对传神经网络算法的改进及其应用
宋晓华,李彦斌,韩金山,牛 达
(华北电力大学 工商管理学院, 北京 102206)
摘 要:针对传统对传神经网络(Counter propagation networks,即CPN)要求输入向量必须均匀分布以及隐含层神经元个数难以确定,其应用受到很大局限等问题,对CPN算法进行改进并运用于电力负荷预测。研究结果表明:通过改进CPN算法的初始权重设置规则,克服了对输入向量限制过于严格的不足;通过优化算法运行步骤,提高了算法的运行效果;改进后的CPN算法比BP算法所得预测结果误差小,比目前电力负荷预测研究中RBF和Elman神经网络所得预测结果误差也小;与BP算法相比,CPN改进算法的预测精度提高4%左右,运算时间减少45%,适用于电力负荷的预测。
关键词:负荷预测;人工神经网络;BP神经网络;对传神经网络
中图分类号:TP39 文献标识码:A 文章编号:1672-7207(2008)05-1059-05
An improved counter propagation networks and its application
SONG Xiao-hua, LI Yan-bin, HAN Jin-shan, NIU Da
(School of Business Administration, North China Electric Power University, Beijing 102206, China)
Abstract: Based on the fact that the input vectors of counter propagation networks(CPN) are supposed to be uniform distribution,,and it is difficult to choose the number of their hidden lay neurons, its application is restricted in a few fields, CPN algorithm was improved and was applied to power load forecasting. The results show that through changing the setting rules of the initiation weight, the problem of too strict limitation to input vectors can be solved. Based on the optimization of the operation process, the efficient of the CPN can be enhanced. The simulation error using the ameliorated CPN is lower than those with BP, RBF and Elman networks. Compared to traditional BP networks, the forecasting accuracy using the ameliorate ameliorated PN improves about 4%, and the computing time reduces 45%. The improved CPN can be used to forecast the power load.
Key words: load forecasting; artificial neural networks; BP Networks; counter propagation networks
电力系统负荷预测按时间可以分为长期负荷预测、中期负荷预测和短期负荷预测。短期负荷预测在电网运行实时控制和发电规划中具有重要地位,提高负荷预测精度对于合理安排电网运行方式、制定机组检修和进行电力需求管理意义重大[1]。短期负荷既呈现周期性变化的规律,又受天气、节假日等特殊情况的影响,同时,还存在由未知的不确定因素引起的随机波动呈现出复杂的非线性特性。神经网络具有较强的非线性映射能力和学习能力等优点,近年来被广泛应用于短期负荷预测中,如反向传播神经网(BP),径向基函数(RBF)网络,反馈性神经网Elman,支持向量机等等在电力负荷预测中都得到广泛应用[2-10]。由于目前神经网络还没有很完善的学习方法,经验参数太多,故一种神经网络只能解决部分特定问题,并没有哪一种网络能够很好地解决预测中遇到的多种问题。在此,本文作者将对传神经网络(Counter propagation networks,简称CPN)这种集自组织和一种基本竞争型网络于一体的神经网络引入短期负荷预测实践中,对其算法加以改进用于负荷预测。
