基于不确定性采样的自训练代价敏感支持向量机研究
江彤1,唐明珠2,阳春华2
(1. 湖南人文科技学院 计算机科学技术系,湖南 娄底,417000;
2. 中南大学 信息科学与工程学院,湖南 长沙,410083)
摘要:针对样本集中的类不平衡性和样本标注代价昂贵问题,提出基于不确定性采样的自训练代价敏感支持向量机。不确定性采样通过支持向量数据描述评价未标注样本的不确定性,对不确定性高的未标注样本进行标注,同时利用自训练方法训练代价敏感支持向量,代价敏感支持向量机利用代价参数和核参数对未标注样本进行预测。实验结果表明:该算法能有效地降低平均期望误分类代价,减少样本集中样本需要标注次数。
关键词:主动学习;代价敏感支持向量机;自训练方法;不确定性采样;支持向量数据描述
中图分类号:T18 文献标志码:A 文章编号:1672-7207(2012)02-0561-06
Self-training cost-sensitive support vector machine with uncertainty based on sampling
Jiang Tong1, TANG Ming-zhu2, YANG Chun-hua2
(1. Department of Computer Science and Technology, Hunan Institute of Humanities,Science and Technology, Loudi 417000, China;
2. School of Information Science & Engineering, Central South University, Changsha 410083, China)
Abstract: Self-training cost-sensitive support vector machine with uncertainty based sampling (SCU) was proposed to solve two difficulties of class-imbalanced dataset and expensive labeled cost. The uncertainty of unlabeled sample was evaluated using support vector data description in uncertainty based sampling. The unlabeled sample with high uncertainty was selected to be labeled. Cost-sensitive support vector machine was trained using self-training approach. Cost parameters and kernel parameters of cost-sensitive support vector machine were employed to predict a class label for an unlabeled sample. The results show that SCU effectively reduces both average expected misclassification costs and labeled times.
Key words: active learning; cost-sensitive support vector machine; self-training approach; uncertainty based sampling; support vector data description
