DOI: 10.11817/j.issn.1672-7207.2020.08.012

基于改进粒子群深度神经网络的频率域航空电磁反演
廖晓龙1,张志厚1,范祥泰1,路润琪1,姚禹1,曹云勇2,徐正宣1, 2
(1. 西南交通大学 地球科学与环境工程学院,四川 成都,611756;
2. 中铁二院成都地勘岩土工程有限责任公司,四川 成都,610000)
摘要:传统的梯度反演方法依赖于初始模型选取,且容易陷入局部极小,在一定程度上影响着反演的求解精度和收敛速度,为此,提出一种基于改进粒子群深度神经网络的频率域航空电磁反演方法。首先,通过频率域航空电磁模型正演获取样本数据集;随后,依据样本数据集建立深度神经网络的基本框架,网络的输入为归一化垂直磁场分量,输出为相应地电模型参数;第三,提出一种惯性权重振荡衰减措施在粒子群优化算法的基础上进行改进,以提高粒子群优化算法的全局寻优能力,并利用改进的粒子群优化算法优化深度神经网络的训练过程,得到连接权值与阈值的最优解;最后,将最优的权值与阈值作为网络的初始值,并利用该网络对未知地电模型进行反演测试。利用层状地质模型测试改进粒子群深度神经网络算法、粒子群神经网络算法和单一的神经网络算法的反演效果,并将此方法运用于实测航空电磁数据反演。研究结果表明:本文提出的改进粒子群神经网络算法充分结合了粒子群优化算法的全局寻优性能和深度神经网络的局部寻优性能,在反演过程中能有效避免反演陷入局部极小,寻找到全局最优解,并能准确地反演出地电模型参数;与粒子群神经网络算法和单一的神经网络算法相比,本文提出的方法具有更高的求解精度和收敛速度。
关键词:航空电磁;频率域;反演;改进粒子群优化算法;深度神经网络
中图分类号:P631 文献标志码:A 开放科学(资源服务)标识码(OSID)
文章编号:1672-7207(2020)08-2162-12
Frequency domain airborne EM inversion based on improved particle swarm depth neural network
LIAO Xiaolong1, ZHANG Zhihou1, FAN Xiangtai1, LU Runqi1, YAO Yu1, CAO Yunyong2,XU Zhengxuan1, 2
(1. Faculty of Geosciences and Environmental Engineering, Southwest Jiaotong University,Chengdu 611756, China;
2. Chengdu Geological Survey Geotechnical Engineering Co., Ltd. Chengdu 610000, China)
Abstract: The traditional gradient inversion depends on the selection of initial model and is easy to fall into local minimum, which affects the accuracy and convergence speed of inversion to a certain extent. To solve the problems, a frequency domain airborne EM inversion method based on an improved particle swarm deep neural network was proposed. Firstly, a large number of sample data sets were obtained through model forward modeling. Secondly, the basic framework of the deep neural network was established based on the data sets. The network input was the normalized vertical magnetic field component and the output was the corresponding electrical model parameters. Thirdly, an inertial weight oscillation attenuation method was proposed to improve the global optimization ability of particle swarm optimization algorithm, and the swarm algorithm optimizes the training process of the deep neural network to obtain the optimal solution of the connection weights and thresholds. Finally, the optimal weights and thresholds were used as the initial values of the network which were used to perform inversion tests on the unknown geoelectric model. In this paper, the inversion results of the improved particle swarm deep neural network algorithm(IPSO-DNN), the particle swarm deep neural network algorithm(PSO-DNN) and the single deep neural network algorithm(DNN) were tested by layered geological model, and this method was applied to inversion of measured aeromagnetic data. The results show that the improved particle swarm neural network algorithm can make full use of the global searching capability of particle swarm optimization and the local optimization of deep neural network. In the process of inversion, it can effectively prevent the inversion from falling into the local minimum, find the global optimal solution and accurately reverse the parameters of geoelectric model. Compared with the particle swarm neural network and a single neural network inversion method, this method has higher accuracy and convergence speed.
