DOI: 10.11817/j.issn.1672-7207.2019.05.014
同分布强化学习优化多决策树及其在非平衡数据集中的应用
焦江丽,张雪英,李凤莲,牛壮
(太原理工大学 信息与计算机学院,山西 太原,030024)
摘要:针对传统决策树在非平衡数据集分类时少数类预测性能出现偏差的问题,提出一种基于强化学习累积回报的属性优化策略即改进型同分布多决策树方法。首先通过同分布随机抽样法对非平衡数据集中的多数类样本进行随机采样,进而对各子集建立单决策树形成多个决策树,各决策树采用分类回归树算法建树,并利用强化学习累积回报机制进行属性选择策略的优化。研究结果表明:提出的基于强化学习累积回报机制的属性优化策略可有效提高少数类被正确分类的概率;同分布多决策树方法可有效提高非平衡数据集整体预测性能,且正类率和负类率的几何平均值都有所提高。
关键词:非平衡数据集;多决策树;累积回报机制属性选择策略;同分布随机抽样;强化学习
中图分类号:TP301 文献标志码:A 文章编号:1672-7207(2019)05-1112-07
Identically distributed multi-decision tree based on reinforcement learning and its application in imbalanced data sets
JIAO Jiangli, ZHANG Xueying, LI Fenglian, NIU Zhuang
(College of Information and Computer, Taiyuan University of Technology, Taiyuan 030024, China)
Abstract: As the general decision tree can not classify the minority class of the imbalanced data sets well, an improved identically distributed multi-decision tree approach based on reinforcement learning cumulative reward was proposed to optimize the attribute selection strategy. Firstly, the majority class samples of the imbalanced data sets were randomly sampled by the identically distributed random sampling approach, and then each single decision tree was established over each subset and eventually a multi-decision tree was formed. Each single decision tree was constructed by classification and regression tree(CART) algorithm firstly and then reinforcement learning cumulative reward mechanism was utilized to optimize the attribute selection strategy. The results show that the proposed attribute optimization strategy based on the reinforcement learning cumulative reward mechanism effectively improves the probability that the minority class can be correctly classified. The identically distributed multi-decision tree method effectively improves the overall prediction performance over imbalanced data sets. Moreover, the positive rate and geometric mean value of positive and negative rates are improved at the same time.