复杂工业过程一旦发生故障,有可能直接影响产品数量和质量,甚至会发生系统瘫痪或灾难性事故,因此,对生产过程的故障诊断提出更高要求。 由于实际工业生产过程不确定因素多且机理复杂,使得建立精确的故障诊断模型极其困难。 随着工业生产过程信息化普及,生产过程中积累了丰富的数据,数据中蕴藏着反映生产规律的信息。数据驱动的故障诊断方法因可避免解析法要求详细、准确的定量数学模型的缺点,已成为研究热点,并广泛应用于工业过程的故障诊断,如半导体蚀刻过程[1]、Tennessee eastman(TE)过程[2]、连续搅拌式反应器工艺过程[3]、密闭鼓风炉过程[4]等。但是,故障诊断样本集中存在2种问题需要解决:(1) 类不平衡问题,即故障类样本与正常类样本之间的类不平衡性问题,故障诊断样本集中正常类样本占绝大多数,故障类样本占极少数;(2) 标注代价昂贵问题,故障诊断样本集中未标注样本占绝大多数,已标注样本占极少数,而标注未标注样本集中的每一个样本要付出一定的金钱和时间代价。针对类不平衡问题,代价敏感元学习算法Metacost[5]通过修改训练集中样本类标签,改变训练集中样本分布比例来减少误分类代价;代价敏感概率神经网络[6]直接嵌入误分类代价来提高故障敏感性和减少误分类代价;代价敏感支持向量机[7](Cost-sensitive support vector machine,CS-SVM)引入2个误分类代价参数来提高故障敏感性。针对标注代价昂贵问题,基于委员会的投票采样[8]选择委员之间判别争议最大的样本作为下一个待标注的样本;期望损失减小采样算法[9]选择当前能够最小化未来测试集上期望损失的样本进行标注;不确定性采样[10]选择最不确定的样本作为下一步待标注的样本。针对类不平衡问题和标注代价昂贵问题,Margineantu[11]将代价敏感学习和主动学习结合起来,提出主动代价敏感学习框架。龙军等[12]以入侵检测试验对象,结合代价敏感学习与主动学习,提出了代价敏感主动学习算法。该算法能够有效地减少标注次数。主动学习在某些样本集上泛化能力不理想,而自训练方法能提高主动学习泛化能力,并已成功地应用于对象检测[13]。Liu等[14]提出自训练代价敏感不确定性采样方法,利用自训练方法能有效提高主动学习泛化能力。针对工业过程故障诊断样本集中的类不平衡问题和标注代价问题,提出基于不确定性采样的自训练代价敏感支持向量机(Self-training cost-sensitive support vector machine with uncertainty based sampling,SCU)。 该方法以代价敏感支持向量机作为基准分类器,通过支持向量数据描述评价未标注样本的不确定性,用自训练方法训练代价敏感支持向量机。 以典型的复杂化学工业过程TE过程数据集为例,验证了SCU的有 效性。
1 代价敏感支持向量机
由Vapnik[15]提出的标准支持向量机(Support vector machine,SVM),以结构风险最小化构造决策超平面,控制模型复杂度和经验风险之间的平衡。标准支持向量机在类平衡样本集上表现出良好分类性能,但在类不平衡样本集上分类性能表现不佳。标准的支持向量机只有1个正则化参数,认为故障类样本误分成正常类样本的代价与正常类样本误分成故障类样本的代价是相同的,而实际过程中故障类样本误分成正常类样本的代价远高于正常类样本误分成故障类样本的代价,因此,Davenport[7]提出了代价敏感支持向量机,类别不同的样本被误分时被赋予不同的误分类代价。假设已知类不平衡样本集为:
,
;
;
;I-=
,能被超平面分类。代价敏感支持向量机优化问题的原问题为:
(1)
其中:C1和C2为误分类代价参数;k(w,xi)为核函数;
为误差。代价敏感支持向量机原问题(1)的对偶问题为:
(2)
其中:αi为Lagrange乘子;k(xi,xj)为核函数。Davenport[7]认为:在没有先验知识的情况下,选择高斯核函数时CS-SVM分类性能优于其他核函数的分类性能。
2 主动学习
往往故障诊断样本集中已标注样本很难获取,而且标注样本的代价非常昂贵、枯燥乏味或异常困难。传统的监督学习算法被认为是被动学习算法。被动学习只是随机地选择训练样本,被动地接受这些样本的信息进行学习。而主动学习在学习过程中可以根据学习进程,选择最有利于分类器性能的样本来进一步训练分类器,它能有效地减少评价样本的数量,因此,主动学习能有效减少故障诊断样本集中样本标注 代价。