Key words: airborne EM; frequency domain; inversion; improved particle swarm algorithm; deep neural network(DNN)
1954年,频率域航空电磁法在加拿大应用于矿产勘探,并发挥了重要作用。经过60多年发展,该方法已广泛应用于基础地质调查、油气资源勘探及环境工程等领域[1]。频率域航空电磁的常用成像方法包括质心深度成像法[2]、微分电阻率成像法[3]等,此类方法可快速获得地下典型参数,但成像分辨率较低。为获得更加准确、定量化的地下电性信息,诸多学者对反演进行了研究,包括一维层状介质反演[4-6]、拟二维横向约束反演[7-9]和整体反演[10-11]等,这些研究极大推动了航空电磁反演的实用化,但此类方法依赖初始模型的选取,反演解容易陷入局部极小,对反演的准确性和稳定性有一定影响。近年来,完全非线性反演方法在地球物理数据处理解释中受到高度重视,此类方法对初始模型依赖小,无需计算灵敏度矩阵,有着良好的全局寻优性能。朱凯光等[12-13]研究了时间域航空电磁的神经网络反演;刘力辉[14]等采用深度神经网络进行地震岩相反演;奚先等[15]实现了散射波场的深度学习反演成像;师学明等[16]研究了大地电磁阻尼粒子群优化反演;柳建新等[17]将遗传算法引入大地电磁测深二维反演;胡祖志等[18-19]实现了大地电磁模拟退火反演及人工鱼群反演;殷长春等[20]采用变维数贝叶斯方法对频率域航空电磁资料进行反演。以上研究均取得了很好的成效,可见完全非线性算法在地球物理资料处理中发挥着重要作用,但这些算法的求解精度和收敛速度仍有提升空间。每种反演方法都有其优点及局限性,将不同反演方法的优势结合起来,通常能达到更好的反演效果。高明亮等[21]将免疫遗传算法与BP神经网络结合,实现了二维高密度电法数据反演;陈东敬等[22]使用带模拟退火的拟BP神经网络反演重力资料;戴前伟等[23]结合粒子群优化算法与BP神经网络,进行了电阻率成像非线性反演;王鹤等[24]结合遗传算法与BP神经网络,实现了大地电磁反演,取得了极好的效果。以上研究表明,混合优化算法能兼顾各方法优点,提高反演的精度与速度,保证反演的稳定性。基于此,本文将深度神经网络出色的局部寻优性能与改进粒子群优化算法良好的全局寻优能力相结合,提出了基于改进粒子群深度神经网络的频率域航空电磁反演方法。首先,阐述频率域航空电磁一维正演理论;其次,介绍改进粒子群优化算法优化深度神经网络的过程;第三,利用该方法对理论模型进行反演测试;最后,对实测航空电磁数据进行反演,并将反演结果与Occam反演结果进行对比,以检验此方法的有效性。
1 频率域航空电磁1D正演理论
目前,频率域航空电磁系统主要采用水平共面装置[20],水平层状介质的回线源激发和接收模型如图1所示。根据NABIGHIAN[25],垂直磁偶源接收线圈的垂直磁场分量表达式为
(1)
其中:
(2)
(3)
(4)
式中:
为总场;m为发射磁矩;
为TE极化的反射数;h为飞机的飞行高度;
为零阶贝塞尔函数;r为接收线圈与发射线圈的距离;
为本征面波阻抗;
为本征阻抗;
与
分别为第n层的磁导率和电导率,通常认为大地磁导率等于自由空间的磁导率,即
;
为发射线圈发射角频率;
为第n层的波速。
反演处理中所使用的是总场去除一次场并归一化后的数据[20]:
(5)
式中:
为一次场;d为归一化垂直磁场分量。层状介质电磁响应公式含有零阶贝塞尔函数的广义积分,为保证计算速度与精度,本文采用快速汉克尔变换数值积分进行计算[26]。
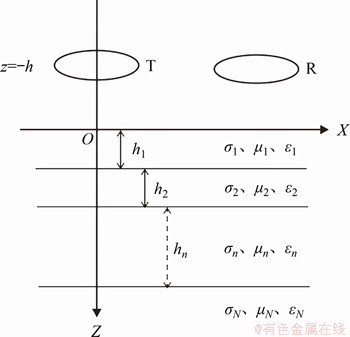
图1 频率域航空电磁层状介质模型示意图
Fig. 1 Schematic diagram of airborne EM layered medium in frequency domain
2 改进粒子群深度神经网络反演
2.1 深度神经网络
深度神经网络(deep neural network,DNN),是近年兴起的一种学习能力极强的机器学习方法,在语音识别、图像识别及自然语言处理等领域应用广泛。它起源于传统的人工神经网络,具备更深的网络深度,能够融合各种非线性特征,具有强大的非线性学习和拟合能力[27]。
