DOI: 10.11817/j.issn.1672-7207.2017.03.017
基于数据驱动的回转支承性能退化评估方法
封杨,黄筱调,洪荣晶,陈捷
(南京工业大学 机械与动力工程学院,江苏 南京,210009)
摘要:为解决工程机械中大型回转支承工作环境恶劣,但可靠性要求较高的问题,提出一种基于集合经验模态分解-主成分分析(ensemble empirical mode decomposition-principle component analysis,EEMD-PCA)的振动信号降噪和性能退化过程评估方法。结合EEMD和PCA各自优势自适应地选择全寿命振动信号中最能反映回转支承性能退化趋势的成分进行重构以实现降噪,提出以PCA模型中的统计量连续平方预测误差(continues square prediction error,C-SPE)作为回转支承的性能退化特征,建立回转支承性能退化模型。利用自制的回转支承性能试验台对型号为QNA-730-22的回转支承进行全寿命疲劳试验。研究结果表明,该方法具有较好的降噪效果,建立的性能退化模型准确地反映出不同阶段回转支承的性能特征,为企业的主动维护提供了数据支撑,从而进一步提高了回转支承的运行可靠性。
关键词:回转支承;性能退化模型; EEMD-PCA; 数据融合
中图分类号:TP206.3 文献标志码:A 文章编号:1672-7207(2017)03-0684-10
A multi-dimensional data-driven method for large-size slewing bearings performance degradation assessment
FENG Yang, HUANG Xiaodiao, HONG Rongjing, CHEN Jie
(School of Mechanical and Power Engineering, Nanjing Tech University, Nanjing 210009, China)
Abstract: A large-size slewing bearing is usually used in extremely heavy load conditions, and its reliability plays a critical role in machinery performances. Therefore, an ensemble empirical mode decomposition-principle component analysis (EEMD-PCA) based de-noising and performance degradation assessment method was proposed. Firstly, an improved EEMD-PCA based method was conducted on life cycle vibration signals of slewing bearings for de-noising and reconstruction. Afterwards, the reconstructed signals were processed by the PCA, and continues square prediction error (C-SPE) was introduced to represent the performance degradation feature for performance degradation model establishment. The results show that the proposed method is better in unstable signal de-noising than the EEMD-MSPCA, and the established performance degradation model can accurately explain the slewing bearing performance degradation process, which helps enterprises to achieve active maintenances, and provides a potential for further research such as slewing bearing prognostics.