主动学习由学习算法和采样算法2部分构成。目前,主动学习研究的重点在于采样算法,根据不同的采样标准,采样算法可分为3类:基于委员会的投票采样算法、期望损失减小采样算法和不确定性采样算法。Freund等[8]提出基于委员会的投票采样算法,该算法训练多个分类器(委员),并选择分类器之间判别争议最大的样本作为下一个查询的样本。该算法能减少训练样本的标注量,但要构建一个能代表不同版本空间区域的模型委员会比较困难。Roy等[9]将期望损失减小采样用于文本分类,但基于期望损失减小的主动学习计算代价昂贵。Lewis等[10]提出不确定性采样算法,采用一个分类器对样本进行评价,并同时给出对所有未标注样本评价的不确定性。分类器评价出最不确定的样本作为下一步待标注的样本。该文献指出不确定性采样能大幅度减小训练规模,从而减少标注代价。
在主动学习中,选择最有利于分类器性能的样本作为待标注的样本,可以认为最有利于分类器性能的样本是距分类边界最近的样本。在分类器边界上或在附近的样本是最难被分类的样本,分类器对其评价最不确定。这些样本能有效地提高分类器的可信度,从而提高分类器的泛化性能。
Vapnik[15]提出的支持向量机无法很好地解决类不平衡问题,而Tax等[16]提出的支持向量数据描述 (Support vector data description,SVDD)能有效地估计故障类、正常类样本在特征空间中的分布,因此,通过支持向量数据描述计算未标注样本的不确定性度进行采样。
样本集X如
,
{+1,-1}。在支持向量数据描述不确定性采样过程中,样本到支持向量数据描述超球面的距离为:
(3)
其中:x为测试特征向量;xi和xj为支持向量;αi和αj为对应支持向量xi和xj的拉格朗日乘子;K(x·x)为核函数;n为样本数。样本距分类边界的距离表征了样本的不确定性度,样本离分类边界越近,则不确定性度越高;反之,样本离分边界越远,则不确定性度越低。因此,可用下式来计算不确定性度:
(4)
其中:阈值θ根据经验设定。本文选择不确定性度高的样本作为待标注样本,选择高斯核函数作为支持向量数据描述的核函数。
3 主动自训练代价敏感支持向量机
3.1 自训练方法
自训练方法是一种半监督学习方法,是基于Angluin和Laird提出的有关训练带噪声时目标假设的PAC可学习性构造[17]。按照Angluin和Laird的结 论[17]:当抽取m个训练样本序列σ时,若样本规模
,则与序列σ一致的最小
的假设Hi具有PAC可学习性,即Pr[d(Hi,H*)≥ξ]≤δ。其中:ξ为最坏情况下假设的分类错误率;η为训练集噪声率的上限;N为假设的数目;δ为置信度;d(Hi,H*)为假设Hi和真实假设H*相应样本集的对称差集中元素概率之和,即
。
令
且μ=m/m′,则m=
。令常量
,则m=
。因此,代价敏感支持向量机训练所得假设的分类错误率与训练集规模、训练集噪声率间有如下关系成立:
(5)
SCU通过不确定性采样保证每个分类器新训练集规模m迭代增大的同时,自训练确保每次重新训练所得假设的分类器错误率ξ降低。
3.2 基于不确定性采样的自训练代价敏感支持向 量机
主动自训练代价敏感支持向量机学习描述如下:训练样本分为极少数已标注样本和绝大多数未标注样本;通过支持向量数据描述计算未标注样本的不确定性度,选择不确定性度大的前|u|个样本作为待标注的样本,提交专家标注样本,加入训练集;利用已标注样本训练集出3个CS-SVM;3个CS-SVM对剩余未标注样本集进行预测,若第2个和第3个CS-SVM对该样本集中未标注样本预测一致,则将未标注样本添加到预测的未标注的样本集中,合并已预测的未标注的样本集和已标注样本集,训练CS-SVM,整个过程迭代多次,最终训练出CS-SVM。
基于不确定性采样的自训练代价敏感支持向量机 (SCU)如下。
输入:标注样本集L,未标注样本集U,代价矩阵C,最大迭代次数tmax,不确定性度高的样本数|u|,平均期望代价的阈值δ,未标注样本的预测标注集
;
输出:代价敏感支持向量机
。
Step 1 初始化标注样本集L,未标注样本集U,代价矩阵C,最大迭代次数tmax,不确定性度高的样本数|u|,平均期望代价的阈值δ,未标注样本的预测标注集
。
Step 2 将标注样本集L复制给样本集dataset,dataset为L。
Step 3 用样本集dataset训练测试分类器