1 CPN算法
1.1 CPN网络
对传神经网络也叫反传网,其网络拓扑结构与BP神经网络类似,都由2层神经网络组成(不需把输入层当成严格意义上的单独一层,因为输入层不执行任何算法,其作用仅仅是将输入向量输送到算法执行的第1层)。但与BP神经网络不同的是,CPN是一个异构网,2层神经网络执行不同的训练算法,网络的异构性更接近于对人脑功能的模拟。对传神经网络第1层执行Kohonen提出的自组织映射(Self-organization map,简称为SOM)算法,被称为Kohonen层。该层执行无导师学习,以“强者占先,弱者退出”方式工作,网络按照SOM学习规则产生竞争层的获胜神经元,并按这一规则调整相应的Kohonen层的连接权值W。第2层为输出层,执行Grossberg提出的散射星(Outstar)算法,被称为Grossberg层。Grossberg层执行有导师训练,按照基本竞争型网络学习规则,得到各输出神经元的实际输出,并按照有导师型的误差校正方法,修正Grossberg层的连接权值V,以实现类的表示功能。
CPN将Kohonen特征映射网络与Grossberg基本竞争型网络相结合,充分发挥了它们各自的特长:无导师训练解决网络隐含层的理想输出未知问题,有导师训练解决输出层按系统要求给出指定输出结果的问题。经过反复学习,CPN可以将任意输入模式映射为输出模式[2-3]。CPN网络拓扑结构如图1所示[3, 5-6]。
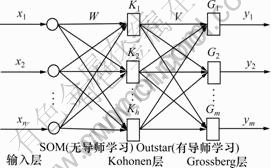
图1 CPN结构图
Fig.1 Architecture of CPN
1.2 CPN网络算法实现步骤
a. 数据预处理:将输入量Pk进行归一化处理。
b. 对CPN网络进行训练。它包括以下几种。
1) Kohonen层训练。即对Kohonen层的连接权wij初始化,将连接权赋予[0,1]内的随机值;然后,计算Kohonen层神经元的输入,获得最大输入的神经元输出为1,其余竞争层神经元的输出为0,并按照如下公式调整连接权向量:
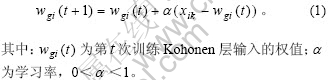
2) Grossberg层训练。对Grossberg层的连接权值V初始化,将连接权向量赋予[0,1]内的随机值;修正竞争层到输出层的连接权值Vl为:

求输出层各神经元的加权输入,并将其作为输出神经元的实际输出值。
3) 重复训练过程。重复以上2步,然后,进行下一个输入模式训练,直到达到最大训练次数或者网络误差E小于预定误差为止。

c. 利用已训练的网络进行预测。
2 CPN常规算法改进
在CPN常规算法中,将Kohonen层取均匀分布的随机数作为层连接权向量,这实际上假设输入向量均匀分布。这个条件在多数情况下不成立,故Kohonen层部分神经元可能得不到激活,受输入连接权值的随机性影响,网络稳定性无法保证。这是CPN网络应用面有限的一个主要原因。此外,CPN常规算法实现步骤复杂,运行效率较低。本文针对CPN网络的上述不足提出改进方案。
2.1 改进CPN算法Kohonen层初始权设置
首先,直接设置Kohonen层的初始权向量,然后,改造输入向量,使网络在开始训练时对问题进行粗略分类,并从这个分类中提取一个较具代表性的量构成样本集,尽可能激活Kohonen层全部神经元。其具体过程是:在Kohonen层训练步骤中将wij初始化改为取wij=1/N1/2,使输入连接权值服从均匀分布,网络输入向量为
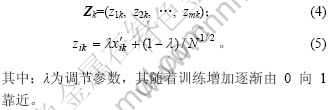
这样,Zk开始时近似于均匀分布的输入连接权值wij,随着训练逐步逼近
,在训练过程中,Wj趋近于某一组Xk的平均值[14-15]。
2.2 改进CPN算法运行模式
采用改进算法运行模式可提高其运算速度。将CPN网络学习分2个阶段进行:第1阶段只对输入层至竞争层的网络连接权值进行调整,当网络在这一阶段进行充分学习之后,才转入第2阶段的学习;第2 阶段只对竞争层至输出层的网络连接权值进行调整。最后,通过动态调整2个不同学习阶段的学习率
和
,使其随着训练次数的增加而递减,既加快网络前期的学习速度,又在网络训练的后期阶段使连接权的调整更加精确。如设

3 算例分析
以东北某地区2007-07-23日到2007-08-03每隔2 h时所测的电力负荷数据为例,分别使用BP算法、CPN算法和CPN改进算法进行预测,并将CPN改进算法预测结果与BP,RB和Elman网络预测结果进行比较,结果如表1所示。网络输入变量分为4部分:周期性因素、气温、天气和日类型。
