文章编号:1004-0609(2008)05-0917-06
基于BP网络逆映射的铝酸钠溶液软测量模型
童长仁,李明周,吴金财,刘道斌
(江西理工大学 材料与化学工程学院,赣州 341000)
摘 要:
针对铝酸钠溶液成分浓度软测量模型的研究现状,为进一步提高软测量精度和命中率,提出多约束条件求解思想, 建立基于BP神经网络的软测量数学模型。该模型以溶液温度和各成分浓度为网络输入变量,对应电导率为输出变量,运用BP网络误差反向传播、权数调整原理实现在多样本约束条件下的网络逆映射求解。实例验证结果表明,该模型能较好地反映铝酸钠溶液电导率与成分浓度、温度间的内在规律,泛化检验散点电导率平均相对误差为1.74%;在多约束条件下,各软测量浓度与实际浓度的相对误差≤2.5%,且浓度适应范围较宽。该研究为实现铝酸钠溶液在线检测奠定了良好的数模基础。
关键词:
中图分类号:TF 821;TF 355.4 文献标识码:A
Soft sensor model of sodium aluminate solution based on
BP neural network with inverse mapping algorithm
TONG Chang-ren, LI Ming-zhou, WU Jin-cai, LIU Dao-bin
(Faculty of Materials Science and Chemistry Engineering, Jiangxi University of Science and Technology, Ganzhou 341000, China)
Abstract: Considering present soft sensor model research of sodium aluminate solution composition concentration, the multiple restrictive conditions solving thinking was presented to improve its soft-sensing precision and hit rate, and the soft-sensing mathematic model was established based on BP neural network. The sodium aluminate solution temperature and the component concentration were selected as the input nodes, and the corresponding electrical conductivity as the output node. Inverse mapping solution was achieved by combining organically the back-propagation principle and the weights values adjustment principle. Results show that the model can reflect the laws among electrical conductivity, composition concentration and temperature, and the average relative error of electrical conductivity at the generalization test is 1.74%; the relative error between the soft-sensing concentration and the real composition concentration is not more than 2.5%; the model is effective to soft measure the sodium aluminate solution compositon concentration waving in wider range, and thus the research lays a better foundation to achieve measure on-line in sodium aluminate solution.
Key words: BP neural network; inverse mapping algorithm; sodium aluminate solution; soft-sensing model
在拜耳法氧化铝生产过程中,铝酸钠溶液是重要的中间产物,其主要化学成分为氧化铝、苛性钠、碳酸钠[1]。各主要成分的浓度反映了氧化铝生产过程的状况,是工艺参数调整控制的重要判据。因此,分析其化学成分浓度是氧化铝生产过程中一项重要的工作。目前,铝酸钠溶液成分浓度的测定,是通过人工定时取样,采用常规分析方法完成。该方式工作量 大,周期长,结果滞后,不利于及时指导生产。因 此,为实现铝酸钠溶液在线自动检测,开展铝酸钠溶液软测量[2-6]模型研究,对提高拜耳法氧化铝生产过程作业水平具有重要的现实意义。本文作者在分析铝酸钠溶液软测量模型研究现状的基础上,提出并建立了基于BP人工神经网络(ANN)[7-10]、采用多约束条件逆映射求解算法的铝酸钠溶液成分软测量数学模型。
1 铝酸钠溶液软测量数模现状