(通过10折交叉验证计算AvgMisCost)。
Step 4 若满足终止条件(迭代最大次数tmax,或迭代次数t和一次选择不确定性高的样本数|u|乘积小于总的未标注样本数|U|,或平均期望代价AvgMisCost小于阈值δ),则输出分类器
参数及分类性能,算法结束;否则,转到Step 5。
Step 5 用标注样本集L训练支持向量数据描述SVDD,通过支持向量数据描述计算未标注样本集中的未标注样本不确定性度,选出前|u|个不确定性度大的未标注样本集。
Step 6 对u每一个样本xl{提交专家标注样本xl,yl=label(xl);更新已标注样本集
;}
Step 7 更新未标注样本集U=U\u。
Step 8 对U每一个样本xl{用hCS-SVM1,hCS-SVM2,hCS-SVM3预测样本xl的类标,
,
,
。
若
,则更新未标注样本的预测标注集
;}
Step 9 将标注样本集L和未标注样本的预测标注集
合并成样本集
。
Step 10 迭代次数增1即t =t+1,转到Step 3。
4 实验与分析
Tennessee eastman (TE)过程[18]是一个典型复杂工业化学过程。TE过程有5个主要操作即单元压缩机、分离器、反应器、冷凝器和汽提塔,有8种成分即A~H。TE过程有12个控制变量和41个测量变量。实验选取41个测量变量和11个控制变量、1个类别变量,其中52个变量作为SCU的输入变量,1个类别变量作为SCU的输出变量。正常类样本采自过程平稳运行状态,采样间隔为3 min,仿真运行时间为50 h,采样 1 000个样本。故障类样本的采样间隔为3 min,每次仿真运行时间为10 h,每类故障采样200个样本,总共有21类故障。以最常见阶跃类型故障和随机变量类型故障为例,验证SCU算法的有效性。数据集1由故障1(阶跃类型)200个样本和正常类1 000个样本组成;数据集2由故障8(随机变量类型)200个样本和正常类1 000个样本组成。
为了全面评价主动代价敏感支持向量机学习算法的分类性能,将全局的平均误分类代价作为评价标准。
全局的平均误分类代价定义为:
(6)
其中:tp和fn表示使用分类器将原本属于故障类样本分别预测为故障类样本的个数和正常类样本的个数;fp和tn表示使用分类器将原本属于正常类样本分别预测为故障类样本的数和正常类样本的数;Ci|j为将第j类的样本误分到第i类的代价;
。
为了验证面向复杂工业过程故障诊断的主动代价敏感支持向量机学习算法的有效性,本文分2个部分进行实验:(1) 验证SCU的支持向量数据描述不确定性采样效果,比较支持向量数据描述不确定性采样与随机采样SCU;(2) 验证SCU的自训练效果,比较SCU与主动非自训练代价敏感支持向量机学习。 实验中采用的代价矩阵为:
(7)
其中:C1|2和C2|1对应于代价敏感支持向量机的2个代价参数C1和C2。本文在机器学习平台Weka进行了2次开发,并实现了SCU算法。下面所有实验结果为10折交叉验证结果。
4.1 不确定性采样与随机采样的比较
将SCU基于支持向量数据描述的不确定性采样和随机采样进行比较,以验证基于支持向量数据描述不确定性采样的效果。除了采样方法不同以外,SCU算法的其他参数一致。
图1和图2所示分别表示SCU不确定性采样和随机采样在数据集1和2上的平均误分类代价比较结果。由图1和图2可知:随着SCU的迭代次数增加,SCU不确定性采样平均误分类代价低于SCU随机采样的平均误分类代价;SCU随机采样分类性能波动比较大,随机采样的样本不具有代表性,可能没有采到信息量最大的样本。这说明SCU不确定性采样由于通过支持向量数据描述评价未标注样本的不确定性度,选择不确定性度高的前|u|个样本作为待标注样本,这可以最大化分类器的分类性能。而随机采样没有评价未标注样本的不确定性度,不能选择最有利分类器的未标注样本。同时,在标注次数相同的情况下,SCU不确定性采样能达到更低的平均误分类代价,说明不确定性采样能减小标注次数,从而降低标注代价。
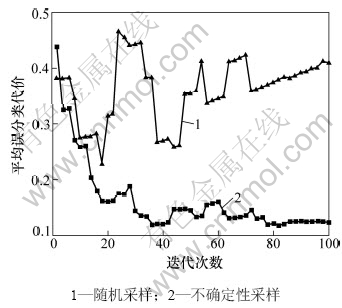
图1 不确定性采样与随机采样在数据集1上的平均误分类代价