表1 负荷预测变量
Table 1 Variables for forecasting model
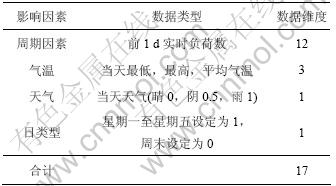
算例取2007-07-24负荷到2007-08-02当天影响因素以及相对应的前1 d的实时负荷作为训练的输入数据,以当天的负荷作为训练目标输出向量,共10组训练数据;以2007-08-03当天影响因素和2007-08-02负荷作为测试的输入数据,以2007-08-03的实际负荷作为测试的目标输出数据,用于检测网络的预测能力。
在本例中负荷数据首先采用归一化公式处理。输入量负荷归一化公式为:
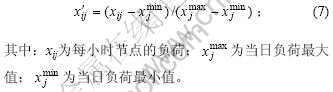
气温数据归一化公式为:

天气和工作日类型是根据定性变量转化成的[0,1]间的数值,不需要进行处理。
分别采用BP算法、CPN算法和CPN改进算法进行预测。这3种算法的隐含层神经元数目分别取17~36对网络进行训练比较。训练误差函数采用均方误差函数,学习速率均采用变动学习率,总训练次数均为500。BP算法由于不太稳定,选取保存的几次训练中综合误差最小的初始权值。表2所示为BP算法、CPN常规算法和CPN改进算法预测的比较结果。其中,测试误差采用平均相对误差:
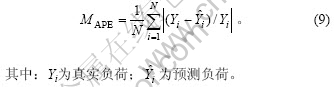
表2 采用BP算法、CPN常规算法与CPN改进算法的MAPE对比
Table 2 Contrast between BP, CPN and enhanced CPN %
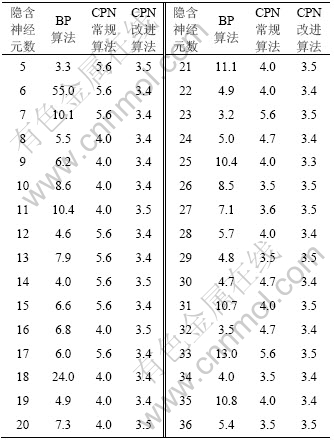
从表2可以看出:BP算法预测结果不稳定,随着隐含层神经元个数增多而波动较大;CPN算法预测结果稍有波动,改进后的CPN算法预测结果波动较小,最大相对误差仅为0.1%,且其预测精度明显提高,测试误差MAPE仅为3.4%左右,比绝大部分BP网络预测误差(8.9%)和常规CPN预测误差(4.5%)的小。CPN改进算法精度相当稳定,不随着隐含层神经元数目变化而变化。为了进一步与其他神经网络算法进行对比,选取RBF和Elman网络进行测试,预测结果见表3和图2。
表3 BP算法、RBF算法、Elman算法和CPN改进算法网络预测结果相对误差对比
Table 3 Comparison of relative errors using BP, RBF, Elman and improved CPN algorithms %
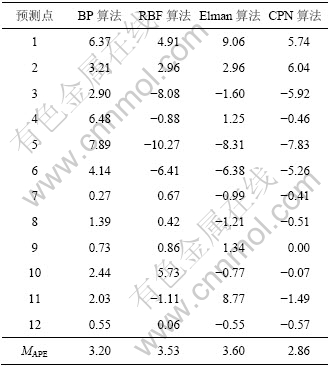
从表3和图2可以看出,虽然这4种神经网络在不同测试点的误差各不相同,但是,总体来说,CPN改进算法相对误差较小,其平均相对误差在4种神经网络中最小,只有2.86%。实例表明,CPN改进算法对于该类型负荷预测具有很强的泛化能力。考虑到本例训练样本的有限性,该精度完全满足要求。
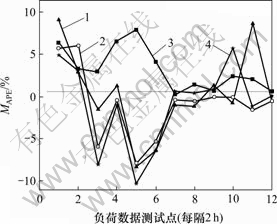
1—Elman算法; 2—CPN算法; 3—BP算法; 4—RBF算法
图2 不同算法所得预测误差
Fig.2 Forecasting errors with different algorithms
表4所示为4种算法所需计算时间的对比结果。可以看出,CPN改进算法较BP算法的计算时间减少45%,因而其具有更高的学习效率。
表4 不同算法的计算时间比较
Table 4 Comparison of computing time between BP, RBF, Elman and improved CPN algorithms
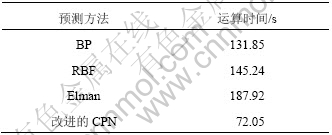
4 结 论
a. 通过优化CPN网络的初始权设置规则,有效地克服了对于输入向量限制过于严格的局限,从而避免了一些原有的缺陷,如:初始权值和样品输入顺序对网络的学习影响较大。改进后的CPN网络性能更加稳定,适用范围扩大。
b. 通过优化CPN算法的运行步骤,提高了算法的计算速度。CPN改进算法预测精度较高,网络收敛速度快,其实际训练时间与BP算法的相比减少45%;同时,CPN改进算法比RBF和Elman的预测精度高,非常适用于电力系统的短期负荷预测。
c. 为了进一步提高CPN改进算法的运算效果,可以引入一些优化算法,对隐层神经元数量进行优化。此外,对于电力负荷预测问题本身的建模有待进一步加强。
参考文献:
[1] 刘 玲, 严登俊, 龚灯才, 等. 基于粒子群模糊神经网络的短期电力负荷预测[J]. 电力系统及其自动化学报, 2006, 18(3): 47-50.