由于在铝酸钠溶液的各种物理性质中,电导率具有与其主要成分关联性强、易测量之优点,所以,目前国内研究者普遍选用电导率作为铝酸钠溶液软测量的辅助变量。在该类软测量数模中,主要有两温两电导和三温三电导数学模型[11-14],前者用于估计氧化铝和苛性钠2个主要成分浓度,后者可估计氧化铝、苛性钠、碳酸钠3个成分浓度。从建模原理来看,这2种模型并无本质区别,两者均采用泰勒二次展开式,对铝酸钠溶液的电导率-成分浓度、温度数据样本进行回归,从而得到以成分浓度和温度为自变量的电导率函数表达式:
在2个或3个温度点测定溶液电导率,将电导率γi和对应温度ti代入上式,得到一个二元或三元非线性方程组,求解该方程组,可得待测溶液的成分浓度估计值。由此可见,在此类数模中,其求解过程的约束条件数等于待求变量数,为经典的等约束条件求解过程。
笔者基于BP人工神经网络,采用多约束条件逆映射求解算法,建立了一种新的铝酸钠溶液成分浓度软测量数学模型。多约束条件指的是约束条件数大于待求变量数,约束条件数的增加有利于提高软测量输出的精度和命中率。
2 多约束逆映射铝酸钠溶液软测量BP模型
2.1 网络输入输出模式的确定
对于以电导率为辅助变量的铝酸钠溶液软测量系统来说,输入变量为待测溶液在n个温度下的电导率γi,输出变量为m个待测成分浓度ρj。网络输入输出模式可以与其相同,但也可不同。图1和2给出了2种网络输入输出模式。
图1 输入输出模式结构A
Fig.1 Construction of In & Out Model A
图2 输入输出模式结构B
Fig.2 Construction of In & Out Model B
在图1中,网络与系统具有相同的输入输出模式。在该模式下,m个待测成分浓度ρj直接由网络正向运算给出,系统响映速度较快,但该模式存在以下缺点:
1) 输入输出结点数目较多,网络训练较为困难;
2) 对训练样本要求高,每一样本都应由n个一定温度下的电导率和成分浓度组成;
3) 约束条件数(等于输入结点数n)固定,灵活性和自适应性差。
在图2中,网络以1个温度ti和m个成分浓度ρj为输入变量,对应的电导率γi为输出变量。在该模式下,样本组织灵活,便于采用正交试验获取输入输出训练样本;同时,输入输出结点数较少(为m+2),网络训练较容易。
本研究采用图2所示模式。在该模式中,输出变量并非待测成分浓度,此时网络的作用只是用来揭示溶液电导率与成分浓度、温度之间的内在规律。作者利用多条件约束BP网络逆映射求解算法,实现了从温度、电导到溶液成分浓度的转换。
2.2 多约束条件的BP网络逆映射原理
多约束条件的BP网络逆映射指的是:将溶液的一组温度-电导率数据作为约束条件,通过BP神经网络逆映射,求出满足网络内部蕴含规律的各成分浓度ρj。
通常认为BP神经网络不具备逆映射能力,但只要运用BP网络的误差反向传播权数调整原理[15-16],就能够方便地实现这一功能,方法如下:在各网络输入变量ρj结点前添加一公共虚拟结点作为新的输入结点,该结点输入值恒为1,原各输入结点分别与新输入结点相连。这样,原来的各成分输入ρj成为对应的连接权值,记为(见图3)。将多个温度-电导率测点数据样本作为约束条件,保持其它网络权数(在训练时已确定)不变,按BP网络的误差反向传播权数调整原理,反复调整
,当
在一定精度下满足所有测点样本约束条件时,
就是待求的溶液成分浓度ρj。
图3 BP逆映射神经网络
Fig.3 BP inverse mapping ANN
显然,在利用权数调整法实现网络逆映射过程中,测点样本数目可任意增加,即约束条件数灵活可变。在实际的软测量过程中,可根据实际情况的需要,灵活调整测点温度和测点数目。增加测点数目有利于提高软测量输出的精度和命中率。
3 铝酸钠溶液软测量数模实例
3.1 样本数据
本实例采用的97条样本数据摘自《氧化铝生产手册》[17] ,数据变化范围为:ρA 120~320 g/L;ρk 108~320 g/L;t 30~80 ℃;电导率γ:0.033~0.51 S/cm。为了检验模型的散点电导率泛化能力和多点约束逆映射软测量效果,使用69条样本作为训练样本集(见表1),其余样本作为泛化检验样本集(见表2)。
3.2 网络结构
输入层结点数:由于样本数据为2组分铝酸钠溶液数据,所以,输入层结点数等于3,分别为苛性钠浓度ρk、氧化铝浓度ρA、温度t;
输出层结点数:等于1,为电导率γ;
隐层数及隐层结点数:经试算,取隐层数为2,各隐层结点数为8。
3.3 网络训练与效果检验
按表1中样本数据对网络进行训练,相对误差控制为1%。并按表2中样本数据对网络进行泛化效果检验,平均相对误差(绝对值)为1.74%。由此可见,网络泛化效果较好。表3所示是多约束条件逆映射软测量检验结果。表中的每一数据行对应一次软测量行为,表中第1,3,5,6,8,10号测试提供了5个温度下的电导率作为软测量约束条件,第2和第7号提供了4个,其他测试提供了3个,均大于待求变量数2;各测试样本的软测量估计浓度与实际浓度相对误差(绝对值)均不大于2.5%,可见,该模型软测量效果良好。
图4所示为表3中第8和第11号软测量所对应的网络输出特性曲线,图5所示为第2和第12号对应的网络输出特性曲线。所谓网络输出特性曲线,指的是某一特定成分浓度的铝酸钠溶液电导率与温度的关系曲线。一般来说,不同成分浓度的铝酸钠溶液具有不同的电导率-温度特性曲线。图中的各黑点对应于软测量过程的输入条件,即各不同测点温度下的电导率;而对应的曲线则代表数模在该多点约束条件下,按网络蕴含规律所求得的最佳匹配曲线,该曲线对应的成分浓度就是软测量的待求溶液成分浓度。
表1 BP神经网路训练样本
Table 1 Training samples of BP artificial neural network
表2 散点电导率泛化检验结果