Fig.1 Average misclassification cost for uncertainty sampling and random sampling on data 1
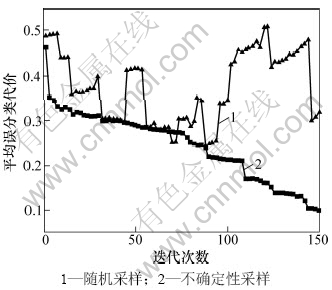
图2 不确定性采样与随机采样在数据集2上的平均误分类代价
Fig.2 Average misclassification cost for uncertainty sampling and random sampling on data 2
4.2 基于不确定性采样的自训练代价敏感支持向量机与基于不确定性采样的非自训练代价敏感支持向量机的比较
将SCU与基于不确定性采样的非自训练代价敏感支持向量机进行比较,以验证自训练的效果。除是否有自训练策略外,其他参数相同。
图3和图4所示分别表示SCU自训练和非自训练在数据集1和2上的平均误分类代价。由图3和图4可知:随着SCU的迭代次数增加,与主动代价敏感支持向量机相比,SCU的平均误分类代价更低,收敛速度更快,分类性能更加稳定;每次SCU迭代表示不同的训练集和测试集,SCU在测试样本集表现良好的分类性能,表明SCU泛化能力更强;而主动代价敏感支持向量机误分类代价波动很大,有时能获得良好的分类性能,有时分类性能较差,平均误分类代价较大。因此,SCU采用自训练方法在整个样本集上训练测试,充分利用正确定标注的未标注样本,使得SCU分类性能更加稳定,泛化能力更强。
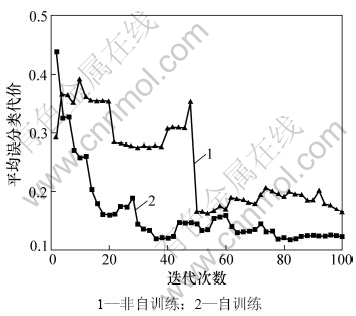
图3 自训练和非自训练在数据集1上平均误分类代价
Fig.3 Average misclassification cost for self-training approach and non-self-training approach on data 1
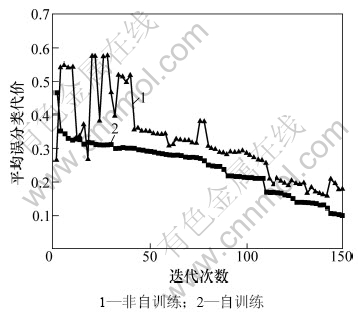
图4 自训练和非自训练在数据集2上平均误分类代价
Fig.4 Average misclassification cost for self-training approach and non-self-training approach on data 2
5 结论
(1) 由于样本标注的代价非常昂贵,为了减少样本的标注代价,主动学习通过支持向量数据描述评价未标注样本的不确定性度,对不确定性高的未标注样本提交专家标注,有效地减少标注的样本量,从而减少样本的标注代价。
(2) 由于故障类样本预测为正常类样本的代价远高于正常类样本预测为故障类样本代价,CS-SVM将未标注样本预测为具有最小平均期望误分类代价的类,从而减少误分类代价。自训练方法将CS-SVM正确标注的未标注样本加入样本集,使得SCU充分利用未标注样本,减少误标注样本的负面影响,有效地提高SCU泛化能力。
(3) 该算法能有效地降低平均期望代价,减少标注样本次数。
参考文献:
[1] Mahadevan S, Shah S L. Fault detection and diagnosis in process data using one-class support vector machines[J]. Journal of Process Control, 2009, 19: 1627-1639.
[2] YU Jian-bo. Hidden Markov models combining local and global information for nonlinear and multimodal process monitoring[J]. Journal of Process Control, 2010, 20(3): 344-359.
[3] Choi S W, Morris J L, Lee I B. Nonlinear multiscale modelling for fault detection and identification[J]. Chemical Engineering Science, 2008, 63(8): 2252-2266.
