J. Cent. South Univ. Technol. (2010) 17: 744-749
DOI: 10.1007/s11771-010-0550-0
Optimization model of unascertained measurement for underground mining method selection and its application
LIU Ai-hua(刘爱华), DONG Lei(董蕾), DONG Long-jun(董陇军)
School of Resources and Safety Engineering, Central South University, Changsha 410083, China
? Central South University Press and Springer-Verlag Berlin Heidelberg 2010
Abstract:
An optimization model of underground mining method selection was established on the basis of the unascertained measurement theory. Considering the geologic conditions, technology, economy and safety production, ten main factors influencing the selection of mining method were taken into account, and the comprehensive evaluation index system of mining method selection was constructed. The unascertained evaluation indices corresponding to the selected factors for the actual situation were solved both qualitatively and quantitatively. New measurement standards were constructed. Then, the unascertained measurement function of each evaluation index was established. The index weights of the factors were calculated by entropy theory, and credible degree recognition criteria were established according to the unascertained measurement theory. The results of mining method evaluation were obtained using the credible degree criteria, thus the best underground mining method was determined. Furthermore, this model was employed for the comprehensive evaluation and selection of the chosen standard mining methods in Xinli Gold Mine in Sanshandao of China. The results show that the relative superiority degrees of mining methods can be calculated using the unascertained measurement optimization model, so the optimal method can be easily determined. Meanwhile, the proposed method can take into account large amount of uncertain information in mining method selection, which can provide an effective way for selecting the optimal underground mining method.
Key words:
1 Introduction
Underground mining method selection is one of the most important decisions that mining engineers have to make. Choosing a suitable underground mining method to extract a mineral deposit is very important in terms of safety production, increasing ore production, reducing loss rate and ore dilution rate and improving productivity of mining operations [1]. However, underground mining method selection is a complex systematic engineering. The ambiguous factors and lack of geological information make underground mining method selection be of great subjectivity and randomness, which bring complexity and difficulty to the right decision of mining method [2-3].
In fact, traditional underground mining method selection is only determined by a single factor or by the intuitive evaluation of several factors. The evaluation is constrained to human abilities, easily getting subjective and incomplete results. In recent years, many scholars worldwide have introduced many new theories and methods in the selection of underground mining method, which mainly include fuzzy mathematics [4], analytical hierarchy process (AHP) [5-6], gray correlation analysis method [7], gray situation decision making [8], multi-objective decision making [9-10], value engineering [11] as well as artificial intelligence [12]. Fuzzy mathematics and multi-objective decision-making had certain degree of randomness and subjectivity when determining the weight of factors, and gray theory did not take into account the relative important extent of various objectives and factors, while value engineering and artificial intelligence had the disadvantage of narrow knowledge access and poor applicability. The most important thing during the selection process of the best mining method is to take into account large amount of uncertain information. The unascertained mathematics theory can be a new way to do so.
The concept of unascertained information theory was first proposed by WANG [13] in 1990 in the research of architectural engineering, which was different from the fuzzy information, the stochastic information and the gray information. The unascertained measurement optimization model of underground mining method selection was established according to the theory of unascertained measurement. The value of the unascertained measurement of each index was calculated, and the indices weights of the factors were calculated using the entropy theory. Finally, credible recognition criteria were established for the evaluation of each mining method. The superiority degrees of mining methods were arranged in order by the credible degree criteria, thus determining the best underground mining method. The proposed model was used in the underground mining method selection of Xinli Gold Mine in Sanshandao of China and obtained ideal results, which can provide a new way for efficient and optimal selection of underground mining method.