DNN由多层自适应的非线性单元组成,每个神经层之间完全连接,通过逐层学习不断减小模型预测误差。图2所示为DNN示意图,由图2可见:输入层节点数M由输入向量维数决定;隐含层节点数目与输入输出节点个数联系紧密;输出层节点数N由输出向量维数决定。
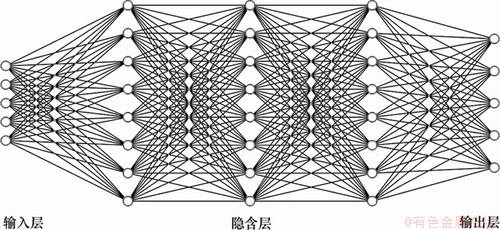
图2 深度神经网络示意图
Fig. 2 Schematic diagram of DNN
DNN的正向传播通过众多的神经元计算实现,工作过程可用式(6)和(7)来表示:
(6)
(7)
式中:
为输入层第i个节点的输入;
为输入层第i个节点与隐含层第j个节点的连接权值;
为隐含层第j个节点的阈值;
为隐含层第j个节点的输出;
为激活函数。常用有以下几种。
(8)
(9)
(10)
DNN的反向传播过程即为网络的反向传播及自我修正过程,代价函数常有2种。
(11)
(12)
式中:
是训练数据的正确输出;M是节点的个数。式(11)为均方误差函数,式(12)为交叉熵函数。由于DNN具有较多的隐含层,训练难度较大,为使代价函数达到最小值而避免陷入局部极值,常用随机梯度下降和Adam等优化方法[28]。
本文采用6层全链接DNN模型,代价函数选择均方误差函数,隐层激活函数使用ReLU函数(能有效改善“梯度弥散”现象),输出层采用sigmoid激活函数。
2.2 粒子群优化算法
粒子群优化算法(particle swarm optimization,PSO)是KENNEDY等[29-30]提出的一种智能优化算法,其基本思想源于对鸟类觅食的研究。假设在1个D维搜索空间中,有m个粒子组成的集群
,第i个粒子的位置为
,第i个粒子的速度为
。在粒子群优化算法迭代进化过程中,粒子会根据个体最优位置和群体最优位置不断调整自身移动方向,再到达下一位置。个体最优位置称为个体极值(Gbest),群体最优位置称为全局极值(Pbest),每个粒子移动的迭代公式如下[31]:

(13)
(14)
式中:t为进化次数;w是惯性权重;
和
分别为学习因子,一般取常数为2;
和
为[0,1]之间的随机数。惯性权重描述了上一代粒子速度对下一代粒子速度的影响,改变其大小可调节算法的全局寻优与局部寻优的能力。w较大有利于提高算法的全局寻优能力;w较小可增强算法的局部寻优性能。学习因子决定着算法的“自我认知”和“社会认知”能力,影响着种群靠近最优区域的能力。
2.3 改进粒子群优化算法优化深度神经网络
邵洪涛等[32-33]研究了粒子群优化算法的改进措施,取得了较好的效果。本文提出一种振荡衰减改进措施,即将惯性权值设置为
(15)
式中:ws和we分别为惯性权值的初值和终值;
为衰减因子;惯性权值
随着迭代次数t振荡衰减。
惯性权重迭代曲线如图3所示,由图3可见:当迭代刚开始时,粒子的惯性权重取值较大,粒子将以较大的飞行速度寻找到全局最优解的范围,保证粒子群优化算法的全局搜索能力;在搜索的后期惯性权重取值较小,粒子的飞行速度较小,进而可以对最优解领域范围进行精确搜索,保证算法的局部搜索能力。同时,在搜索过程中,惯性权重振荡调整,可以不断改变粒子的速度与位置,增强粒子之间的信息交互,避免惯性权重减小导致算法陷入局部最优。
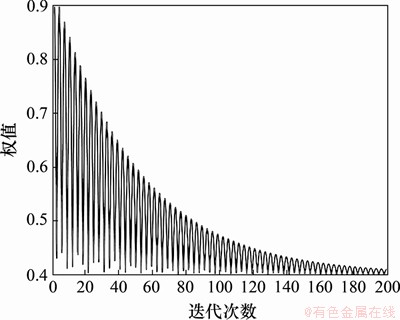
图3 惯性权重迭代曲线
Fig. 3 Inertia weight iteration curve
在求解复杂的非线性问题时,深度神经网络在设置初始权值时依赖于对样本空间反复试验,易产生网络不稳、收敛速度慢以及陷入局部最优等问题。而改进的粒子群优化算法具有良好的全局寻优能力,将其与深度神经网络结合,理论上可以寻找到求解问题的全局最优解。改进粒子群优化算法优化深度神经网络基本流程如图4所示。本文利用改进粒子群优化算法对深度神经网络优化步骤如下。
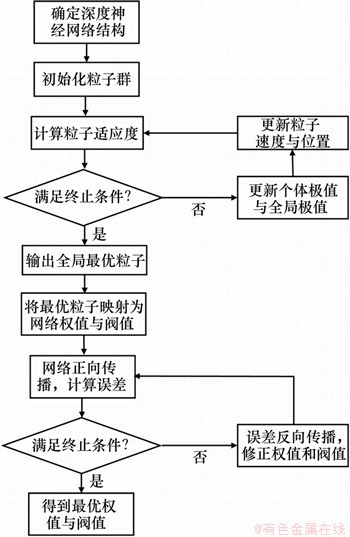
图4 改进粒子群优化深度神经网络流程图
Fig. 4 Flow chart of DNN optimized by IPSO
1) 依据待解决问题,初始化深度神经网络,设定网络的输入层、隐含层和输出层神经元个数,并确定传递函数、学习函数和训练函数。