Key words: slewing bearing; performance degradation model; EEMD-PCA; data fusion
机械设备在长期的服役过程中其性能会逐渐衰退直至发生严重故障导致失效,在这一过程中,设备通常会经历多个性能退化状态[1]。大型回转支承作为核心回转连接件常用于风力发电机、挖掘机、起重机等大重型设备中,一旦出现突然的断齿、卡死甚至内外圈滑落,便会引起严重的设备故障,导致巨大的损失。因此,建立回转支承性能退化模型,以便在不同时段判断回转支承性能退化的程度,对制定有效的主动维护或更换策略有重要的意义。设备退化模型的建立通常包含2部分工作:信号降噪与特征提取。大型回转支承转速很低,且工作背景噪声大,各部件产生的非平稳随机信号中的故障通过频率[2]可低至1 Hz以下,这导致其振动信号的信噪比很低,一般的降噪方法很难达到理想效果[3]。对此,MATEJ等[4-5]提出了基于EEMD-MSPCA的降噪方法,先计算出信号的各个经验模态函数(intrinsic mode function,IMF),然后确定相关阈值对其滤波,再选择合适的IMF进行信号重构,获得了较好的降噪效果,但是其降噪效果取决于阈值计算和IMF选择过程中的经验参数,而实际应用中很难确定这些参数的最优选择。与之类似,YU等[6-7]先用EMD分解信号,然后用PCA选取IMF主元,再分别利用自组织映射和Savitzky–Golay自适应滤波处理降维后的特征向量,以分别实现性能退化趋势建立和噪声频谱能量分析,但是回转支承振动信号多被噪声淹没,其EMD分解后的前几阶IMF中多为噪声成分且能量较高,直接使用PCA会误选这些IMF分量。贾峰等[8]结合EMD和多重分形去趋势法(MFDFA)去除振动信号的趋势项,然后使用SVM实现轴承的故障诊断。潘玉娜等[9-10]分别使用支持向量描述(SVDD)和模糊c均值研究了轴承性能退化的评估方法。但是以上2种方法研究对象都是小型轴承且未考虑信号降噪[1]。武兵等[11-13]在研究设备寿命预测模型时使用了信号的时域、频域、时频域等特征作为设备性能退化趋势的特征向量,得到了较为可靠的性能退化模型。但考虑到大型回转支承直径可达800~5 000 mm,通常会采集多维不同位置的振动数据[4-5],如果按照文献[11-13]中的方法直接处理,会带来维数过多的问题。据此,本文作者提出一种融合了EEMD和PCA的回转支承性能退化过程评估方法,针对回转支承全寿命振动加速度数据,先进行信号分段,然后用EEMD将各段信号分解成多个IMF,再利用PCA比较各个IMF在回转支承整个寿命周期中的变化趋势,选取最能反映回转支承性能退化的部分IMF分量进行信号重构以实现降噪。最后再次运用PCA,对4组正交分布的加速度传感器的重构信号进行处理,提出以连续平方预测误差(continues square prediction error,C-SPE)反映4组信号在整个寿命周期中的变化趋势,实现数据的融合和降维。
1 集合经验模态分解(EEMD)和主成分分析(PCA)
1.1 集合经验模态分解
经验模态分解(EMD)非常适用于分析非平稳信号,将一组信号分解成多个IMF,不同的IMF代表此信号在同一时间序列上不同的频域范围。然而,非平稳信号中的非线性成分会导致在同一IMF中出现多个频带,这种现象被称为“模态混叠效应”。为解决此问题,WU等[14]提出了EEMD方法,在原信号中多次加入高斯白噪声并进行EMD,最后取多个IMF的均值为EEMD的结果。对于一组信号x(t),EEMD步骤如下。
步骤1) 生成随机白噪声n(t),令其幅值水平为
,将其加入原信号,生成新的序列y(t):