2 Unascertained model of underground mining method optimal selection
2.1 Determining classification model system of optimized objects
Suppose R1, R2, …, Rn are n objects to be optimized, and the optimization object space is R={R1, R2, …, Rn}. Each object of Ri (i=1, 2, …, n) has m evaluating indices, so the evaluating index space is X={x1, x2, …, xm}. Then, Ri can be denoted as m-dimension Ri=…,
where
is the measured value of optimization object Ri with respect to evaluating index xj. For different
the contribution to the optimized result Ri is different.
can be divided into two categories of A and B. For category A, the larger the value of
, the greater the contribution to the superiority degree Q; while the smaller the value, the greater the contribution to that of Q for category B. For each
(i=1, 2, …, n; j=1, 2, …, m), we assume that there are p evaluation grades of C1, C2, …, Cp.
The evaluation space is U, denoted as {C1, C2, …, Cp}. Suppose Ck (k=1, 2, …, p) is the kth evaluation grade, and the kth grade is higher than the (k+1)th one, denoted as Ck>Ck+1. If the grading rank {C1, C2, …, Cp} satisfies C1>C2>C3>…>Cp or C1<C2<C3<…<Cp, {C1, C2, …, Cp} is called the ordered partition class of evaluation space U [14].
2.2 Unascertained measurement of single index
Denote the unascertained measurement as
where
is the degree of
belonging to the kth evaluation grade of Ck, which satisfies
0≤≤1 (1)
(2)
(k=1, 2, …, p) (3)
where Eq.(1) is defined as non-negative bound, Eq.(2) is called normalization and Eq.(3) is called additivity. Then, μ satisfying Eqs.(1)-(3) is called unascertained measurement.
2.3 Determination of index weights
The order degree and effectiveness of the obtained system information can be evaluated using the information entropy [15] when determining the weights of evaluating indices, that is, the weights are determined by the judgment matrix consisting of the value of evaluating indices.
Suppose wj is the relative important extent of measured index Xj compared with other indices, wj satisfies 0≤wj≤1, which is called the weight of xi. Index weight vector w is characterized by w={w1, w2, …, wm}. Then, wj is given by
(4)
(5)
The evaluation matrix of unascertained measurement of single index is known, so wj can be obtained by Eqs.(4) and (5).
2.4 Composite unascertained measurement of multiple indices
μik is denoted as the degree of the optimized object Ri belonging to the kth evaluation grade of Ck. When μik is equal toμik is called the composite unascertained measurement of multiple indices.
Based on the unascertained measurement of single index and index weights, the composite unascertained measurement of multiple indices can be worked out as follows:
(i=1, 2, , n; k=1, 2, …, p) (6)
where μik satisfies 0≤μik≤1 and
2.5 Optimization results recognizing and sequencing
It is necessary to adopt the credible degree identification rule, in order to get the final results of the optimized objects. λ (λ≥0.5) is denoted as the credible degree. If the evaluation space {C1, C2, …, Cp} is orderly and meets C1>C2>…>Cp, let
≥λ, (k=1, 2, …, p)} (7)
Then, Ri belongs to the k0th evaluating grade of Ck0.
Suppose the score value of Cl is Il, is given by
(8)
where is the unascertained superiority degree of optimization object Ri, so
…,
) is called the vector of unascertained superiority degree. The superiority degree of Ri is ordered according to the magnitude of
.