2) 根据深度神经网络模型确定粒子的维数,使粒子每个维度分量对应神经网络的连接权值与阈值,并随机初始化粒子群。
3) 计算种群中每个粒子的适应度fi。将每一轮最小适应度记为个体极值Gbest,将种群中所有粒子中最小适应度记为全局极值Pbest。
4) 更新个体极值Gbest和全局极值Pbest。在每一轮迭代过程中,若粒子适应度fi小于Gbest,则Gbest=fi;若Pbest小于Gbest,则Pbest=Gbest。
5) 根据式(13)和(14)更新粒子的速度与位移,采用式(15)惯性权重进行改进。按照粒子位置区间[Xmin,Xmax]和速度区间[Vmin,Vmax]规范超出限定范围的粒子。若满足迭代的终止条件,则进行下一步,否则返回步骤3)。
6) 当达到迭代次数或目标精度时,选择最佳粒子作为深度神经网络的初始连接权值和阈值,然后进行网络训练,直到达到最大迭代次数或满足精度要求,算法结束。
3 模型试验
本文分别采用改进的粒子群深度神经网络(IPSO-DNN)、标准粒子群深度神经网络(PSO-DNN)和深度神经网络(DNN)对层状地电模型进行反演测试。设置参数如下:DNN为6层全链接方式(包含输入、输出及隐含层),其中隐含层4层,节点数随训练数据集改变;粒子群规模20,进化次数200,学习因子c1=c2=2.0,PSO-DNN算法惯性权值取0.8,IPSO-DNN算法惯性权值见式(15)。
3.1 2层地电断面模型
将正演得到的d值的实分量等距取20个频点作为网络的输入,则输入层节点数为20;将2层地电断面模型的电阻率及厚度作为网络的输出,则输出层节点(
,
,
)个数为3。为减少计算量,将电阻率与厚度值固定在一定范围,
和
取值范围为100~1 000
,
的取值范围为100~1 000 m,每个参数等距选择10个值,即
与
为100,200,…,1 000
,
为100,200,…,1 000 m。则模型总量为1 000组,将其中800组模型作为训练样本,200组模型作为测试样本,并对数据进行归一化处理。
利用IPSO-DNN,PSO-DNN和DNN算法分别对800组已知模型进行学习训练,图5所示为训练过程中均方误差(MSE)收敛曲线。由图5可见:DNN,PSO-DNN和IPSO-DNN算法起始反演结果均方误差分别为0.102,0.090和0.079,说明改进粒子群优化算法降低了网络的初始误差;在训练过程中,IPSO-DNN算法在前20轮收敛速度极快,明显优于其余2种算法,当训练至100轮以后,PSO-DNN和DNN算法收敛速度极慢,计算已陷入局部极小,而IPSO-DNN算法避开了此问题,100轮以后反演结果均方误差已小于0.010;当训练至200轮时,DNN算法的反演结果均方误差为0.038,PSO-DNN算法的反演结果均方误差为0.025,IPSO算法的反演结果均方误差为0.008,说明IPSO-DNN算法在收敛速度及精度上均高于DNN和PSO-DNN算法。
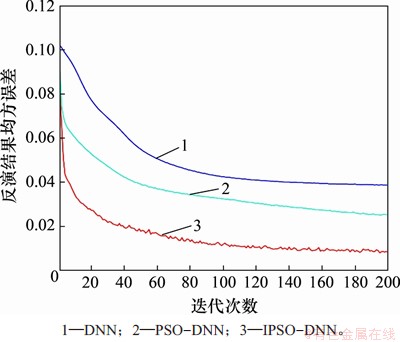
图5 不同方法训练2层模型反演结果的均方误差收敛曲线
Fig. 5 MSE convergence curve of inversion for training two-layer model with different method
对200组测试数据分别利用IPSO-DNN,PSO-DNN与DNN算法进行反演,并从中随机抽取10组数据的反演结果如表1所示,反演结果相对误差如表2所示。从表1和2可见:随机抽取的10组数据中IPSO-DNN算法反演相对误差明显低于其余2种反演方法的相对误差。为了更直观地展示反演结果,抽取一组G型与D型地电断面模型绘制电阻率-深度图,如图6所示。从图6可见:相比于PSO-DNN和DNN算法,IPSO-DNN算法反演出的电阻率和深度更接近真实模型。
表1 2层模型10组数据不同方法反演结果对比
Table 1 Comparison of inversion results of 10 sets of data for the two-layer model with different methods
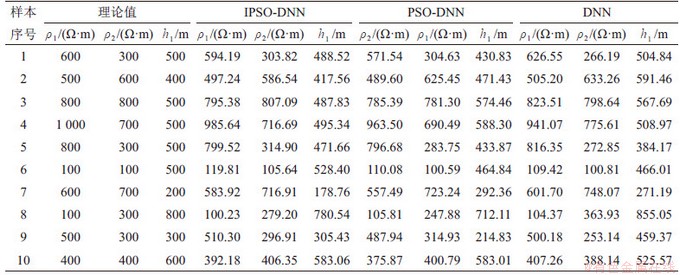
表2 2层模型10组数据不同方法反演结果相对误差对比
