采空区危险性评价方法优化
冯岩,王新民,程爱宝,张钦礼,赵建文
(中南大学 资源与安全工程学院,湖南 长沙,410083)
摘要:为了更合理精确地评价采空区危险性,对利用神经网络评价的方法进行优化,建立主成分分析法与神经网络结合的采空区危险性评价模型。从地质和工程条件出发,综合考虑影响采空区稳定性的13项主要因素,统计样本数据。运用主成分分析法对影响采空区稳定性的样本进行预处理,将分析结果作为神经网络的输入数据,减少输入变量,消除变量之间的相关性,从而加快数据处理速度,提高预测精度。将该方法应用于锡矿山的采空区危险性评价。结果表明,预测误差在8%以内,较未经主成分分析的神经网络预测精度有了很大提高。利用主成分分析法和神经网络结合建立的采空区危险性评价模型具有分析速度快、预测精度高等优点。该方法科学合理,为采空区危险性评价提供了一种更完善的评价体系。
关键词:危险性评价;采空区;主成分分析法;BP神经网络;方法优化
中图分类号:TD325 文献标志码:A 文章编号:1672-7207(2013)07-2881-08
Method optimization of underground goaf risk evaluation
FENG Yan, WANG Xinmin, CHENG Aibao, ZHANG Qinli, ZHAO Jianwen
(School of Resources and Safety Engineering, Central South University, Changsha 410083, China)
Abstract: The method of neural networks was optimized, and the principal component analysis and neural networks were used to construct a model about underground goaf risk evaluation to evaluate the underground goaf risk more accurately and reasonably. On the basis of geological and engineering conditions, the 13 main factors that affecting the stability of goaf were considered to count the sample data. The principal component analysis was conducted to the samples, taking the results as the input data for neural network. By reducing the number of input variables, and eliminating the correlation between variables, the data processing was sped up, and the prediction accuracy was improved. The method was applied to evaluate the risk underground goafs in Xi mine. The results show that the prediction error is controlled within 8%, and the accuracy