Key words: imbalanced data sets; multi-decision tree; cumulative reward mechanism attributes selection strategy; identically distributed random sampling; reinforcement learning
分类是数据挖掘领域的研究热点之一,目前成熟的算法主要包括朴素贝叶斯、神经网络和决策树[1]等。其中,随机森林算法因其具有简单易行、运算速度快和准确度高的特点而在交通、医学、生物学等众多领域得到了广泛应用[2-3]。然而,随着大数据时代的到 来,数据的非平衡特性也更加突出。决策树分类算法基本思想是通过一个树形结构模型,将数据集在不同分裂节点根据不同属性划分为各类别,其模型构建的核心是对分类节点的分裂属性进行最佳选择。其中比较经典的算法有ID3,C4.5和分类回归树(CART)算法等[4-5],这些算法的本质区别是节点分裂属性选择策略不同;而随机森林方法是一种将多个单决策树分类器集成的多决策树学习方法[6],在一定程度上可以解决单决策树过拟合导致的分类准确性不高的问题。上述单决策树方法及随机森林方法用于平衡数据集,一般可得到较好的分类效果,但对非平衡数据集,这些方法则存在少数类预测准确度偏低的缺陷。为此,人们提出多种改进算法。ALSHOMRANI等[7]利用特征加权法优化属性选择策略以改善非平衡数据集分类性能。在对非平衡数据集进行分类时,由于各类别的样本数目分布不均匀,少数类样本通常得不到充分训练,从而使分类器容易倾向于多数类而忽略少数类样本,但在现实生活中少数类样本通常具有更重要的作用[8-9],因此,如何提高少数类预测准确度具有重要意义。目前,国内外研究者针对非平衡数据集如何提高分类器性能开展了大量工作:LI等[10]提出了基于属性混合策略的代价敏感Top-N多决策树算法,并将其用于非平衡数据集分类预测;KOZIARSKI等[11]提出了一种用于改变非平衡数据集数据不平衡率的预处理方法;付忠良[12]提出了一种多标签代价敏感分类集成学习算法。但针对非平衡数据分类问题,虽然引入代价敏感因子和采用抽样的方法可提高分类的性能,但在实施中往往难以确定代价,且抽样的方法容易造成样本信息丢失或者过拟合等问题。强化学习方法是从自适应控制理论中发展出来的一种机器学习方法,其通过判断当前学习环境对分类器的影响,综合分析各种影响所造成的结果可对学习方法进行调整,从而使最终的分类预测偏向更加准确的结果[13-14]。周浦城等[15]将Q-Learning强化学习算法与决策树模型相结合,根据决策树当前节点输入的数据不断更新Q-表生成新的决策节点。人们对利用强化学习方法进行属性选择策略优化的研究较少,而属性选择直接影响决策树性能,为此,本文作者首先基于强化学习累积回报机制提出一种属性选择优化策略,进而将该策略与集成学习算法结合,提出一种基于同分布随机抽样的多决策树集成学习算法。
1 基于强化学习累积回报机制的优化策略
强化学习是研究一个能够感知环境的智能体,在没有先验知识的情况下,从延迟回报中学习优化的控制策略,进而使智能体的累积回报最大[16]。传统决策树采用特征选择策略建树以实现分类,其中CART算法采用基尼(Gini)指数作为特征选择策略,对非平衡数据集分类时会使多数类数据分类性能更优[17]。由于强化学习会根据智能体做出动作后的执行结果进行奖励或者惩罚,并通过延迟回报获得各个状态下的最优动作。若能借鉴这种奖惩策略,使少数类分类正确时获得更大的奖励,并将这种策略融合于决策树属性选择阶段,则有利于提高决策树中少数类分类性能。借鉴这种优化思路,本文作者提出基于累积回报机制的属性选择优化策略,通过决策树每一层的回报系数计算决策树属性选择的累积回报值以使决策树属性选择策略得到优化。
1.1 累积回报求解
依据强化学习的累积回报思想,结合CART算法,通过每一层的回报系数优化决策树的属性选择策略,本文将第1~i层的层累积回报系数相乘定义为累积回报R,其具体表达式如下:
R=R1·R2…Ri (1)
式中:Ri为该节点在决策树的第i层的累积回报系数,Ri越大,说明分类性能越优。考虑预测效果的几何平均值系数、真实正类率以及模型的准确率,本文将Ri定义为
Ri=P+i·Ai·Gmi (2)
式中:P+i为分类模型第i层的真实正类率即正确预测的正类与实际为正类的样本数量的比值,P+i越大,说明正类预测越准确,性能越好;Ai为模型第i层分类准确率即正确预测的样本数与总样本数的比值,其值越大,说明总体预测越准确,性能越好;Gmi为模型中第i层几何平均值系数即真实正类率与真实负类率乘积的开方,其值越大,说明总体预测性能越好。
(3)
式中:P-i为分类模型中第i层的真实负类率即正确预测的负类与实际为负类的样本数量的比值,其值越大,说明负类预测越准确,性能越好;NTPi为所有样本在当前节点第i层被正确的分为正类的数量;NTNi为所有样本在当前节点第i层正确分为负类的数量;NFPi为第i层错误分为正类的样本数量,NFNi为第i层错误分为负类的样本数量。
1.2 基于累积回报机制的属性选择策略
传统的属性选择策略在非平衡数据集预测中表现并不佳[18-19],为此,本文作者结合上述强化学习累积回报机制的思想,提出基于强化学习的累积回报机制的属性选择策略(cumulative reward mechanism attributes selection strategy, CRS),利用每个属性被选择后当前节点的分类结果作为影响因子反馈到当前节点属性选择的过程中,即在传统属性选择方法的基础上加入累积回报系数进行当前节点最佳分裂属性选择的优化。属性选择策略SAS定义为
(4)
式中:CGini为所有属性的Gini系数。在传统决策树属性选择中,CGini系数越小,最终分类效果越好,而累积回报系数Ri越大,说明对属性选择的优化效果越好,因此,选择SAS最小值对应的属性作为当前节点分裂的属性最好。
1.3 基于累积回报机制的决策树模型构建
本文采用自上向下递归二分技术,利用强化学习累积回报机制得到各节点的最佳分裂属性。基于累积回报机制的决策树(cumulative reward mechanism attributes selection strategy decision tree, CRDT)原理图如图1所示。
图1中CART决策树根节点采用传统的属性选择策略构建,各子节点的构建采用本文提出的累积回报机制,即根据式(4)计算各子节点的SAS,最小SAS对应的属性为子节点最佳属性,直至达到叶节点。
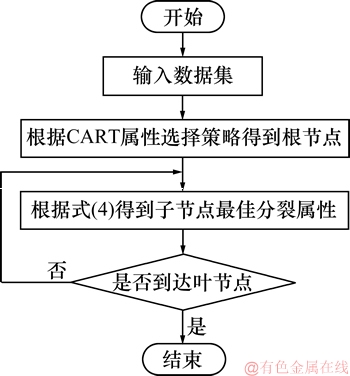
图1 CRDT原理图
Fig. 1 Schematic diagram of CRDT
2 同分布随机抽样的CRS多决策树集成学习算法
在数据集不平衡的情况下,少数类被抽取的概率很小,而经典随机森林中单决策树的训练样本是随机抽取的,因此,少数类在最后决策树形成过程中不会被充分训练[20]。已有方法是将非平衡数据集中的多数类样本随机分为T个子集,然后,分别将这些子集中的样本与少数类样本合成新的训练样本子集,通过T个决策树对各个新的样本子集分别进行训练,最后采用投票法将这些决策树结果集成。但此种方法使T个数据集之间的多数类样本完全不同,虽然少数类样本得到了充分训练,但多数类样本训练不足,因此,这种方法的分类效果并不理想。
为此,本文作者提出一种同分布随机抽样的CRS多决策树集成学习(identically distributed random sampling cumulative reward attributes selection strategy multi-decision tree, IDCRMDT)算法。该方法首先对输入数据集采用同分布随机抽样法进行预处理,得到k个样本子集,以使非平衡数据集中的多数类样本均匀分布至各子集,进而使用本文提出的累积回报属性选择策略建立各个单决策树。IDCRMDT算法原理图如图2所示。
图2中同分布随机抽样法使用K-means聚类算法对输入数据集中的多数类进行聚类,得到各决策树训练时使用的样本子集,这样既可以保证抽取的多数类样本服从同分布,又可以使少数类样本也被均匀抽样,克服了非平衡数据集的非平衡率过大时少数类样本得不到充分训练的弊端。