[4] 蒋少华, 桂卫华, 阳春华, 等. 基于RS与LS-SVM多分类法的故障诊断方法及应用[J]. 中南大学学报: 自然科学版, 2009, 40(2): 447-451.
JIANG Shao-hua, GUI Wei-hua, YANG Chun-hua,et al. Fault diagnosis method based on rough set and least squares support vector machine and its application[J]. Journal of Central South University: Science and Technology, 2009, 40(2): 447-451.
[5] Domingos P. MetaCost: A general method for making classifiers cost-sensitive[C]//Proceedings of the Fifth International Conference on Knowledge Discovery and Data Mining. San Diego, USA, 1999: 155-164.
[6] 唐明珠, 阳春华, 桂卫华, 等. 代价敏感概率神经网络及其在故障诊断中的应用[J]. 控制与决策, 2010, 25(7): 1074-1078.
TANG Ming-zhu, YANG Chun-hua, GUI Weo-hua, et al. Cost-sensitive probabilistic neural network and its application in fault diagnosis[J]. Control and Decision, 2010, 25(7): 1074-1078.
[7] Davenport M A. The 2nu-SVM: A cost-sensitive extension of the nu-SVM[R]. Texas: Rice University ECE Technical Report: No. TREE 0504, 2005.
[8] Freund Y, Seung H S, Shamir E, et al. Selective sampling using the query by committee algorithm[J]. Machine Learning, 1997, 28: 133-168.
[9] Roy N, McCallum A. Toward optimal active learning through sampling estimation of error reduction [EB/OL]. [2010-07-06]. http://www.connex.lip6.fr/~amini/RelatedWorks/Roy01.pdf.
[10] Lewis D, Gale W. A sequential algorithm for training text classifiers[C]//Proceedings of the ACM SIGIR Conference on Research and Development in Information Retrieval. London: ACM/Springer, 1994: 3-12.
[11] Margineantu D D. Active cost-sensitive Learning[C]//The Nineteenth International Joint Conference on Artificial Intelligence. Edinburgh, Scotland: Elsevier Science, 2005: 1622-1623.
[12] 龙军, 殷建平, 祝恩, 等. 针对入侵检测的代价敏感主动学习算法[J]. 南京大学学报: 自然科学, 2008, 44(5): 527-535.
LONG Jun, YIN Jian-ping , ZHU En, et al. Cost-sensitive active learning algorithm for intrusion detection[J]. Journal of Nanjing University: Natural Sciences, 2008, 44(5): 527-535.
[13] Tomanek K, Hahn U. Semi-supervised active learning for sequence labeling[C]//Proceedings of the 47th Annual Meeting of the ACL and the 4th IJCNLP of the AFNLP. London: Springer-Verlag, 2009: 1039-1047.
[14] Liu A, Jun G, Joydeep G. A self-training approach to cost sensitive uncertainty sampling[J]. Machine Learning, 2009, 76(2): 257-270.
[15] Vapnik V. Statistical learning theory[M]. New York: John Wiley, 1998: 53-76.
[16] Tax D M J, Duin R P W. Support vector data description[J]. Machine Learning, 2004, 54(1): 45-66.
[17] Greiner K, Grove A J, Roth O. Learning cost sensitive active classifiers[J]. Artificial Intelligence, 2002, 139(2): 137-174.
[18] Downs J J, Vogel E F. A plant-wide industrial process control problem[J]. Computers and Chemical Engineering, 1993, 17(3): 245-255.
(编辑 陈灿华)
收稿日期:2011-03-05;修回日期:2011-05-28
基金项目:国家杰出青年科学基金资助项目(61025015);国家自然科学基金资助项目(60874069);国家高技术研究发展计划(“863”计划)项目(2009AA04Z137);湖南省教育厅科学研究项目(11C0699);中南大学优秀博士学位论文扶植项目(2010年)
通信作者:唐明珠(1983-),男,湖南平江人,博士研究生,从事数据挖掘、机器学习、故障诊断等研究;电话:0731-88836876;E-mail:tmzhu@163.com