is improved greatly compared with that predicted by neural network without principal component analysis. Assessing the risk of goaf with principal component analysis and neural network has the advantage of rapid analysis and prediction accuracy. The method is scientific and reasonable, and a better evaluation system for the underground risk evaluation is provided.
Key words: risk evaluation; underground goaf; principal component analysis; BP-neural networks; method optimization
随着矿产资源加速消耗,易于开采的优质资源日渐枯竭,为了满足工业生产的不断需求以及矿产资源的合理利用,浅部残矿资源的回收显得尤为重要。但是,许多矿山由于早期采用空场法以及民采、盗采等原因,形成了大量采空区,空区的存在使残矿回采环境恶化,矿柱易发生变形破坏,严重时可能导致大面积冒落、地表塌陷和突水等灾害的发生,造成重大的人员伤亡事故和严重的经济损失[1]。因此,有关采空区危险性评价的研究,对保证矿山安全生产和持续发展有着非常重要的意义。近年来,国内外学者就采空区危险性方面提出了各具特色的分析研究方法,主要包括模糊综合评价法[2]、聚类分析法[3]、灰色理论分析法[4]、未确知理论法[5]和神经网络预测法[6]等。其中,神经网络由于具有高度的非线性映射能力,被广泛应用于采空区稳定性分析中。目前,将神经网络应用到采空区危险性评价时,往往忽视了输入变量间存在的相关性,而且由于输入数据过多,建模的效率较低[7]。因此,本文作者采用主成分分析法与BP神经网络结合的方式建立采空区危险性评价模型,用主成分分析法对原始数据进行预处理,以减少神经网络输入因子的数量,消除输入因子之间的相关性,提高了网络学习速度和分析精度[8]。
1 主成分分析法的基本原理与数学模型
1.1 主成分分析法的基本原理
主成分分析法(primary component analysis)主要是通过降维过程,将多个相关联的数值指标转化为少数几个互不相关的综合指标的统计方法[9]。对于一些多指标问题,指标间可能彼此相关,直接分析不但复杂而且可能得不出准确的结论。主成分分析就是运用线性变换,用少数几个可以充分反映原来样本信息的变量替换原来的指标,从而在不丢掉主要信息的前提下,避开了变量之间共线性的问题,便于进一步分析。
1.2 主成分分析的数学模型
设n个样本包括m个原始变量x1, x2, …, xm,整理得到原始数据矩阵为:
(1)
由于研究实际问题涉及的变量往往具有不同的量纲,可能会影响计算结果,因此,在主成分分析前利用式(2)对原始数据进行标准化处理。
(2)
式中:
和
分别为第j个变量的平均值和标准差;i=1, 2, …, n;j=1, 2, …, m。
数据标准化处理后得到新的变量矩阵y,y可以由原来的变量x1, x2,…, xm线性表示:
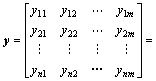
(3)