(1)
步骤2) 使用EMD将y(t)分解成一组IMF,第h (h∈[1,H])个IMF用fh(t)表示。
步骤3) 将步骤1)和2)重复I次,总共生成I组IMF,设第i (i≤I)次EMD后的第h个IMF用
表示;
步骤4) 以ch(t)表示信号经由EEMD后生成的第h阶IMF,则
(2)
曹冲锋等[15]将EEMD应用于大型旋转机械非平稳振动信号,达到了较好的降噪效果。因此,可将EEMD作为回转支承振动信号的前置处理方法。需要注意的是,使用EEMD时,噪声水平
和重复次数I需要确定,陈忠等[16]指出,噪声水平
常取0.01~0.50,重复次数I可取100~200次。因为在相同的噪声水平下,
的变化并不能显著影响计算结果,而I的过分增大不仅不能改善计算结果,而且易使计算异常缓慢。因此,令
=0.2,I=100较为合适。
1.2 主成分分析
主成分分析(PCA)主要用于处理多变量多样本的试验监测数据,近年来被广泛使用。通过降维处理,将多维数据矩阵投影到主元子空间和残差子空间中,从而用互不相关的几个主元代表所有变量的变化情况。
设矩阵X(m×n)是归一化后的信号矩阵,其中m为每个变量的样本数,n为变量的个数,对X进行PCA后可得:
(3)
其中,tj为m×1的向量,表示第j个主元;pj为n×1的向量,表示第j个载荷向量;E为残差矩阵,多为随机噪声。令主元矩阵为T(m×r),载荷矩阵为P(n×r),则X亦可表示为
(4)
因此,X在残差子空间上投影为
(5)
为判断监测过程的运行情况,Hotelling-T2统计、平方预测误差(SPE)和贡献图常用来反映新测数据的变化情况。研究表明,SPE能够更显著地体现出新测数据与基准数据(正常数据)间的差异[17]。SPE计算公式为
(6)
式中:
为平均预测误差。
为判断新测数据是否出现异常,
的阈值Qα计算如下:
(7)
其中:
为置信度为
的标准正态分布的统计值;
;
;d=1, 2, 3;
为X的协方差矩阵的第j个特征值。
对于向量
,若仅有少量数据大于其控制阈值
,则认为过程运行正常,反之,则认为运行过程可能较正常情况有所变化,因此,将回转支承整个寿命周期的数据与正常数据进行对比,将不同时期的SPE连接起来得到连续SPE(C-SPE),既能反映出回转支承性能退化过程,又能达到数据降维的目的。
2 基于EEMD-PCA的回转支承性能退化模型
2.1 基于EEMD-PCA的非平稳信号降噪方法
EEMD作为纯数字驱动的信号分解方法,对非平稳信号的分解有很强的自适应性,在文献[4-7, 15]中都得到了较好的结果。然而,在处理回转支承振动信号时,现有的方法中[4-5]最佳的过程参数难以确定,而类似文献[6-7]中的方法又会误选包含高频噪声的IMF。为解决这一问题,本文结合EEMD和PCA各自优势提出了一种回转支承振动信号自适应降噪方法,针对全寿命试验数据,利用PCA在状态监测中区分不同数据差异,以SPE为指标定量地分析了各个IMF在整个回转支承寿命周期中的变化趋势,自适应地选择合适的IMF进行信号降噪和重构,如图1所示。
具体步骤如下。
步骤1) 对回转支承进行疲劳寿命试验,获取其整个试验周期的多组振动信号监测数据,取其中某一组信号a,等间隔分成K段,每段取N个数,第k(k∈(1, K])段信号用ak表示。
步骤2) 每段信号进行EEMD,设每次EEMD后产生H个经验模态函数,则信号ak产生的所有经验模态函数为Fhk(h∈[1,H])。
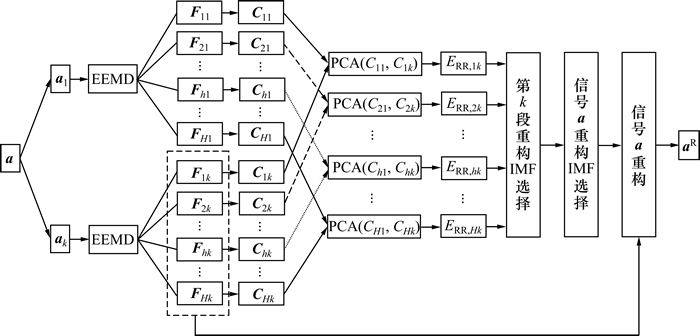
图1 EEMD-PCA算法流程图
Fig. 1 Flow chart of proposed EEMD-PCA
步骤3) 将每段信号产生的经验模态函数Fhk拆分成矩阵Chk,矩阵的维数可任意确定,但是为保证PCA的有效性,建议列数不小于3。