2.1 同分布随机抽样法
本文提出的IDCRMDT算法首先采用同分布随机抽样法对多数类样本进行聚类,形成k(选取和少数类样本一致的个数)个样本子集Dma,以使分布相近的多数类样本划分为1个集合,然后将各个子集分别与少数类样本进行组合形成新的同分布样本子集D。同分布随机抽样法流程图如图3所示。
2.2 IDCRMDT算法
数据样本集合D分为多数类样本集合Dma和少数类样本集合Dmi,记多数类样本的数量为m,少数类样本的数量为k。IDCRMDT算法流程如下。
输入:数据集D。
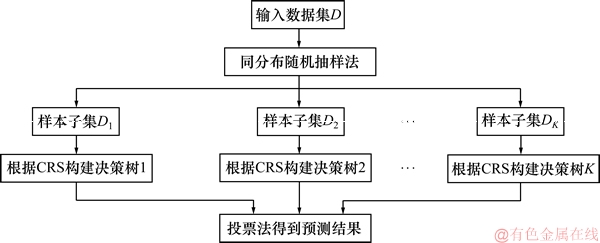
图2 IDCRMDT算法原理图
Fig. 2 Schematic diagram of IDCRMDT algorithm
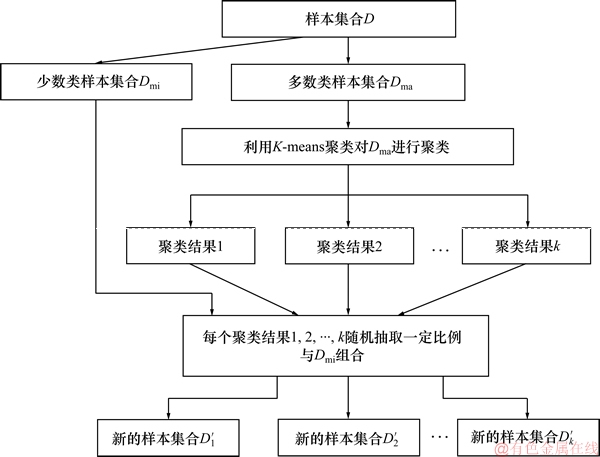
图3 同分布随机抽样法流程图
Fig. 3 Diagram of identically distributed random sampling method
输出:分类预测结果。
1) 同分布随机抽样法将数据集进行预处理,处理后数据集合变为k个子集
(j=1, 2, …, k);
2) 对各个新的数据子集
(j=1, 2, …, k)分别采用本文提出的CRS方法建立多个单决策树;
3) 使用投票法得到多决策树分类预测结果。
3 实验及结果分析
本文设计实验分别验证基于强化学习累积回报的属性选择策略的优势以及提出的IDCRMDT算法性能优劣。
3.1 实验数据
实验数据集采用KEEL-Imbalanced Data Sets中的10个不同平衡率的非平衡数据集,各数据集详情如 表1所示。
3.2 实验设计
本文设计实验方案,并采用10折交叉验证的方法得到实验结果。
1) 方案1。
对比方法:CART(单决策树);
表1 实验数据集
Table 1 Experimental data sets
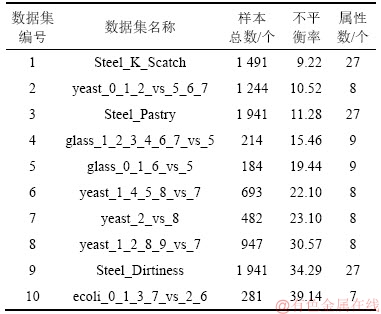
本文方法:CRDT(基于累积回报机制的单决策树)。
2) 方案2。
对比方法1:RF(传统随机森林算法);
对比方法2:CRRF(基于单决策树CRS属性选择策略的随机森林,cumulative reward mechanism attributes selection strategy random forest);
本文方法:IDCRMDT(同分布随机抽样的CRS多决策树)。
3.3 评价指标
本论文采用真实正类率P+、准确率A和几何平均值Gm评估算法的性能。其中,真实正类率P+和准确率A越大,说明决策树的分类效果更好,而几何平均值Gm是真实正类率和真实负类率乘积的开方,对于非平衡数据集而言更能反映总体预测分类性能的优劣。
3.4 实验结果
本文提出的方法CRDT与CART单决策树性能对比见图4。由图4(a)可知:相较CART,采用本文提出的CRDT算法各数据集的真实正类率P+均有所提高;对于数据集3,4,7,9和10,真实正类率分别提高16.7%,9.9%,22.0%,27.7%和60.2%。由图4(b)可以看出:除数据集1外,采用CRDT各数据集的准确率A均有所提高。由图4(c)可以看出:除了数据集1之外,采用CRDT时其余数据集几何平均值Gm都有所提高。综合来看,CRDT应用于非平衡数据集整体性能比已有的CART算法的分类性能更优。
将传统的随机森林算法(RF)与本文提出的IDCRMDT和CRRF的真实正类率、准确率以及几何平均值进行对比,结果如图5所示。从图5(a)和图5(b)可知:采用本文的IDCRMDT算法时,除数据集10的真实正类率下降较多外,其余数据集的真实正类率都比采用RF和CRRF时的真实正类率高,但准确率提高幅度不是很明显。由图5(c)可知:采用本文的IDCRMDT算法时,数据集1,2,3,5和8的Gm与采用传统随机森林算法(RF)得到的Gm相比均有所提高,而较CRRF得到的Gm则分别提高3.9%,16.9%,6.3%,7.8%和32.2%。
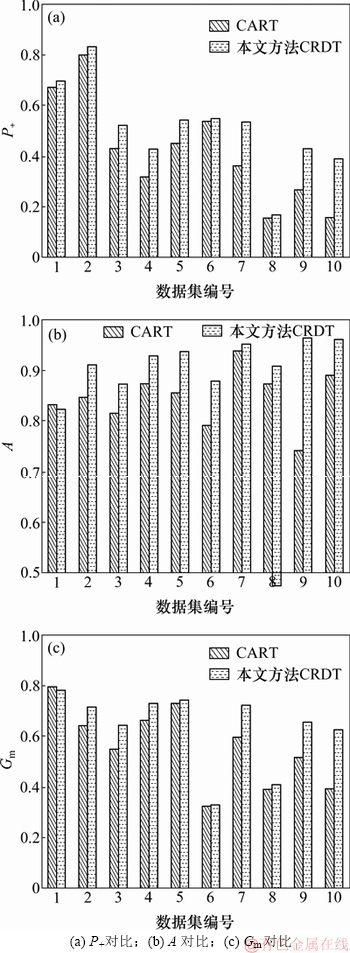
图4 CRDT与CART性能对比
Fig. 4 Performance comparison of CRDT and CART