Table 2 Comparison of relative errors of 10 set of data for the two-layer model with different methods %
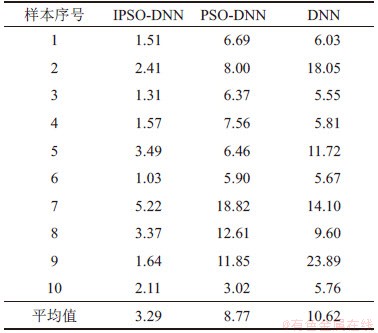
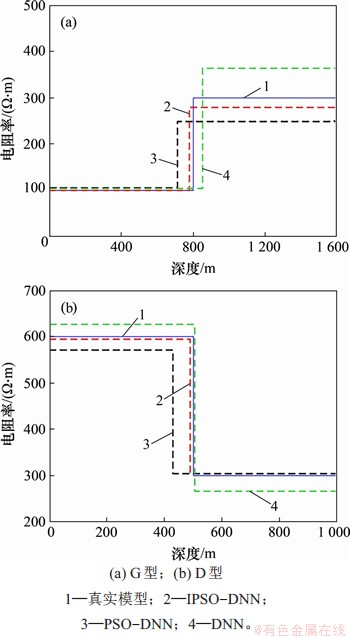
图6 2层理论模型不同方法反演结果对比
Fig. 6 Comparison of inversion results of the two-layer model with different methods
3.2 3层理论模型
采用3层水平模型进行研究,则网络输出层节点(
,
,
,
,
)个数为5。令
,
和
的取值范围100~1 000 Ω·m,
和
的取值范围为100~1 000 m,每个参数等间隔取10个值,则模型总量为10×104。将其中80%(即8×104组)数据模型作为训练样本,其余20%(即2×104组)数据模型作为测试样本。利用IPSO-DNN,PSO-DNN和DNN算法分别对8×104组已知模型进行学习训练,图7所示为训练过程中均方误差(MSE)收敛曲线。由图7可见:DNN,PSO-DNN和IPSO-DNN算法起始均方误差分别为0.093,0.060和0.040,IPSO-DNN算法降低了网络的起始误差;在训练过程中,IPSO-DNN算法前10轮均方误差就已下降到较低值,相比之下,DNN和PSO-DNN算法收敛速度较慢;当训练至200轮时,IPSO算法的均方误差为0.016,PSO-DNN算法的均方误差为0.024,DNN算法的均方误差为0.029,说明在3层地电断面模型中,IPSO-DNN算法的收敛速度及精度也高于其余2种算法。
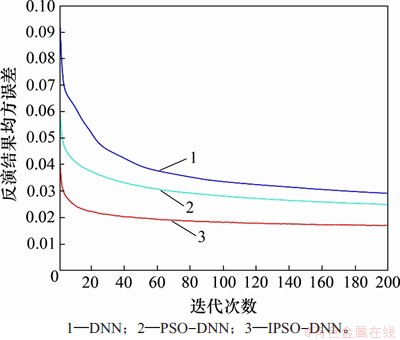
图7 不同方法训练3层模型反演结果的均方误差收敛曲线
Fig. 7 MSE convergence curve of inversion results for training three-layer model with different methods
对2×104组测试数据分别利用IPSO-DNN,PSO-DNN和DNN算法进行反演,并从中抽取10组数据的IPSO-DNN反演结果如表3所示,相对误差如表4所示。由表3和4可知:IPSO-DNN算法的反演结果与理论模型的平均相对误差仅为4.53%,而PSO-DNN和DNN的平均相对误差均超过了10%。从表3中分别抽取A,H,K和Q型地电断面模型绘制电阻率-深度图,如图8所示,IPSO-DNN算法的反演结果能更准确地接近真实模型。
表3 3层模型10组数据IPSO-DNN反演结果
Table 3 IPSO-DNN inversion results of 10 sets of data for the three-layer model
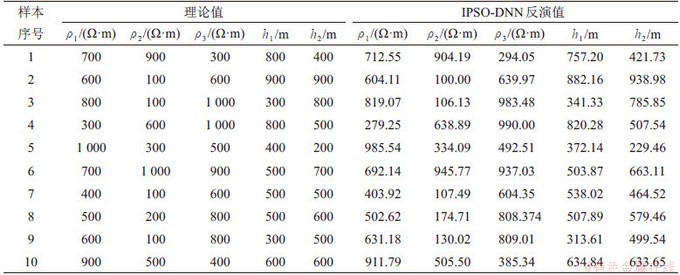
表4 3层模型10组数据不同方法反演结果相对误差对比
Table 4 Comparison of relative errors of inversion results of 10 set of data for the three-layer model with different methods %
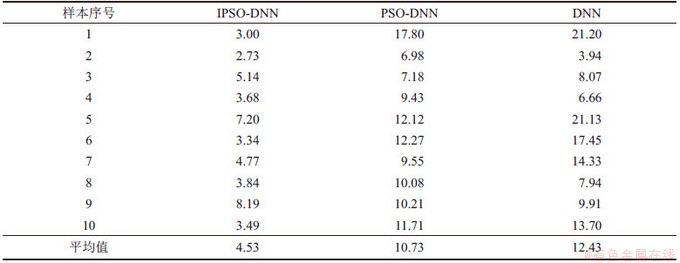
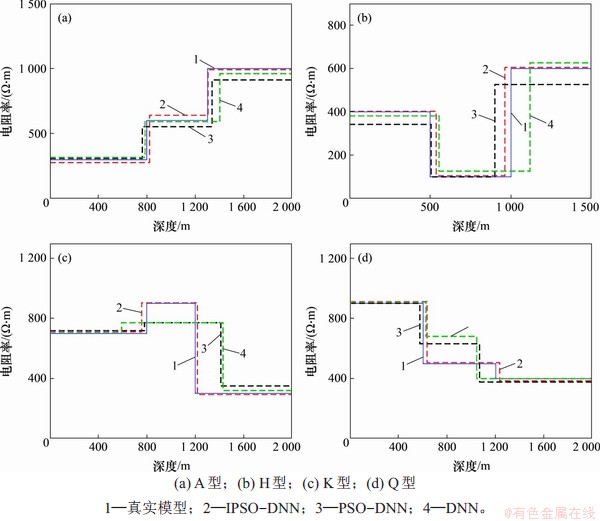
图8 3层理论模型不同方法反演结果对比
Fig. 8 Comparison of inversion results of the three-layer model with different methods
3.3 不同程度干扰下的反演结果对比
