian: Xian Jiaotong University Press,1998. 156-215.
[11]Hech-Nielsen R. Neurocomputing[M]. Massachusetts: Addison Wesley Publishing Company, 1991.
[12]张建民, 徐可为, 马飞. 用改进嵌入原子法计算Cu晶体的表面能[J]. 物理学报, 2003, 52(8): 1993-1999.
ZHANG Jian-min, XU Ke-wei, MA Fei. Calculation of suface energy of Cu crystal with modified embedded atom method[J]. Acta Physica Sinica, 2003, 52(6): 1993-1999.
[13]De Boer F R, Boom R, Mattens W C M, et al. Cohesion in metals[M]. Amsterdam, North-Holland: Elsevier Science, 1988. 716.
[14]Foiles S M, Baskes M I, Daw M S. Embedded atom method functions for the FCC metals Cu, Ag, Au, Ni, Pd, Pt, and their alloys[J]. Phys Rev B, 1986, 33(12):7983-7990.
[15]闻立时. 固体材料界面研究的物理基础[M]. 北京: 科学出版社, 1991. 21.
WEN Li-shi. Physics Basis of Solid Materials Interface Research[M]. Beijing: Science Press, 1991. 21.
基金项目: 国家自然科学基金资助项目(50471007); 福建省自然科学基金资助项目(A0210008)
收稿日期: 2004-05-25; 修订日期: 2004-11-07
作者简介: 汤 伟(1981-), 男, 硕士研究生.
通讯作者: 朱定一, 副教授; 电话: 0591-87893540; E-mail: zdy7081@163.com
(编辑陈爱华)