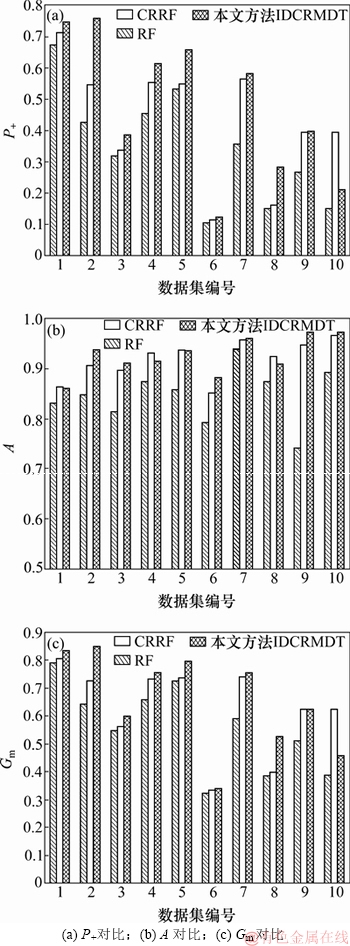
图5 IDCRMDT与CRRF和RF的性能对比
Fig. 5 Performance comparison of IDCRMDT, CRRF and RF
从实验方案1可以看出:利用强化学习累积回报机制思路改进决策树属性选择策略的性能都有所提高。随机森林算法在很大程度上解决了单决策树的过拟合问题,传统的随机森林在建树过程中采用可放回随机抽样方法产生不同的数据集,但往往非平衡数据集的少数类被抽取的概率会很小。从实验方案2可以看出:采用同分布随机抽样的方法可使少数类得到充分训练,与传统的RF以及改进的属性选择策略的CRRF算法相比,本文提出的IDCRMDT算法的真实正类率和准确率均有所提高,同时,模型整体性能有所提高。
4 结论
1) 本文提出一种同分布类随机抽样的CRS多决策树集成学习(IDCRMDT)算法,利用累积回报机制进行决策树属性选择优化,并对非平衡数据集中的多数类采用同分布随机抽样法进行预处理,以使多数类样本均匀分布于各子集并使少数类样本得到充分训练。
2) 本文提出的方法对多数非平衡数据集的分类效果均有提升;而对于个别数据集,虽然少数类分类性能得到了提高,但数据集整体预测性能稍有下降。
参考文献:
[1] HAN Jiawei, KAMBER M, PEI Jian. Data mining: concepts and techniques[M]. 3rd ed. San Francisco, USA: Elsevier, 2011: 15-23.
[2] 杨宏宇, 徐晋. 基于改进随机森林算法的Android恶意软件检测[J]. 通信学报, 2017, 38(4): 8-16.
YANG Hongyu, XU Jin. Android malware detection based on improved random forest[J]. Journal on Communications, 2017, 38(4): 8-16.
[3] 杜春蕾, 张雪英, 李凤莲. 改进的CART算法在煤层底板突水预测中的应用[J]. 工矿自动化, 2014, 40(12): 52-56.
DU Chunlei, ZHANG Xueying, LI Fenglian. Application of improved CART algorithm in prediction of water inrush from coal seam floor[J]. Industry and Mine Automation, 2014, 40(12): 52-56.
[4] STROBL C. Fifty years of classification and regression trees discussion[J]. International Statistical Review, 2014, 82(3): 349-352.
[5] 姚亚夫, 邢留涛. 决策树C4.5连续属性分割阈值算法改进及其应用[J]. 中南大学学报(自然科学版), 2011, 42(12): 3772- 3776.
YAO Yafu, Xing Liutao. Improvement of C4.5 decision tree continuous attributes segmentation threshold algorithm and its application[J]. Journal of Central South University (Science and Technology), 2011, 42(12): 3772-3776.
[6] 邓生雄, 雒江涛, 刘勇, 等. 集成随机森林的分类模型[J]. 计算机应用研究, 2015, 32(6): 1621-1624.
DENG Shengxiong, LUO Jiangtao, LIU Yong, et al. Classification model based on ensemble random forests[J]. Application Research of Computers, 2015, 32(6): 1621-1624.