3 Construction of comprehensive evaluation index system of underground mining method
The optimal mining method should be primarily selected to fit to the geology and occurrence conditions of deposit to ensure safety in production. Besides, it should maximize economic and social benefits as much as possible. Therefore, a lot of factors have to be taken into consideration during the selection process of mining method. The degrees of the influence of the factors are different, so all the factors should be weighted in order to reflect the influencing degrees more precisely. On the basis of the related studies [1-2], ten factors affecting the selection of the best mining method are selected as the evaluation indices, which are stope capacity, mining efficiency, mining cost, ore loss rate, ore dilution rate, mining cutting ratio, operation safety degree, ventilation condition, degree of difficulty in implementation and adaptive degree to the change of orebody, designated as X1 , X2 , X3 , X4 , X5 , X6 , X7 , X8 , X9 and X10, respectively. Among these indices, stope capacity (X1), mining efficiency (X2), mining cost (X3), ore loss rate (X4), ore dilution rate (X5) and mining cutting ratio (X6) are evaluated using quantitative data. And the classification standards for these indices are determined with reference to similar mines [1, 6], combined with mining methods, as shown in Table 1. The evaluation set is { C1, C2, C3}, designated classes C1, C2 and C3, which are denoted as poor, medium and superior, respectively. And operation safety degree (X7), ventilation condition (X8), degree of difficulty in implementation (X9) and adaptive degree to the change of orebody (X10) are evaluated by changing non-quantized index into quantized index. In order to avoid one-sidedness and randomness, the classification and value assignment for qualitative indices are decided using expert assessment method. And normalization processing is made for the obtained results, which are given in Table 2. The indices for category A are: stope capacity (X1), mining efficiency (X2), operation safety degree (X7), ventilation condition (X8), degree of difficulty in implementation (X9), adaptive degree to the change of orebody (X10). And the indices for category B are: mining cost (X3), ore loss rate (X4), ore dilution rate (X5) and mining cutting ratio (X6 ). It should also be noted that the values in Tables 1 and 2 are relative values, and the variation of the specific values does not affect the ranking of the superiority of mining methods though it may affect the level of superiority degree.
4 Unascertained measurement optimization model for underground mining method selection and its engineering application
4.1 Engineering conditions
Sanshandao Gold Mine is located in the special industrial area of Sanshandao in Laizhou, Shandong Province, China. Xinli Mine Field is located in the southwest of Sanshandao Gold Mine, which belongs to submarine deposit. The capacity of Xinli Gold Mine is required to reach 6 000 t/d. The ore body is 1 145 m-long in strike, 135-900 m in dip, with a thickness of 0.48- 40.65 m (average thickness of 8.96 m). It presents little undulatory form in strike and dip, the dip of it is southeastward and inclination angle is 33?-67?, with an average dip angle of 46?. The orebody is close to the main controlling fault, which contains fault gouge with a thickness of 5-10 cm. The fractured rock on the hanging wall of the orebody would collapse once exposed. The morphology of orebody is inclined, with the thickness changing greatly, and the boundary between ore body and surrounding rock is unclear. And the ore body has developed joints and fissures. So there is difficulty in maintaining the hanging wall rock and ore body. Therefore, the suitable mining method is extremely important for this complicated ore body.
According to the principle of underground mining method selection, six representative mining methods are selected, combined with the mining technical conditions of Xinli Gold Mine in Sanshandao. The six methods are: in-vein reparatory work and deep and medium hole caving with subsequent filling method (MethodⅠ), mechanized upward horizontal cut and fill stopping (Method Ⅱ), out-vein preparatory work and point-pillar sublevel filling method (Method Ⅲ), room and pillar sublevel filling method (Method Ⅳ), high access back-filling method (Method Ⅴ), and point and pillar individual area filling method (Method Ⅵ). The indicators of the six mining methods are given in Table 3.
Table 1 Value assignment of three-level indicators of evaluation indices in underground mining method selection
The unascertained measurement functions of single index were constructed to get the value of the evaluation factors, on the basis of the definition of the unascertained measurement function and the classification in Tables 1 and 2. The unascertained measurement function of each index is illustrated in Fig.1. Then, the evaluation matrix of unascertained measurement of six mining methods could be obtained, according to the functions in Figs.1(a)-(g) and values of the factors given in Table 3. Taking method Ⅰ for example, the values of the ten evaluation indices for method Ⅰ in Table 3 were substituted into the corresponding unascertained measurement functions in Figs.1(a)-(g), respectively.