其中:y1m, y2m, …, ynm表示通过主成分分析后的变量,u为变量之间的相关系数矩阵。并且满足以下条件:
(1)
;
(2)
,即主成分无重叠的信息;
(3)
,即主成分重要性依次递减。
通过以上方法所确定的y1, y2, …, ym即为原变量的第1, 2, …, m个主成分,其方差在总方差中所占比例依次递减。主成分选取个数取决于主成分的累积方差贡献率,累积贡献率越大,丢失的数据信息越少,但神经网络输入变量增多,通常方差贡献率的取值以80%为宜[10]。
2 采空区稳定性分析的BP神经网络模型
2.1 BP神经网络的基本原理
BP神经网络是一种由信号前向传递和误差反向传递2个过程组成的多层前馈神经网络。前向传递中,输入信号从输入层经隐含层逐层处理,直到输出层。若输出层得不到期望的输出,则转入反向传播,网络权值和阀值根据预测误差进行调整,使预测输出逐渐逼近期望输出。通过这2个过程的交替进行,完成信息提取和记忆过程[11-13]。
2.3 BP神经网络模型训练算法
首先给网络提供输入一组样本,通过不断地训练该网络,使其调整、修正各神经元的权值和阀值,直到网络输出能够准确地逼近给定训练样本的输出时,则该网络完成训练过程[13]。设给定N个样本对(Xk, Yk)(k=l, 2, …, N),从输入层到隐层及从隐层到输出层的传递函数f采用Sigmoid函数,即
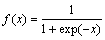
(4)
对于第l层的第i个单元,当输入第m个样本时,正向传播的函数为:
(5)
式中:
为隐层第j个神经元与输入层第i个神经元之间的连接权值;
为隐层节点j的阀值。
BP网络的连接权值和阀值的调整过程也就是误差的反向传播过程。定义误差函数为:
(6)
式中:
和
分别为输出层节点k的期望输出和实际输出。
总误差为:
(7)
任意选取权系数初值,隐层与输入层的权值
调整量为:
(8)
(9)
式中:
(0<μ<1)为学习速率。
同理可得到隐层与输入层及隐层与输出层之间的阀值调整量。重复上述正向和反向传播过程直到E<
(
为给定精度)。
3 工程实例
以锡矿山为例,采用主成分分析法和BP神经网络相结合的方法,对统计到的17个采空区群进行危险性评价。综合考虑了影响采空区稳定性的13项因素,并对其进行了取值,将其作为采空区危险性的评价对象[14-15],具体数据见表1。危险性等级评定标准为:Ⅰ级 (特大危险性);Ⅱ级 (重大危险性);Ⅲ级 (较大危险性);Ⅳ级 (一般危险性)。
表1 锡矿山采空区稳定性影响因素及危险等级调查数据
Table 1 Survey data of risk level and factors affecting goaf stability in Xi mine
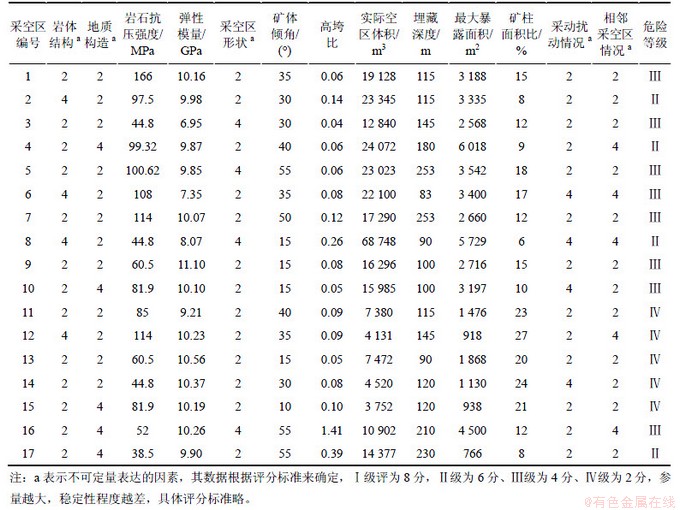
3.1 相关性分析
将影响采空区稳定性的13项因素作为输入因素,危险性等级作为输出因素,利用SPSS软件包中的相关性分析功能对表1中的数据进行相关性分析。输出因素为:危险性等级。相关系数采用Pearson,即皮尔逊相关。显著性检验采用Two-tailed,即双尾T检验。
表2所示为各指标的Pearson相关系数矩阵。由表2和共线性诊断结果(条件指数Φ=173.406)表明:自变量存在严重的共线性,采空区形状、高跨比、空区体积和最大暴露面积的VP(variance proportion)值均很大,极度相关,必定会对BP神经网络预测精度造成影响。因此,有必要对输入数据进行主成分分析。
3.2 数据的主成分分析