步骤4) 将第k段信号的第h个IMF矩阵Chk与第1段信号的第h个IMF矩阵Ch1进行PCA,得到平方预测误差ESP,hk,若ESP,hk显著大于PCA产生的SPE阈值,则表示相对于正常信号(第1段信号)的第h阶IMF,第k段信号的第h阶IMF随着时间的推移产生了较大的异常;反之,则结论相反。为量化这一比较过程,取ESP,hk均值并减去SPE阈值,差值可称为ERR,计为ERR,hk。显然,ERR,hk越大,表明第k段信号中第h阶IMF越能表现信号的变化趋势(即设备性能的退化趋势)。
步骤5) 由于白噪声的均匀性,包含大量噪声的高频段IMF在不同时段不会有太大变化,因此,其ERR较小,而包含低频有效信息的IMF在不同时段会有较大变化。因此,对于某一确定的k,由式(2)~(4)得到H个误差,将其从大到小排列后,可选取占比超过80%的几个较大的误差对应的IMF进行信号重构,而高频噪声由于较小的误差会被自动舍去,达到降噪的效果。
步骤6) 由于性能退化过程中的随机性,比如不同时期回转支承产生故障的部件会有所不同,这会导致不同时段的信号ak重构所需的IMF会有所不同。为统一信号在所有时间段重构所需的IMF,对所有K组选择的IMF序列进行统计分析,选择其中权值最大的几个IMF用于整个寿命周期的信号重构,得到重构信号aR。
步骤7) 对其余的加速度信号重复步骤2)~6)的操作,得到所有监测振动信号的重构信号,完成信号的降噪和重构。
需要指出的是:现有降噪方法多是针对几秒甚至更短时长的信号进行处理,而本方法针对全寿命振动信号,能够更准确地从信号中提取出最能反映回转支承性能退化过程的成分,从而达到更佳的降噪效果。此外,由以上步骤可知,本方法可根据原始信号特征自适应地调整信号分段、PCA建模、ERR计算和排序以及IMF选择过程中的参数,整个降噪过程无需人工干预,克服了现有方法中需要人为确定部分经验参数的问题。
2.2 回转支承性能退化模型的建立
设备性能退化模型的建立一般包括振动信号时域、频域和时频域的特征提取方法,武兵等[11,13,18]用以上多种方法处理一组振动信号,选择能够较好地反映性能退化过程的指标作为退化特征。然而,仔细观察可以发现,同一组信号的大多数时域、频域和时频域特征趋势非常接近,因此,选取1~2个具有代表性的特征即可。此外,工程机械中的大型回转支承直径通常为400~5 000 mm,仅以1组振动信号的变化情况来反映整个回转支承的性能状态并不妥当。据此,本文利用PCA对多组正交的加速度信号进行对比分析,以C-SPE指标作为回转支承性能退化参数,实现多维数据的融合和降维,建立回转支承性能退化模型。
具体而言,对于试验过程中采集的4组振动信号aⅠ,aⅡ,aⅢ和aⅣ,首先将其降噪和重构,然后按照一定时长将各信号分解成Q段,将各自的第q(q∈[1,Q]))段信号组成矩阵Aq,则
(8)
将Aq逐个与A1进行PCA,通过其SPE反映出第q组数据与第1组之间的差异,差异越大,则表明回转支承的性能退化越严重。接着,将Q组SPE组合起来得到C-SPE,用以反映全寿命试验过程中4组振动信号相对正常信号的变化趋势,最后以C-SPE的相关时域特征建立回转支承性能退化模型。
3 实验验证
3.1 大型回转支承全寿命实验
为验证本文所提方法,采用如图2所示回转支承试验台对型号为QNA-730-22的内齿式回转支承进行全寿命周期的疲劳试验,图2中G1,G2和G3为3个双向液压油缸,其中在G1和G2上施加的力的方向相反,大小不同,从而组合产生轴向力和倾覆力,在G3上产生径向力。由某公司YC60-8挖掘机参数计算可得,此回转支承极限载荷为轴向力96 kN,倾覆力240 kN·m。对此回转支承施以极限载荷,并以4 r/min的转速进行了11 d的满载荷疲劳寿命试验。试验过程中采用4个型号为Kistler-8395A010、响应频率为0~1 000 Hz的加速度传感器,以2 kHz的采样率记录回转支承的径向振动信号,传感器安装位置如图3所示。需要指出的是,4组加速度传感器在尽可能多地收集回转支承运行状态数据的同时,两两相位差90°的安装方式也一定程度上避免了振动数据冗余。此外,试验中还使用温度、扭矩、噪声传感器分别记录了润滑脂温度、驱动力矩以及背景噪声,以辅助判断回转支承的运行状态。
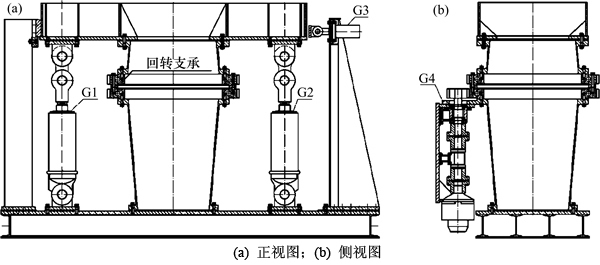
图2 工程机械回转支承综合性能试验台