Table 2 Generalization analysis results of dispersal points electrical conductivity
表3 多点约束逆映射软测量检验结果
Table 3 Analysis result of inverse mapping with multiple constraints soft sensor
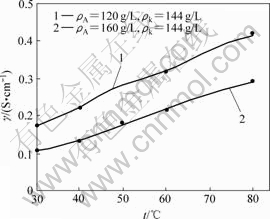
图4 不同氧化铝浓度样本电导率与温度的关系
Fig.4 Relations between electrical conductivity and temperature for samples in different concentrations of alumina
图5 不同苛性钠浓度样本电导率与温度的关系
Fig.5 Relations between electrical conductivity and temperature for samples in different concentrations of caustic soda
4 结论
1) 基于BP神经网络的铝酸钠溶液软测量模型,较好地反映了溶液电导率与成分浓度、温度间的内在规律。拟合精度高,泛化能力强,泛化检验散点电导率平均相对误差为1.74%。
2) 多约束条件逆映射求解算法软测量精度较高,输出估计浓度与实际浓度的相对误差不大于2.5%。
3) 模型对铝酸钠溶液成分浓度适应范围较宽。在本文实例中,ρA为120~320 g/L,ρk为108~320 g/L。
4) 所建立的模型为实现铝酸钠溶液成分浓度在线检测奠定了良好的数模基础。
REFERENCES
[1] 杨重愚. 轻金属冶金学[M]. 北京: 冶金土业出版社, 1991: 21-30.
YANG Chong-yu. Light metals metallurgy[M]. Beijing: Metallurgy Industry Press, 1991: 21-30.
[2] 徐 敏, 俞金寿. 软测量技术[J]. 石油化工自动化, 1998(2): 1-3.
XU Min, YU Jin-shou. Soft-sensing technique[J]. Automation in Petro-Chemical Industry, 1998(2): 1-3.
[3] THAM M T, MONTAGUE G A. Soft-sensors for process estimation and inferential control[J]. J Process Control, 1991, 1(1): 3-14.
[4] 朱学峰. 软测量技术及其应用[J]. 华南理工大学学报: 自然科学版, 2002, 30(11): 61-67.
ZHU Xue-feng. Soft-sensing technique and its applications[J]. Journal of South China University of Technology: Natural Science, 2002, 30(11): 61-67.
[5] 李 凌. 软测量过程中的若干建模方法研究[J]. 沈阳化工学院学报, 2006, 20(2): 118-120.
LI Ling. Study some modelling methods of soft-sensing process[J]. Journal of Shenyang Institute of Chemical Technolgy, 2006, 20(2): 118-120.
[6] 孔建益, 李公法, 熊禾根, 蒋国璋, 杨金堂, 王兴东, 侯 宇. 工业生产中软测量建模方法及其应用研究[J]. 机床与液压, 2007, 35(6): 149-151.
KONG Jian-yi, LI Gong-fa, XIONG He-gen, JIANG Guo-zhang, YANG Jin-tang, WANG Xing-dong, HOU Yu. Research on soft-sensing modeling methods and its application in industrial production[J]. Machine Tool & Hydraulics, 2007, 35(6): 149-151.
[7] 刘瑞兰, 苏宏业, 褚 健. 模糊神经网络的混合学习算法及其软测量建模[J]. 系统仿真学报, 2005, 17(12): 2878-2881.
LIU Rui-lan, SU Hong-ye, CHU Jian. Fuzzy neural network based on hybrid learning algorithm and its application to soft sensor[J]. Journal of System Simulation, 2005, 17(12): 2878-2881.
[8] 刘载文, 王正祥, 王小艺, 杨 斌, 程志强. 过程神经元网络学习算法及软测量方法的研究[J]. 系统仿真学报, 2007, 19(7): 1456-1459.
LIU Zai-wen, WANG Zheng-xiang, WANG Xiao-yi, YANG Bin, CHENG Zhi-qiang. Study on training algorithms and soft sensing method of process neural network[J]. Journal of System Simulation, 2007, 19(7): 1456~1459.