由于影响采空区稳定性的样本数据量纲和数据级的不同,使得数据之间的差异比较大,因此要先利用式(4)对数据进行标准化处理见表3。
表2 各指标的Pearson相关系数矩阵
Table 2 Pearson correlation coefficient matrix of each index
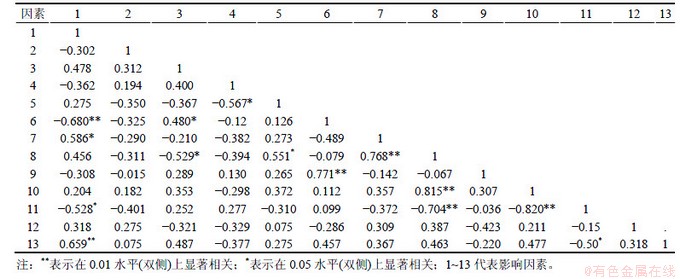
表3 标准化处理后的部分数据
Table 3 Partial standardized data
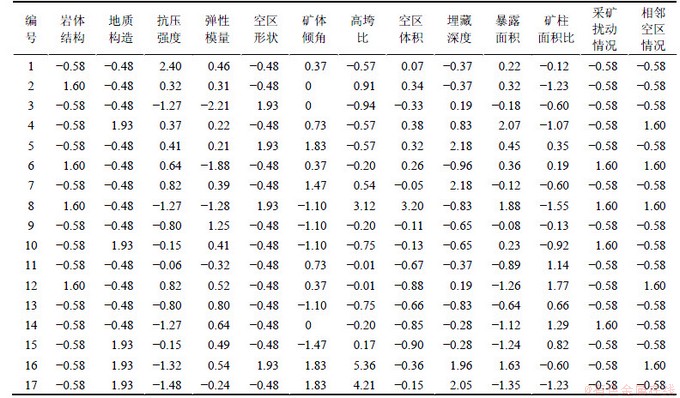
利用SPSS中的主成分分析功能对表3中的数据进行分析,得到主成分碎石图(图1)。从图1可以看出:因子1与2、因子2与3、因子3与4和因子4与5的特征值之差比较大,相对而言其他因子之间的特征值差值较小,可以初步得出提取前5个因子将能概括绝大部分信息。
表4所示为主成分列表。由表4可知:前5个成分足以解释总变异的83.39%,和碎石图显示的信息相吻合,符合主成分方差占总方差80%的要求,因此可以确定选取前5个成分作为主成分,代替原始变量进行分析。
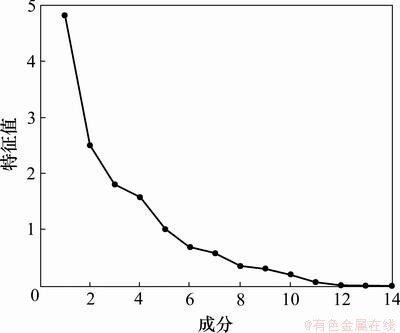
图1 主成分分析碎石图
Fig.1 Scree plot of principal component analysis
表5所示为原始变量与主成分之间的相关系数矩阵,表达了出了主成分1~5与原始变量之间的关系(即式(3)中的u值)。
根据该系数矩阵和式(3)确定因子表达式,对标准化后的数据进行主成分分析计算,结果见表6,即理想的输入变量,将其作为神经网络的输入数据。
3.3 BP神经网络模型的训练与预测
将主成分1~5作为输入因素,危险级别Z作为输出因素。同时,把表5中的数据分为2个样本子集:训练样本子集(1~12)和预测样本子集(13~17)。建模时有关参数选取为:学习率0.9;冲量系数0.7;通过训练最终确定最佳网络结构为7:11:1。经主成分分析及未经主成分分析的神经网络训练过程如图2和3所示。由图2和图3可以看出:经主成分分析后的神经网络学习步骤大大减小,训练速度明显加快。
BP神经网络模型预测结果见表7。可见:13~17号空区的危险性等级均为4级即一般危险。误差分析见图4。由图4可知:实际输出与预期输出的相对误差都控制在8%以内,与未经主成分分析的预测误差有明显降低,说明经过主成分分析后的神经网络模型对采空区危险性等级的预测更为精确。
表4 主成分列表
Table 4 List of principal component
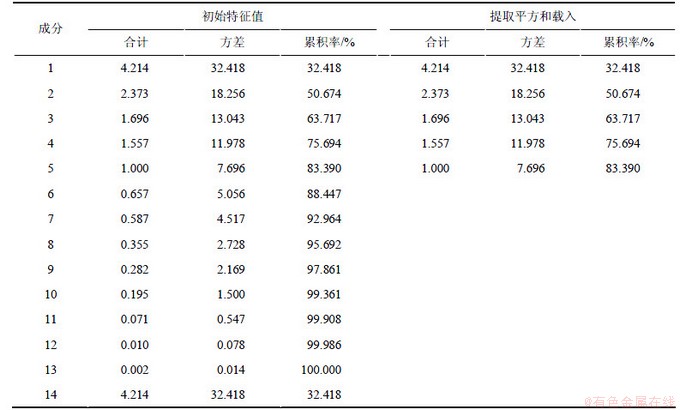
表5 主成分因子荷载矩阵
Table 5 Factor load matrix of principal component
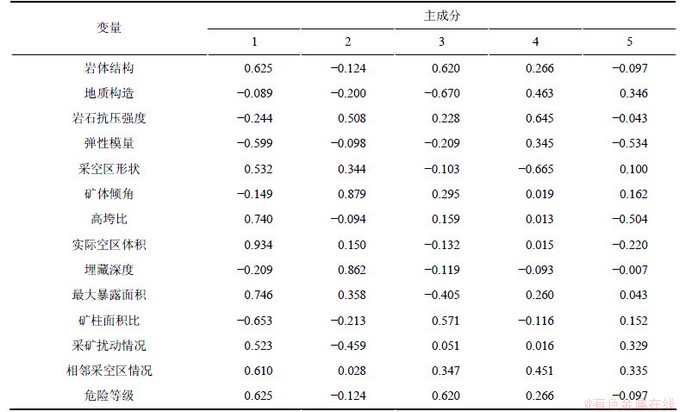
表6 主成分计算后的数据
Table 6 Calculated data of principal component
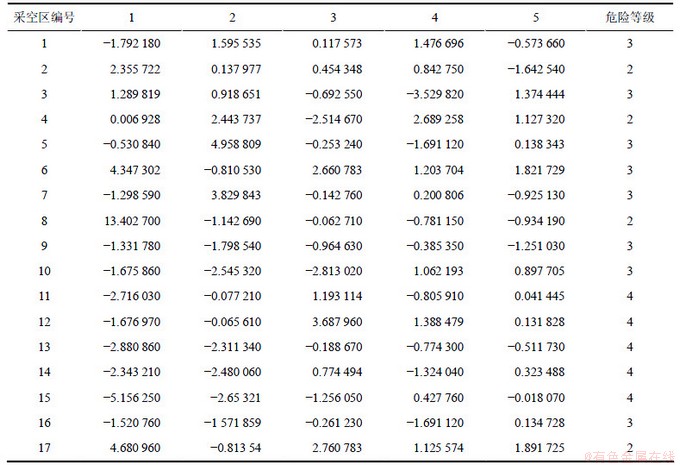
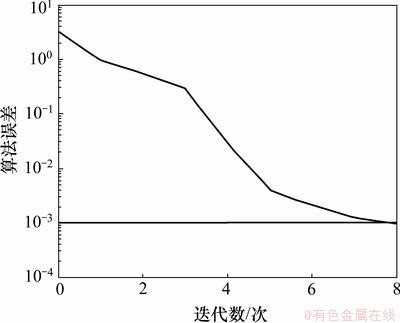
图2 经过主成分分析的神经网络训练过程
Fig.2 Neural network training process with principal component analysis
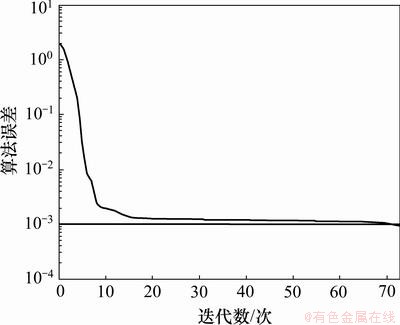
图3 未经主成分分析的神经网络训练过程