Fig. 2 Test rig for slewing bearings
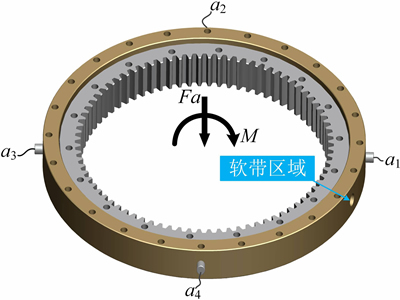
图3 加速度传感器位置示意图
Fig. 3 Distribution of four acceleration transducers
温度和扭矩的变化趋势如图4(a)和4(b)所示。试验初期,回转支承初始磨合,润滑脂温度和驱动力矩持续上升;6 000转后温度和扭矩趋于平衡,而温度随着昼夜温差波动;运行7 d后,回转支承达到国家标准要求的30 000转[19],此时,出现了一根螺栓的疲劳断裂,为了研究回转支承在其寿命达到国家标准时的状态,进行了拆机,发现软带附近滚道由于疲劳出现了整体小幅滑移,如图5(a)所示,但这并不影响回转支承运行,因此,再次装机,更换了失效的螺栓并加注了润滑脂后继续试验,此时,由于停机冷却且润滑条件改善,温度和驱动力矩均大幅度下降;但第9天后,润滑脂温度和驱动力矩均急剧上升,至第11天试验结束时,回转支承已经完全失效卡死,定圈滚道出现了严重的疲劳剥落,部分滚珠甚至产生了疲劳断裂,如图5(b)和5(c)所示。
由轴承部件故障通过频率经验公式[2]可得,被试回转支承最低的故障频率为0.34 Hz,文献[20]指出,用于分析的振动信号的时长不能低于最低故障频率的倒数。此外,在连续的疲劳寿命试验中,深夜的振动数据受环境温度的干扰少。据此,为确保所取振动信号中包含尽量多的信息,11 d试验中每个传感器取每天深夜3 s的(即6 144个样本)数据进行降噪重构并建立回转支承的退化模型。
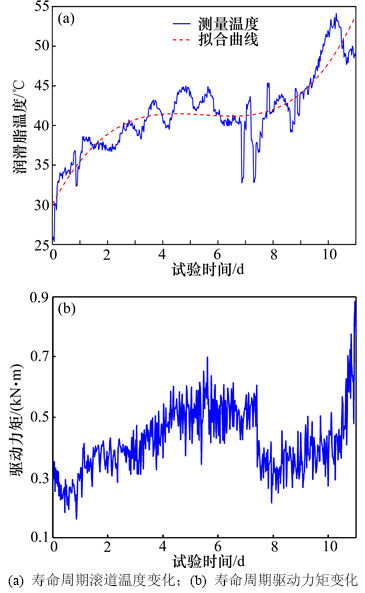
图4 试验过程中温度和驱动力矩的变化
Fig. 4 Changes of both temperature and driven torque during whole test
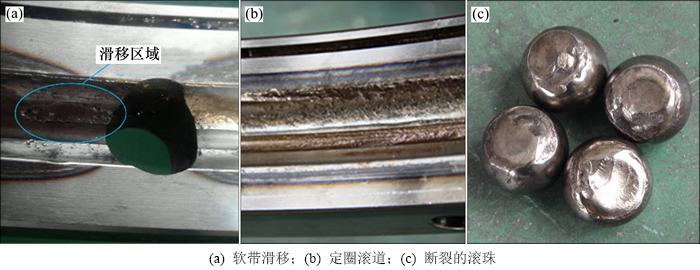
图5 疲劳受损的回转支承部件
Fig. 5 Damaged slewing bearing parts
3.2 大型回转支承退化过程评估
3.2.1 信号降噪与重构
所选取的加速度原始信号如图6所示。从图6可以看出:试验过程中各个加速度信号幅值总体呈增大趋势,但是信号的细节被高频的噪声所覆盖。
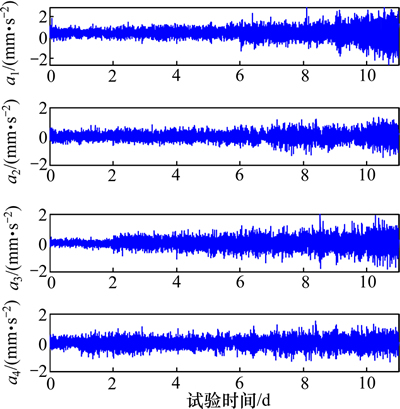
图6 寿命周期加速度a 1~a4原始信号
Fig. 6 Raw life cycle vibration signals
对于加速度a1,取每天的6 144个样本进行EEMD分解,将得到的13个IMF分量组成当天的IMF矩阵,共计算出11个IMF矩阵,以第5天为例进行具体分析,其EEMD分解结果如图7(a)和图7(b)所示。
将第5天的第1阶IMF拆分成三维矩阵M51,同时将第1天第1阶IMF拆分成三维矩阵M11,将M51与M11进行PCA,以M11为基础样本得到M51的平方预测误差向量ESP,51,求其均值并减去阀值
,得到其差值ERR,51。重复以上步骤,计算出第5天13阶IMF各自的误差,最终确定用第6,8,9,10,11,12阶IMF重构第5天的a1信号。据此对每天的a1信号进行相同处理,最终选择序列为5,6,8,11,12的IMF重构出整个寿命周期的振动信号a1。重复以上步骤,即可获得4组加速度全寿命周期信号的重构信号,如图8(b)所示。为进行对比验证,同时采用了MATEJ等[4-5]提出的EEMD-MSPCA进行降噪,结果如图8(a)所示。
由图8(a)可以看出:EEMD-MSPCA重构信号高频的白噪声有所削弱,但由于IMF过滤时最优阈值难以获得,重构的信号仍然被大量噪声淹没;而图8(b)中重构后的加速度信号背景噪声大大降低,清晰地展现了信号的变化情况,在试验中后期可以观察到明显的周期性冲击。对各自的4个加速度重构信号进一步处理,将每天的加速度矩阵与第1天的加速度矩阵进行PCA,最终得到各自的C-SPE,如图9所示。