LIU Lin, YAN Deng-jun, GONG Deng-cai, et al. New method for short term load forecasting based on particle swarm optimization and fuzzy neural network[J]. Proceedings of the Chinese Society of Universities, 2006, 18(3): 47-50.
[2] 张乃尧, 阎平凡. 神经网络与模糊控制[M]. 北京: 清华大学出版社, 1998.
ZHANG Nai-yao, YAN Ping-fan. Neural networks and fuzzy control[M]. Beijing: Tsinghua University Press, 1998.
[3] 阎平凡, 张长水. 人工神经网络与模拟进化计算[M]. 北京: 清华大学出版社, 2006.
YAN Ping-fan, ZHANG Chang-shui. Artificial neural networks and evolutionary computing[M]. Beijing: Tsinghua University Press, 2006.
[4] Adeli H, Park H S. CPN in engineering[J]. Journal of Structural Engineering, 1995, 121(8): 1205-1212.
[5] 戴彦群, 王茂芝. 基于改进CPN的快速矢量量化[J]. 计算机应用, 2004, 24(5): 64-67.
DAI Yan-qun, WANG Mao-zhi. Fast vector quantization based on a modified counterpropagation network[J]. Journal of Computer Applications, 2004, 24(5): 64-67.
[6] 牛丽红, 倪国强, 苏秉华. 改进的对向传播网络及其在多传感器目标识别中的应用[J]. 光子学报, 2003, 32(2): 244-247.
NIU Li-hong, NI Guo-qiang, SU Bing-hua. Modified counter propagation network and its application to multi-sensor target recognition[J]. Acta Photonica Sinica, 2003, 32(2): 244-247.
[7] Weich P. Forecasting regional electricity load based on recurrent support vector machines with genetic algorithms[J]. Electric Power Systems Research, 2005, 74(3): 417-425.
[8] 康重庆, 夏 清, 张伯明. 电力系统负荷预测研究综述与发展方向的探讨[J]. 电力系统自动化, 2004, 27(18): 1-11.
KANG Chong-qing, XIA Qing, ZHANG Bo-ming. Review of power system load forecasting and its development[J]. Automation of Electric Power Systems, 2004, 27(18): 1-11.
[9] 朱 晟, 蒋传文, 侯志俭. 基于气象负荷因子的Elman神经网络短期负荷预测[J]. 电力系统及其自动化学报[J]. 2005, 17(1): 23-26.
ZHU Sheng, JIANG Chuan-wen, HOU Zhi-jian. Application of a weather component based Elman neural network to short-term load forecasting[J]. Proceedings of the Chinese Society of Universities, 2005, 17(1): 23-26.
[10] 李媛媛, 牛东晓. 基于最优可信度的月度负荷综合最优灰色神经网络预测模型[J]. 电网技术, 2005, 29(5): 16-19.
LI Yuan-yuan, NIU Dong-xiao. An optimum credibility based integrated optimum gray network model of monthly power load forecasting[J]. Power System Technology, 2005, 29(5): 16-19.
[11] LI Xiang, YANG Shang-dong, QI Jian-xun. A new support vector machine optimized by improved particle swarm optimization and its application[J]. Journal of Central South University of Technology, 2006, 13(5): 568-572.
[12] Yam I H Y, Shahideh P S M, Li Z. Adaptive short-term electricify price forecasting using artificial neural networks in the restructured power markets[J]. Electrical Power and Energy Systems, 2004, 26(8): 571-581.
[13] 邹政达, 孙雅明, 张智晟. 基于蚁群优化算法递归神经网络的短期负荷预测[J]. 电网技术, 2005, 29(3): 59-63.
ZHOU Zheng-da, SUN Ya-ming, ZHANG Zhi-sheng. Short-term load forecasting based on recurrent neural network using ant colony optimization algorithm[J]. Power System Technology, 2005, 29(3): 59-63.
[14] Cao L J, Tay E H. Support vector machine with adaptive parameters in financial time series forecasting[J]. IEEE Transctions on Neural Networks, 2003, 6(14): 1506-1518.
[15] PAI Ping-feng, HONG Wei-chiang. Forecasting regional electricity load based on recurrent support vector machines with genetic algorithms[J]. Electric Power Systems Research, 2005, 74(3): 417-425.
收稿日期:2008-05-10;修回日期:2008-06-29
基金项目:国家自然科学基金资助项目(70671042)
通信作者:宋晓华(1971-),女,黑龙江五常人,博士,副教授,从事计算机模拟仿真、计算机财务管理、经济技术评价与决策优化研究等;电话:010-80798623;E-mail: sxh@ncepu.edu.cn