为测试该算法在加入干扰后的适用性,本文在正演得到的测试集数据中加入了均值和方差均为零的正态分布随机噪声,含噪等级分别为3%,5%和10%。分别利用IPSO-DNN,PSO-DNN和DNN算法对3种不同程度噪声水平的数据进行反演,并从中随机抽取了15组反演结果,计算出与理论数据平均相对误差,如表5所示,由表5可见:在2层及3层模型的含噪数据反演结果中,不论噪声程度如何,IPSO-DNN算法反演结果相对误差均低于PSO-DNN和DNN算法;此外,噪声等级3%对各个方法反演效果的影响并不大。但随着噪声等级增加至5%和10%,PSO-DNN和DNN反演结果已出现较大误差,IPSO-DNN反演误差虽然也有增大,但误差增加幅度比PSO-DNN和DNN反演算法的小,表明IPSO-DNN算法对于含噪数据具有更高的反演精度。
4 实测数据反演
利用IPSO-DNN方法对某地区近地表的实测航空电磁数据进行反演,验证该方法的有效性。实测数据为HCP装置测量的垂直磁场分量,共观测5个频点,分别为386,1 538,6 257,25 790和100 264 Hz。根据该地区已知的地质资料及岩矿物性测定结果,确定了适合本地区的IPSO-DNN算法参数取值范围,并进行学习训练;再利用训练好的参数对实测数据进行反演,与Occam反演结果进行对比。
表5 不同程度干扰下各方法反演相对误差对比
Table 5 Comparison of relative error inversion of various methods under different degrees of interference %
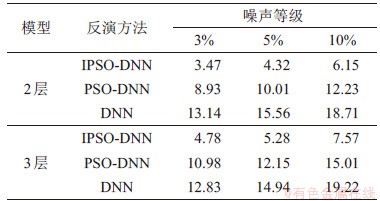
图9所示为航空电磁实测数据Occam反演结果。由图9可知剖面地层的连续性较好,地下电性结果大体分为3层:第1层电阻率为8~18 Ω·m,厚度约为10 m,经实际勘查确定为砂泥层;第2层电阻率为3~8 Ω·m,厚度约为10 m,确定为黏土层;第3层电阻率为8~18 Ω·m,确定为含砂质地层。
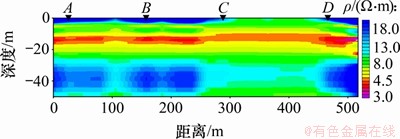
图9 航空电磁实测数据Occam反演结果(据殷长春等[20])
Fig. 9 Inversion results of survey data with Occam’s method
图10所示为4个测点的IPSO-DNN与Occam反演结果对比图,图10中的虚线为测点A,B,C和D(距离分别为25.7,157.7,288.2和466.1 m)进行IPSO-DNN反演的结果,实线为Occam反演结果。由图10可见:2种方法反演结果变化趋势基本吻合,IPSO-DNN算法的有效性得以验证。图10(d)中测点D的第1和3层反演电阻率与Occam反演结果有一定差异,说明此方法的预测结果与实际工区结果仍存在误差,需要考虑更多实际影响因素。
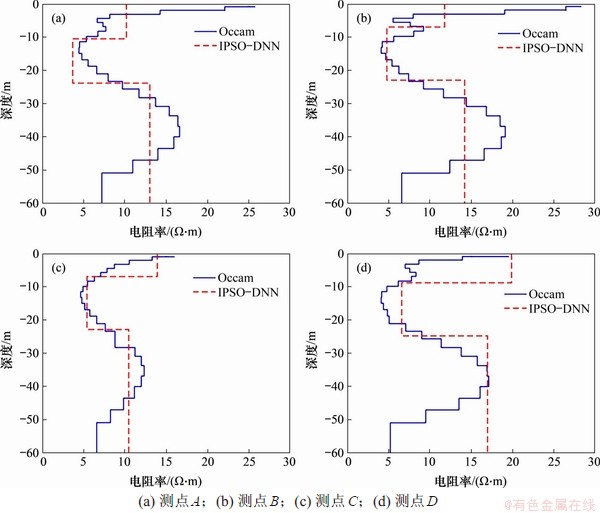
图10 航空实测数据IPSO-DNN与Occam反演结果对比图
Fig. 10 Comparison of inversion results of IPSO-DNN and Occam data from survey data
5 结论
1) 将深度神经网络与改进粒子群优化算法结合,提出了改进粒子群神经网络反演方法,将该方法运用于频率域航空电磁反演,理论和实际结果均证明该方法的可行性。
2) 改进粒子群深度神经网络算法结合了改进粒子群优化算法的良好的全局寻优性能与深度神经网络出色的局部寻优能力,有效避免了神经网络训练学习训练陷入局部极小等问题。相比于粒子群神经网络和单一神经网络,该方法具有更高精度与收敛速度;此外,改进粒子群深度神经网络算法对含干扰数据的反演效果也优于粒子群深度神经网络算法和单一的深度神经网络算法。
3) 对于实测数据,受计算量的影响,需要了解测区相关地质资料以确定网络参数控制范围;为了减小实际资料反演误差,应考虑更多实际影响因素,建立符合实际地质情况的数据集。
参考文献:
[1] 雷栋, 胡祥云, 张素芳. 航空电磁法的发展现状[J]. 地质找矿论丛, 2006, 21(1): 40-44, 53.
LEI Dong, HU Xiangyun, ZHANG Sufang. Development status quo of airborne electromagnetic[J]. Contributions to Geology and Mineral Resources Research, 2006, 21(1): 40-44, 53.