从图9(a)可知:EEMD-MSPCA重构信号得到的C-SPE中,第2天和第3天由于噪声能量较大导致C-SPE幅值过高,第4天以后呈稳定增长趋势,但并未体现出回转支承失效前期特征幅值陡增的过程;而本文提出的方法噪声消除更彻底,图9(b)中C-SPE在试验初期幅值较小,中期平缓增长,后期急剧升高,符合设备退化过程中信号特征变化的一般趋势。
3.2.2 大型回转支承性能退化模型
首先,按照董绍江等[13]的方法,分别计算降噪信号a1~a4的均方根(RMS)如图10所示。从图10可以看出,不同位置的加速度变化趋势总体符合设备性能退化过程,第3~9天基本平衡,9 d后幅值迅速提高直至失效,但是前3天区别明显,这主要是由于不同位置不同的滚道几何误差、工艺、载荷所导致滚珠与滚道磨合过程存在差异。由此可见,多个与位置互相垂直的加速度传感器安装在大型回转支承上能够提供更多的有效数据,相比常见的单组加速度分析,多维加速度能够更全面地解释大型回转支承的整体性能退化过程。
然而,单组振动信号常见的时域、频域、时频域特征可达30多个,若直接用于处理多维加速度信号,则过多维数的特征会引起后续寿命预测研究中由于过拟合而导致模型精度降低以及耗时过长的问题。因此,进一步研究C-SPE对回转支承整体性能退化过程的评估能力,计算其均方根如图11所示。从图11可以看出,C-SPE的均方根在试验周期中保持持续增长趋势,第1~2天为磨合期,幅值小幅增长,第2~6天为滚道与滚珠均匀磨损期,幅值总体较为平稳,达到国家标准要求的寿命后,在第7~9天进入快速磨损期,然后幅值上升趋势陡增,进入快速失效期直至完全失效,这一过程与试验观察及过程中其他各项测试参数的分析结果一致。因此,C-SPE及其均方根代替多维加速度数据作为此回转支承的性能退化模型,既能准确地评估回转支承的整体性能退化过程,又大幅降低了反映回转支承性能特征所需的维数。
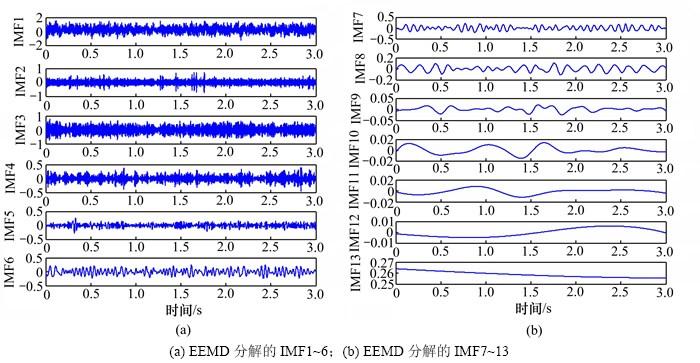
图7 加速度a1第5天信号EEMD分解结果
Fig. 7 IMF 1-13 of a1 on the fifth experiment day
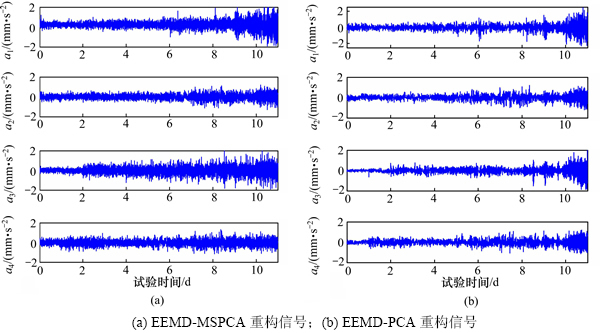
图8 EEMD-MSPCA和EEMD-PCA重构信号对比
Fig. 8 Reconstructed signals of EEMD-MSPCA and EEMD-PCA methods
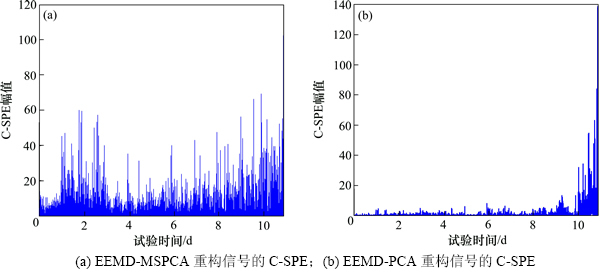
图9 不同方法C-SPE变化趋势对比
Fig. 9 C-SPE of different methods
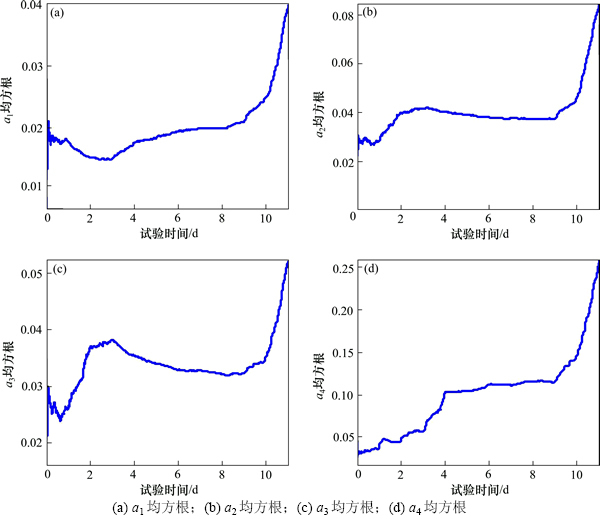
图10 加速度a1~a4的均方根
Fig. 10 RMS of a1~a4
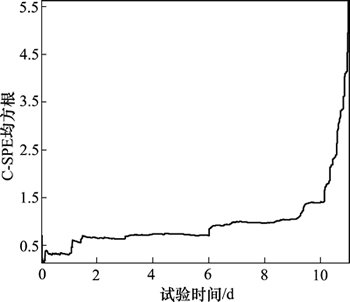
图11 C-SPE均方根
Fig. 11 RMS of C-SPE
4 结论
1) 针对回转支承低频高噪的非平稳振动信号提出了一种基于EEMD-PCA的自适应降噪方法,相比常用的EEMD-MSPCA具有更好的降噪效果,可以应用到回转支承及类似设备产生的非平稳信号中。
2) 利用PCA的C-SPE融合多个加速度传感器信息以全面反映回转支承的性能退化过程,解决了以往使用单组加速度信号信息量不足的问题。该方法建立的回转支承性能退化模型能够准确地评估试验过程中回转支承性能退化过程,符合试验观察。
3) 建立的回转支承性能退化模型能够为企业主动维护规划提供有利的数据支撑,同时为后续的寿命预测研究提供了基础。
参考文献:
[1] 潘玉娜. 滚动轴承的性能退化特征提取及评估方法研究[D]. 上海: 上海交通大学机械与动力工程学院, 2011: 1-3.
PAN Yuna. Study on feature extraction and assessment method of rolling element bearing performance degradation[D]. Shanghai: Shanghai Jiao Tong University. School of Mechanical Engineering, 2011: 1-3.