Fig.3 Neural network training process without principal component analysis
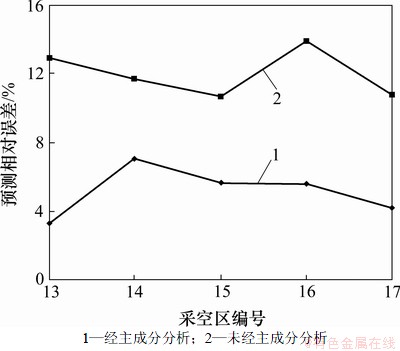
图4 误差分析图
Fig.4 Error analysis diagram
表7 预测结果
Table 7 Prediction results
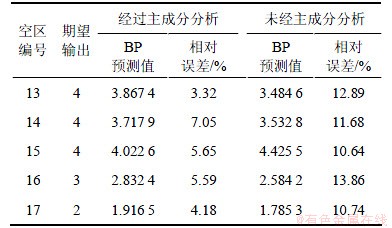
4 结论
(1) 13~17号采空区均为一般危险等级,经主成分分析法处理后的BP网络预测值与期望输出值之间的误差都控制在8%以内,能够较准确地预测采空区的危险性,与未经主成分处理的预测结果相比,误差有明显降低。
(2) BP神经网络具有高度非线性映射能力,可避免传统评价方法的主观性和随机性等。运用统计学主成分分析法对原始样本进行预处理,可减少神经网络的输入变量,加快训练速度,消除变量之间的相关性,从而提高预测精度和效率。
(3) 以锡矿山作为实例,采用主成分分析法和BP神经网络相结合的方法建立模型对采空区危险性等级进行预测,效果良好,为采空区危险性评价提供了一种更加完善的评价方法。
参考文献:
[1] 国家安全生产监督管理总局. 国家安全生产科学规划: 非煤矿山领域研究报告(2004—2010)[R]. 北京: 国家安全生产监督管理总局, 国家煤矿安全监督局, 2003: 8-10.
State Administration of Work Safety. State technological development programming of work safety: Non-coal mine domain research bulletin (2004—2010)[R]. Beijing: State Administration of Work Safety, State Administration of Coal Mine Safety, 2003: 8-10.
[2] 易丽平, 王李管, 童汝彦, 等. 基于模糊评价法的狮凤山铜矿采空区评价与管理[J]. 中国钼业, 2010, 34(5): 19-22.
YI Liping, WANG Liguan, TONG Ruyan, et al. Evaluation and management of Shifengshan copper mine based on fuzzy comprehensive analysis method[J]. China Molybdenum Industry, 2010, 34(5): 19-22.
[3] 薛希龙, 王新民, 刘奇, 等. 基于AHP-灰色聚类模型的采空区危险性分析研究[J]. 湖南科技大学学报: 自然科学版, 2011, 26(2): 5-10.
XUE Xilong, WANG Xinmin, LIU Qi, et al. Underground goaf risk analysis evaluation based on AHP-gray clustering model[J]. Journal of Hunan University of Science & Technology: Natural Science Edition, 2011, 26(2): 5-10.
[4] 王新民, 丁德强, 段瑜. 灰色关联分析在地下采空区危险度评价中的应用[J]. 中国安全生产科学技术, 2006, 2(4): 35-39.