Then, the evaluation matrix of unascertained measurement of methodⅠwas calculated as
(9)
4.3 Composite unascertained measurement of multiple indices of mining method
The weights of the indices were determined by Eqs.(1)-(6). So the weights of R1 denoted as {w1, w2, …, w10} were {0.103 4, 0.103 4, 0.103 4, 0.103 4, 0.103 4, 0.069 4, 0.103 4, 0.103 4, 0.103 4, 0.103 4}. Then, the composite unascertained measurement of multiple indices of R1 were calculated as {0.310 2, 0.318 5, 0.371 3}.
Table 2 Classification and value of qualitative indices in underground mining method selection
Table 3 Measured data of mining methods evaluation indices
Fig.1 Unascertained measurement function of evaluation indices: (a) Stope capacity; (b) Mining efficiency; (c) Mining cost; (d) Ore loss rate; (e) Ore dilution rate; (f) Mining cutting ratio; (g) Qualitative indices of operation safety, ventilation condition, degree of difficulty in implementation and adaptive degree to change of orebody
4.4 Optimization results recognizing
The credible recognition rule was adopted to judge the grade of Xi instead of the maximum measurement identification rule, due to the sequence of the evaluating grade {C1, C2 , C3}.
The confidence level was taken as 0.6. According to Eq.(7) of composite unascertained measurement vector of multiple indices and Eq.(8) of credible recognition rule, k0=0.628 7, which was larger than λ in ascending order, thus the superiority degree of R1 belonged to grade C2; when in descending order, k0=0.689 8>λ, the same result could be obtained.
From the above, we can get that the two identified results are in accordance. Therefore, the superiority degree of R1 is determined as grade C2, that is, method Ⅰ is not the most suitable. In the same way, the other mining methods are evaluated. The composite unascertained measurement of multiple indices and the optimized results are listed in Table 4. It shows that the superiority degrees of methodsⅠ- Ⅵ are 2.061 1, 2.038 6, 1.784 8, 1.377 1, 2.082 4 and 2.029 4, respectively.
Table 4 Results of unascertained measurement evaluation
4.5 Analysis of evaluation results
The conclusions can be drawn from Table 4 that the superiority degrees of the mining methods are decreasingly ordered as follows: method Ⅴ, methodⅠ, method Ⅱ, method Ⅵ, method Ⅲ and method Ⅳ. Therefore, method Ⅴ is superior to other methods, which is selected as the best mining method. The practice shows that the chosen mining method is applicable to Xinli Gold Mine and gets high technical and economic benefits.
5 Conclusions
(1) Large numbers of factors are involved in the selection of underground mining method. In this work, ten evaluation indices including stope capacity, mining efficiency, mining cost, ore loss rate, ore dilution rate, mining cutting ratio, operation safety degree, ventilation condition, degree of difficulty in implementation and adaptive degree to the change of orebody are taken into consideration. The comprehensive evaluation index system of mining method selection is constructed. And new measurement standards are constructed. Then the unascertained measurement functions of ten evaluation indices are established.
(2) The optimal selection model of underground mining method is established based on the unascertained measurement theory. During the selecting process, the indices weights of the factors are comparatively determined using the entropy theory, which avoids the disadvantage of difficulty in the weight distribution of so many factors. And then the evaluation results are obtained according to the magnitudes of unascertained superiority degrees.
(3) The unascertained measurement optimization model is applied to the comprehensive evaluation and selection of the preselected standard mining methods in Xinli Gold Mine in Sanshandao. According to the evaluation results of the unascertained measurement optimization model, method Ⅴ is selected as the best mining method. And the result is compared with the practical situation, which shows the high access filling mining method has already made some favorable effects. The model is proved to be reasonable and effective for underground mining method selection, which enriches the methods of mining method selection. Furthermore, the comprehensive evaluation model can also be used for the multi-scheme optimal selection of other system engineering.
References
[1] ALPAY S, YAVUZ M. Underground mining method selection by decision making tools [J]. Tunnelling and Underground Space Technology, 2009, 24(2): 173-184.