[2] SENGPIEL K P. Approximate inversion of airborne em data from a multilayered ground[J]. Geophysical Prospecting, 1988, 36(4): 446-459.
[3] HUANG Haoping, FRASER D C. The differential parameter method for multifrequency airborne resistivity mapping[J]. Geophysics, 1996, 61(1): 100-109.
[4] FARQUHARSON C G, OLDENBURG D W, ROUTH P S. Simultaneous 1D inversion of loop-loop electromagnetic data for magnetic susceptibility and electrical conductivity[J]. Geophysics, 2003, 68(6): 1857-1869.
[5] HUANG Haoping, FRASER D C. Inversion of helicopter electromagnetic data to a magnetic conductive layered earth[J]. Geophysics, 2003, 68(4): 1211-1223.
[6] 周道卿, 谭林, 谭捍东, 等. 频率域吊舱式直升机航空电磁资料的马奎特反演[J]. 地球物理学报, 2010, 53(2): 421-427.
ZHOU Daoqing, TAN Lin, TAN Handong, et al. Inversion of frequency domain helicopter-borne electromagnetic data with Marquardt's method[J]. Chinese Journal of Geophysics, 2010, 53(2): 421-427.
[7] AUKEN E, CHRISTIANSEN A V, JACOBSEN B H, et al. Piecewise 1D laterally constrained inversion of resistivity data[J]. Geophysical Prospecting, 2005, 53(4): 497-506.
[8] VALLEE M A, SMITH R S. Inversion of airborne time-domain electromagnetic data to a 1D structure using lateral constraints[J]. Near Surface Geophysics, 2009, 7(1): 63-71.
[9] 蔡晶, 齐彦福, 殷长春. 频率域航空电磁数据的加权横向约束反演[J]. 地球物理学报, 2014, 57(3): 953-960.
CAI Jing, QI Yanfu, YIN Changchun. Weighted Laterally-constrained inversion of frequency-domain airborne EM data[J]. Chinese Journal of Geophysics, 2014, 57(3): 953-960.
[10] BRODIE R, SAMBRIDGE M. A holistic approach to inversion of frequency-domain airborne EM data[J]. Geophysics, 2006, 71(6): G301-G312.
[11] BRODIE R, SAMBRIDGE M. Holistic inversion of frequency-domain airborne electromagnetic data with minimal prior information[J]. Exploration Geophysics, 2009, 40(1): 8-16.
[12] 朱凯光, 林君, 韩悦慧, 等. 基于神经网络的时间域直升机电磁数据电导率深度成像[J]. 地球物理学报, 2010, 53(3): 743-750.
ZHU Kaiguang, LIN Jun, HAN Yuehui, et al. Research on conductivity depth imaging of time domain helicopter-borne electromagnetic data based on neural network[J]. Chinese Journal of Geophysics, 2010, 53(3): 743-750.
[13] 朱凯光, 马铭遥, 车宏伟, 等. 基于主成分的时间域航空电磁数据神经网络反演仿真研究[J]. 应用地球物理, 2012, 9(1): 1-8, 114.
ZHU Kaiguang, MA Mingyao, CHE Hongwei, et al. PC-based artificial neural network inversion for airborne time-domain electromagnetic data[J]. Applied Geophysics, 2012, 9(1): 1-8, 114.
[14] 刘力辉, 陆蓉, 杨文魁. 基于深度学习的地震岩相反演方法[J]. 石油物探, 2019, 58(1): 123-129.
LIU Lihui, LU Rong, YANG Wenkui. Seismic lithofacies inversion based on deep learning[J]. Geophysical Prospecting for Petroleum, 2019, 58(1): 123-129.
[15] 奚先, 黄江清. 散射波场的深度学习反演成像法[J]. 地球物理学进展, 2018, 33(6): 2483-2489.
XI Xian, HUANG Jiangqing. Deep learning inversion imaging method for scattered wavefield[J]. Progress in Geophysics, 2018, 33(6): 2483-2489.
[16] 师学明, 肖敏, 范建柯, 等. 大地电磁阻尼粒子群优化反演法研究[J]. 地球物理学报, 2009, 52(4): 1114-1120.
SHI Xueming, XIAO Min, FAN Jianke, et al. The damped PSO algorithm and its application for magnetotelluric sounding data inversion[J]. Chinese Journal of Geophysics, 2009, 52(4): 1114-1120.
[17] 柳建新, 童孝忠, 杨晓弘, 等. 实数编码遗传算法在大地电磁测深二维反演中的应用[J]. 地球物理学进展, 2008, 23(6): 1936-1942.
LIU Jianxin, TONG Xiaozhong, YANG Xiaohong, et al. Application of real coded genetic algorithm in two-dimensional magnetotelluric inversion[J]. Progress in Geophysics, 2008, 23(6): 1936-1942.
[18] 胡祖志, 陈英, 何展翔, 等. 大地电磁并行模拟退火约束反演及应用[J]. 石油地球物理勘探, 2010, 45(4): 597-601.
HU Zuzhi, CHEN Ying, HE Zhanxiang, et al. MT parallel simulated annealing constrained inversion and its application[J]. Oil Geophysical Prospecting, 2010, 45(4): 597-601.
[19] 胡祖志, 何展翔, 杨文采, 等. 大地电磁的人工鱼群最优化约束反演[J]. 地球物理学报, 2015, 58(7): 2578-2587.