[2] 万书亭, 吕路勇, 何玉灵. 基于提升模式非抽样小波变换的滚动轴承故障诊断方法研究[J]. 振动与冲击, 2009, 28(1): 170-173.
WAN Shuting, L
Luyong, HE Yuling. Fault diagnosis method of rolling bearing based on undecimated wavelet transformation of lifetime[J]. Journal of Vibration and Shock, 2009, 28(1): 170-173.
[3] 张慧芳, 陈捷. 大型回转支承故障信号处理方法综述[J]. 机械设计与制造, 2012, 3(3): 216-218.
ZHANG Huifang, CHEN Jie. Research on signal processing method of large slewing bearings[J]. Machinery Design & Manufacture, 2012, 3(3): 216-218.
[4] MATEJ Z, SAMO Z, IVAN P. Multivariate and multiscale monitoring of large-size low-speed bearings using ensemble empirical mode decomposition method combined with principal component analysis[J]. Mechanical Systems and Signal Processing, 2010, 24(4): 1049-1067.
[5] MATEJ Z, SAMO Z, IVAN P. Non-linear multivariate and multiscale monitoring and signal denoising strategy using kernel principal component analysis combined with ensemble empirical mode decomposition method[J]. Mechanical Systems and Signal Processing, 2011, 25(7): 2631-2653.
[6] YU Zhang, CHRIS B, YANG Zhijing, et al. Machine fault detection by signal denoising—with application to industrial gas turbines[J]. Measurement, 2014, 58: 230-240.
[7] LU Chen, YUAN Hang, TANG Youning. Bearing performance degradation assessment and prediction based on EMD and PCA-SOM[J]. Journal of Vibroengineering, 2014, 16: 1387-1396.
[8] 贾峰, 武兵, 熊晓燕, 等. 基于EMD与多重分形去趋势法的轴承智能诊断方法[J]. 中南大学学报(自然科学版), 2015, 46(2): 491-497.
JIA Feng, WU Bing, XIONG Xiaoyan, et al. Intelligent diagnosis of bearing based on EMD and multifractal detrended fluctuation analysis[J]. Journal of Central South University (Science and Technology), 2015, 46(2): 491-497.