[2] WANG Xin-min, ZHAO Bin, ZHANG Qin-li. Mining method choice based on AHP and fuzzy mathematics [J]. Journal of Central South University: Science and Technology, 2008, 39(5): 875-880. (in Chinese)
[3] FREIDIN AM, TAPSIEV AP, USKOV V A, NAZAROVA LA, ZAPOROZHTSEV A A, SERGUNIN MP. Reequipment and development of mining method at Zapolyarny Mine [J]. Journal of Mining Science, 2007, 43(3): 290-299.
[4] GLIGORIC Z, BELJIC C, SIMEUNOVIC V. Shaft location selection at deep multiple orebody deposit by using fuzzy TOPSIS method and network optimization [J]. Expert Systems with Applications, 2010, 37(2): 1408-1418.
[5] GURAY C, CELEBI N, ATALAY V, PASAMEHMETOGLU A G. Ore-age: A hybrid system for assisting and teaching mining method selection [J]. Expert Systems with Applications, 2003, 24(3): 261-271.
[6] NAGHADEHI M Z, MIKAEIL R, ATAEI M. The application of fuzzy analytic hierarchy process (FAHP) approach to selection of optimum underground mining method for Jajarm Bauxite Mine, Iran [J]. Expert Systems with Applications, 2009, 36(4): 8218-8226.
[7] CHEN Ri-hui, XIONG Da-min. Application of grey association analysis in optimization of mining method [J]. Yunnan Metallurgy, 2002, 31(4): 6-9. (in Chinese)
[8] CARVALHO J F, HENRIQUES P, FALE P, LUIS G. Decision criteria for the exploration of ornamental-stone deposits: Application to the marbles of the Portuguese estremoz anticline [J]. International Journal of Rock Mechanics and Mining Sciences, 2008, 45(8): 1306-1319.
[9] ZHANG Chun-lei, XU Shui-tai. Optimum of fuzzy multi-objective decision making in the selection mining methods [J]. Industrial Minerals and Processing, 2005, 34(9): 20-23. (in Chinese)
[10] YAVUZ M, APLAY S. Underground mining technique selection by multicriterion optimization methods [J]. Journal of Mining Science, 2008, 44(4): 391-401.
[11] WU Ai-xiang, ZHANG Wei-feng, GUO Li. Application of the similarity ratio value engineering method in optimization of mining method [J]. Journal of Central South University of Technology: Natural Science, 2000, 31(4): 294-296. (in Chinese)
[12] HUANG X, HASSANI F, LAKSHMANAN V S. Knowledge-base management system for mine design and evaluation [J]. Engineering Applications of Artificial Intelligence, 1994, 7(4): 415-425.
[13] WANG Guang-yuan. Uncertainty information and its mathematical treatment [J]. Journal of Harbin Architecture and Engineering Institute, 1990, 23(4): 52-58. (in Chinese)
[14] DONG Long-jun, PENG Gang-jian, FU Yu-hua, BAI Yun-fei, LIU You-fang. Unascertained measurement classifying model of goaf collapse prediction [J]. Journal of Coal Science and Engineering, 2008, 14(2): 221-224.
[15] SHAMILOY A. Generalized entropy optimization problems and the existence of their solutions [J]. Statistical Mechanics and its Applications, 2007, 382(2): 465-472.
Foundation item: Project(2007CB209402) supported by the National Basic Research Program of China; Project(SKLGDUEK0906) supported by the Research Fund of State Key Laboratory for Geomechanics and Deep Underground Engineering of China
Received date: 2009-12-10; Accepted date: 2010-03-23
Corresponding author: LIU Ai-hua, PhD, Professor; Tel: +86-13755006918; E-mail: alexliu@163.com
- Optimization model of unascertained measurement for underground mining method selection and its application
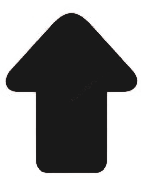