WANG Xinmin, DING Deqiang, DUAN Yu. Applications of the grey relation analysis in the evaluation of the risk degree of the underground mined-out stopes[J]. Journal of Safety Science and Technology, 2006, 2(4): 35-39.
[5] 宫凤强, 李夕兵, 董陇军, 等. 基于未确知测度的采空区危险性评价研究[J]. 岩石力学与工程学报, 2008, 27(2): 323-330.
GONG Fengqiang, LI Xibing, DONG Longjun, et al. Underground goaf risk evaluation based on uncertainty measurement theory[J]. Journal of Rock Mechanics and Engineering, 2008, 27(2): 323-330.
[6] 罗一中. 大面积采空区失稳的重大危险源辨识[D]. 长沙: 中南大学资源与安全工程学院, 2005: 66-86.
LUO Yizhong. The major hazard sources identification in widespread mine-out area[D]. Changsha: Central South University. School of Resources and Safety Engineering, 2005: 66-86.
[7] 王淑红, 李英龙, 戈保梁, 等. 主成分分析法与神经网络在选矿建模中的应用[J]. 有色矿冶, 2001, 17(6): 25-28.
WANG Shuhong, LI Yinglong, GE Baoliang, et al. Application of principal component analysis and neural network model in mineral processing[J]. Non-ferrous Mining and Metallurgy, 2001, 17(6): 25-28.
[8] 陈建宏, 刘浪, 周智勇, 等. 基于主成分分析与神经网络的采矿方法优选[J]. 中南大学学报: 自然科学版, 2010, 41(5): 1967-1972.
CHEN Jianhong, LIU Lang, ZHOU Zhiyong, et al. Optimization of mining methods based on combination of principal component analysis and neural networks[J]. Journal of Central South University: Science and Technology, 2010, 41(5): 1967-1972.
[9] 林杰斌, 刘明德. SPSS10.0与统计模式建构[M]. 北京: 人民统计出版社, 2001: 185-190.
LIN Jiebing, LIU Mingde. SPSS10.0 and construction of statistics model[M]. Beijing: China Statistics Press, 2001: 185-190.
[10] 叶双峰. 关于主成分分析做综合评价的改进[J]. 数理统计与管理, 2001, 20(2): 52-55, 61.
YE Shuangfeng. Application and consideration about principal component analysis[J]. Application of Statistics and Management, 2001, 20(2): 52-55, 61.
[11] 闻新. MATLAB神经网络仿真与应用[M]. 北京: 科学出版社, 2003: 278-283.
WEN Xin. Neural network simulation and application based on MATLAB[M]. Beijing: Science Press, 2003: 278-283.
[12] 范磊, 张运陶, 程正军. 基于Matlab的改进BP神经网络及其应用[J]. 西华师范大学学报, 2005, 26(1): 71-73.
FAN Lei, ZHANG Yuntao, CHENG Zhengjun. Application of improved BP neural network based on MATLAB[J]. Journal of China West Normal University, 2005, 26(1): 71-73.
[13] 伍春香, 刘琳. 三层BP网络隐层节点数确定方法的研究[J]. 武汉测绘科技大学学报, 1999, 24(2): 177-178.
WU Chunxiang, LIU Lin. Study on the method of determining of hidden layer’s nodes for three-layer network[J]. Journal of Wuhan Technical University of Surveying and Mapping, 1999, 24(2): 177-178.
[14] 古德生. 地下金属矿采矿科学技术的发展趋势[J]. 黄金, 2004, 25(1): 18-22.
GU Desheng. The science &technology development of underground mental mining[J]. Gold, 2004, 25(1): 8-22.
[15] 解世俊, 任天贵, 付兆华, 等. 采矿手册: 第4卷[M]. 北京: 冶金工业出版社, 1990: 415-492.
XIE Shijun, REN Tianhua, FU Zhaohua, et al. Mining manual: Vol.4[M]. Beijing: Metallurgical Industry Press, 1990, 415-492.
(编辑 赵俊)
收稿日期:2012-07-06;修回日期:2012-10-15
基金项目:“十一五”国家科技支撑计划项目(2006BAB02A03)
通信作者:王新民(1957-),男,安徽安庆人,教授,从事采矿与充填技术研究;电话:13187011058;E-mail: caiyan0703@126.com