[9] 潘玉娜, 陈进. 结合循环平稳和支持向量数据描述的轴承性能退化评估研究[J]. 机械科学与技术, 2009(4): 442-445.
PAN Yunna, CHEN Jin. Assessment of bearing performance degradation by cyclostationarity analysis and SVDD[J]. Mechanical Science and Technology for Aerospace Engineering, 2009(4): 442-445.
[10] 潘玉娜, 陈进, 李兴林. 基于模糊c-均值的设备性能退化评估方法[J]. 上海交通大学学报, 2009, 43(11): 1794-1797.
PAN Yunna, CHEN Jin, LI Xinglin. Fuzzy c-means based equipment performance degradation assessment[J]. Journal of Shanghai Jiaotong University, 2009, 43(11): 1794-1797.
[11] 武兵, 林健, 熊晓燕. 基于支持向量回归的多参数设备故障预测方法[J]. 振动、测试与诊断, 2012, 32(5): 791-795.
WU Bing, LIN Jian, XIONG Xiaoyan. Equipment fault prediction method based on multi-variable SVM[J]. Journal of Vibration, Measurement & Diagnosis, 2012, 32(5): 791-795.
[12] 鞠华, 沈长青, 黄伟国, 等. 基于支持向量回归的轴承故障定量诊断应用[J]. 振动、测试与诊断, 2014, 34(4): 767-771.
JU Hua, SHEN Changqing, HUANG Weiguo, et al. Quantitative diagnosis of bearing fault based on support vector regression[J]. Journal of Vibration, Measurement & Diagnosis, 2014, 34(4): 767-771.
[13] 董绍江. 基于优化支持向量机的空间滚动轴承寿命预测方法研究[D]. 重庆: 重庆大学机械工程学院, 2012: 41-60.
DONG Shaojiang. Research on space bearing life prediction method based on optimized support vector machine[D]. Chongqing: Chongqing University. School of Mechanical Engineering, 2012: 41-60.
[14] WU Z, HUANG N E. Ensemble empirical mode decomposition: a noise-assisted data analysis method[J]. Advances in Adaptive Data Analysis, 2009, 1(1): 1-41.
[15] 曹冲锋, 杨世锡, 杨将新. 大型旋转机械非平稳振动信号的EEMD降噪方法[J]. 振动与冲击, 2009, 28(9): 33-38.
CAO Chongfeng, YANG Shixi, YANG Jiangxin. De-noising method for non-stationary vibration signals of large rotating machineries based on ensemble empirical mode decomposition [J]. Journal of Vibration and Shock, 2009, 28(9): 33-38.
[16] 陈忠, 符和超. 基于EEMD方法的永磁同步电机电磁噪声诊断[J]. 振动与冲击, 2013, 32(20): 124-128.
CHEN Zhong, FU Hechao. Electromagnetic noise diagnosis for a permanent magnet synchronous motor based on EEMD[J]. Journal of Vibration and Shock, 2013, 32(20): 124-128.
[17] 许丽, 张进明, 张广明, 等. 基于PCA的滚动轴承故障检测方法[J]. 计算机仿真, 2010, 27(6): 325-329.
XU Li, ZHANG Jinming, ZHANG Guangming, et al. A method of rolling bearing fault detection based on PCA[J]. Computer Simulation, 2010, 27(6): 325-329.
[18] 申中杰, 陈雪峰, 何正嘉. 基于相对特征和多变量支持向量机的滚动轴承剩余寿命预测[J]. 机械工程学报, 2013, 49(2): 183-189.
SHEN Zhongjie, CHEN Xuefeng, HE Zhengjia, et al. Remaining life predictions of rolling bearing based on relative features and multivariable support vector machine[J]. Journal of Mechanical Engineering, 2013, 49(2): 183-189.
[19] JB/T 2300—1999, 回转支承[S].
JB/T 2300—1999, Slewing bearing [S].
[20] WAHYU C, PRABUONO B K, ANH K T, et al. Condition monitoring of naturally damaged slow speed slewing bearing based on ensemble empirical mode decomposition[J]. Journal of Mechanical Science and Technology, 2013, 27(8): 2253-2262.
(编辑 赵俊)
收稿日期:2016-03-06;修回日期:2016-06-12
基金项目(Foundation item):国家自然科学基金资助项目(51375222, 51105191) (Projects(51375222, 51105191) supported by the National Natural Science Foundation of China)
通信作者:黄筱调,教授,从事先进数控理论、设备健康监测研究;E-mail: njgdhxd@yeah.net