HU Zuzhi, HE Zhanxiang, YANG Wencai, et al. Constrained inversion of magnetotelluric data with the artificial fish swarm optimization method[J]. Chinese Journal of Geophysics, 2015, 58(7): 2578-2587.
[20] 殷长春, 齐彦福, 刘云鹤, 等. 频率域航空电磁数据变维数贝叶斯反演研究[J]. 地球物理学报, 2014, 57(9): 2971-2980.
YIN Changchun, QI Yanfu, LIU Yunhe, et al. Trans-dimensional Bayesian inversion of frequency-domain airborne EM data[J]. Chinese Journal of Geophysics, 2014, 57(9): 2971-2980.
[21] 高明亮, 于生宝, 郑建波, 等. 基于IGA算法的电阻率神经网络反演成像研究[J]. 地球物理学报, 2016, 59(11): 4372-4382.
GAO Mingliang, YU Shengbao, ZHENG Jianbo, et al. Research of resistivity imaging using neural network based on immune genetic algorithm[J]. Chinese Journal of Geophysics, 2016, 59(11): 4372-4382.
[22] 陈东敬, 张新兵. 带模拟退火的拟BP神经网络在伊朗某地区重力资料反演中的应用[J]. 勘探地球物理进展, 2005, 28(3): 215-218.
CHEN Dongjing, ZHANG Xinbing. Application of SA-BPNN in inversion of gravity data from Iran[J]. Progress in Exploration Geophysics[J], 2005, 28(3): 215-218.
[23] 戴前伟, 江沸菠. 基于混沌振荡PSO-BP算法的电阻率层析成像非线性反演[J]. 中国有色金属学报, 2013, 23(10): 2897-2904.
DAI Qianwei, JIANG Feibo. Nonlinear inversion for electrical resistivity tomography based on chaotic oscillation PSO-BP algorithm[J]. The Chinese Journal of Nonferrous Metals, 2013 23(10): 2897-2904.
[24] 王鹤, 刘梦琳, 席振铢, 等. 基于遗传神经网络的大地电磁反演[J]. 地球物理学报, 2018, 61(04): 1563-1575.
WANG He, LIU Menglin, XI Zhenzhu, et al. Magnetotelluric inversion based on BP neural network optimized by genetic algorithm[J]. Chinese Journal of Geophysics, 61(4): 1563-1575.
[25] 纳比吉安. 勘查地球物理电磁法[M]. 赵经详, 译. 北京: 地质出版社, 1992: 195-200.
NABIGHIAN M N. Electromagnetic method in applied geophysics(in Chinese)[M]. Zhao Jinxiang, Tran. Beijing: Geological Publishing House, 1992: 195-200.
[26] 朴化荣, 殷长春. 频率测深正演问题滤波算法及人机反演[J]. 物化探计算技术, 1987, 9(2): 137-149.
PIAO Huarong, YIN Changchun, Calculation of EM sounding by filtering method and an interactive inversion system[J]. Computing Techniques for Geophysical and Geochemical Exploration, 1987, 9(2): 137-149.
[27] LECUN Y, BENGIO Y, HINTON G. Deep learning[J]. Nature, 2015, 521(7553): 436-444.
[28] KINGMA D P, BA J. Adam: a method for stochastic optimization[EB/OL]. 2014: arXiv:1412.6980[cs.LG]. https:// arxiv.org/abs/1412.6980
[29] KENNEDY J, EBERHART R. Particle swarm optimization[C]// Proceedings of ICNN'95-International Conference on Neural Networks, Perth, WA, Australia. IEEE, 1995, 1942-1948.
[30] EBERHART R, KENNEDY J. A new optimizer using particle swarm theory[C]// MHS'95. Proceedings of the Sixth International Symposium on Micro Machine and Human Science, Nagoya. Japan. IEEE, 1995, 39-43.
[31] TAN Yue, TAN Guanzheng, DENG Shuguang. Hybrid particle swarm optimization with chaotic search for solving integer and mixed integer programming problems[J]. Journal of Central South University, 2014, 21(7): 2731-2742.
[32] 邵洪涛, 秦亮曦, 何莹. 带变异算子的非线性惯性权重PSO算法[J]. 计算机技术与发展, 2012, 22(8): 30-33, 38.
SHAO Hongtao, QIN Liangxi, HE Ying. A nonlinear inertia weight particle swarm optimization algorithm with mutation operator[J]. Computer Technology and Development, 2012, 22(8): 30-33, 38.
[33] TANG Yang, WANG Zidong, FANG Jianan. Feedback learning particle swarm optimization[J]. Applied Soft Computing, 2011, 11(8): 4713-4725.
(编辑 秦明阳)
收稿日期: 2020 -01 -07; 修回日期: 2020 -05 -09
基金项目(Foundation item):四川省科技厅研发项目(2019YFG0460,2019YFG0001,2017JY0264);国家自然科学基金资助项目(41672295) (Projects(2019YFG0460, 2019YFG0001, 2017JY0264) supported by the Research and Development Programs of Science and Technology Department of Sichuan Prorince; Project(41672295) supported by the National Natural Science Foundation of China)
通信作者:张志厚,博士,讲师,从事电磁正反演与深度学习算法研究;E-mail:logicprimer@163.com