[7] ALSHOMRANI S, BAWAKID A, SHIM S O, et al. A proposal for evolutionary fuzzy systems using feature weighting: dealing with overlapping in imbalanced datasets[J]. Knowledge-Based Systems, 2015, 73(1): 1-17.
[8] BEYAN C, FISHER R. Classifying imbalanced data sets using similarity based hierarchical decomposition[J]. Pattern Recognition, 2015, 48(5): 1653-1672.
[9] 陈橙, 颜艳, 何琼, 等. 决策树与logistic回归模型用于开奶时间延迟影响因素分析[J]. 中南大学学报(医学版), 2018, 43(3): 306-312.
CHEN Cheng, YAN Yan, HE Qiong, et al. Risk factors for delayed breastfeeding initiation based on decision tree model and logistic regression model[J]. Journal of Central South University (Medical Sciences), 2018, 43(3): 306-312.
[10] LI Fenglian, ZHANG Xueying, ZHANG Xiqian, et al. Cost-sensitive and hybrid-attribute measure multi-decision tree over imbalanced data sets[J]. Information Sciences, 2018, 422: 242-256.
[11] KOZIARSKI M, WO
NIAK M. CCR: A combined cleaning and resampling algorithm for imbalanced data classification[J]. International Journal of Applied Mathematics & Computer Science, 2017, 27(4): 727-736.
[12] 付忠良. 多标签代价敏感分类集成学习算法[J]. 自动化学报, 2014, 40(6): 1075-1085.
FU Zhongliang. Cost-sensitive ensemble learning algorithm for multi-label classification problems[J]. Acta Automatica Sinica, 2014, 40(6): 1075-1085.
[13] LI Hongjia, CAI Ruizhe, LIU Ning et al. Deep reinforcement learning: Algorithm, applications, and ultra-low-power implementation[J]. Nano Communication Networks, 2018, 16: 81-90.
[14] WU Jingda, HE Hongwei, PENG Jiankun, et al. Continuous reinforcement learning of energy management with deep Q network for a power split hybrid electric bus[J]. Applied Energy, 2018, 222: 799-811.
[15] 周浦城, 洪炳镕. 基于决策树的强化学习算法[J]. 计算研究与发展, 2005, 42(S): 233-237.
ZHOU Pucheng, HONG Bingrong. Decision tree based reinforcement learning algorithm[J]. Journal of Computer Research and Development, 2005, 42(S): 233-237.
[16] SUTTON R S, BARTO A G. Reinforcement learning: an introduction[M]. 2nd ed. London, UK: MIT Press, 2018: 1-68
[17] SUN Shasha, FAN Wenlai, XU Yan, et al. Comparative analysis of the aroma components of Cabernet Sauvignon in four regions [J]. Science & Technology of Food Industry, 2013, 34(24): 70-69.
[18] ALSHOMRANI S, BAWAKID A, SHIM S O, et al. A proposal for evolutionary fuzzy systems using feature weighting: Dealing with overlapping in imbalanced datasets[J]. Knowledge-Based Systems, 2015, 73(1): 1-17.
[19] ANTONELLI M, DUCANGE P, MARCELLONI F. An experimental study on evolutionary fuzzy classifiers designed for managing imbalanced datasets[J]. Neurocomputing, 2014, 146: 125-136.
[20] ARCHER K J, KIMES R V. Empirical characterization of random forest variable importance measures[J]. Computational Statistics and Data Analysis, 2008, 52(4): 2249-2260.
(编辑 伍锦花)
收稿日期:2018-06-11;修回日期:2018-08-11
基金项目(Foundation item):国家自然科学基金资助项目(61376693);山西省重点研发计划(社会发展领域)项目(201803D31045);山西省自然科学基金资助项目(201801D121138);山西省重大专项(20181102008);山西省优秀人才科技创新项目(201605D211021) (Project(61376693) supported by the National Natural Science Foundation of China; Project(201803D31045) supported by Key R&D (Social Development) Program of Shanxi Province; Project(201801D121138) supported by the Natural Science Foundation of Shanxi Province; Project(20181102008) supported by the Major Program of Shanxi Province; Project(201605D211021) supported by the Science and Technology Innovation Program for Excellent Talents in Shanxi Province)
通信作者:张雪英,博士,教授,博士生导师,从事语音信号处理以及人工智能等研究;E-mail: tyzhangxy@163.com