[9] Venkatasubramanian V, King C. A neural network methodology for process fault diagnosis[J]. American Institute of Chemical Engineerrs Journal, 1989, 35(12): 1993-2002.
[10] 常玉清, 王小刚, 王福利. 基于多神经网络模型的软测量方法及应用[J]. 东北大学学报: 自然科学版, 2005, 26(6): 519-522.
CHANG Yu-qing, WANG Xiao-gang, WANG Fu-li. Multi neural network method for soft sensing and its application[J]. Journal of Northeastern University: Natural Science, 2005, 26(6): 519-522.
[11] 张明健. 基于三温三电导法的铝酸钠溶液在线实时测量[D]. 辽宁工程技术大学, 2004: 6-17.
ZHANG Ming-jian. On-line real-time measuring of sodium aluminum solution on three-temperature three-conductivity method[D]. Liaoning Technical University, 2004: 6-17.
[12] 张 石, 张宏勋, 毕诗文, 杨毅宏. 三参数法在线实时测量铝酸钠溶液的化学成分[J]. 东北大学学报: 自然科学版, 1996, 17(3): 243-247.
ZHANG Shi, ZHANG Hong-xun, BI Shi-wen, YANG Yi-hong. Three parameter method for the real time concentration measurement of industrial sodium aluminate solution[J]. Journal of Northeastern University: Natural Science, 1996, 17(3): 243-247.
[13] 李志宏, 杜 娟, 马 莹, 张 平, 路铁桩, 张 石. 铝酸钠溶液化学成分实时测量系统设计及应用[J]. 仪器仪表学报, 2005, 26(10): 1019-1022.
LI Zhi-hong, DU Juan, MA Ying, ZHANG ping, LU Tie-zhuang, ZHANG shi. Design and application of the real time system for measuring the concentrations of the chemical composition of sodium aluminum solution[J]. Chinese Journal of Scientific Instrument, 2005, 26(10): 1019~1022.
[14] 黄迎春, 李新光, 路铁桩, 张 石, 张 平. 铝酸钠溶液成分浓度在线测定系统数学模型的建立与求解[J].控制与决策, 2004, 19(1): 111-113.
HUANG Ying-chun, LI Xin-guang, LU Tie-zhuang, ZHANG shi, ZHANG ping. Model and resolution of the on-line system for measuring the densities of the composition of sodium aluminum solution[J]. Control and Decision, 2004, 19(1): 111-113.
[15] 王洪元. 人工神经网络技术及其应用[M]. 北京: 中国石化出版社, 2002: 29-38.
WANG Hong-yuan. Technologies and applications of artificial neural network[M]. Beijing: China Petrochemical Press, 2002: 29-38.
[16] 张卫华, 梅 炽, 鄂加强, 刘湖滨. 铜精炼炉保温过程铜液温度软测量模型及应用[J]. 冶金自动化, 2005(1): 19-23.
ZHANG Wei-hua, MEI Chi, E Jia-qiang, LIU Hu-bin. Soft-sensing model for copper liquid temperature of heat preservation phase in copper refining rotary anode furnace and its application[J]. Metallurgical Industry Automation, 2005(1): 19-23.
[17] AGELANOFSJI A A. 氧化铝生产手册[M]. 沈阳铝镁设计院. 北京: 冶金工业出版社, 1974: 46-47.
AGELANOFSJI A A. Manuals of alumina production[M]. Shenyang Aluminum and Magnesium Engineering and Research Institute. Beijing: Metallurgy Industry Press, 1974: 46-47.
收稿日期:2007-05-25;修订日期:2008-01-16
通讯作者:童长仁,教授;电话:0797-8313034;E-mail: Tong_CR@163.com
(编辑 陈爱华)
摘 要:针对铝酸钠溶液成分浓度软测量模型的研究现状,为进一步提高软测量精度和命中率,提出多约束条件求解思想, 建立基于BP神经网络的软测量数学模型。该模型以溶液温度和各成分浓度为网络输入变量,对应电导率为输出变量,运用BP网络误差反向传播、权数调整原理实现在多样本约束条件下的网络逆映射求解。实例验证结果表明,该模型能较好地反映铝酸钠溶液电导率与成分浓度、温度间的内在规律,泛化检验散点电导率平均相对误差为1.74%;在多约束条件下,各软测量浓度与实际浓度的相对误差≤2.5%,且浓度适应范围较宽。该研究为实现铝酸钠溶液在线检测奠定了良好的数模基础。