支持向量机及其在机械故障诊断中的应用
何学文1,2,赵海鸣1
(1.中南大学 机电工程学院,湖南 长沙,410083; 2.江西理工大学 机电工程学院,江西 赣州,341000)
摘要: 针对目前机械故障诊断中难以获得大量故障数据样本以及特征提取和诊断知识获取困难等不足,提出了应用支持向量机进行机械故障诊断的方法,研究了将小波包分析与信号能量分解用于机械故障的特征提取。该方法将振动信号小波包分析后的信号频带能量作为特征向量,输入到由多个支持向量机构成的多故障分类器中进行故障识别和分类。该分类器只需少量训练样本,而且不必预先知道故障分类的经验知识就能实现正确分类。研究结果表明:选用不同核函数及其参数的多故障分类器对分类精度有影响;在样本不带噪声和带15%噪声情况下,支持向量机的分类精度均高于BP神经网络的分类精度,具有更好的分类性能。
关键词: 支持向量机; 小波包分析; 特征提取; 故障诊断; 多故障分类器
中图分类号:TP206.3 文献标识码:A 文章编号: 1672-7207(2005)01-0097-05
Support Vector Machine and Its Application to Machinery Fault Diagnosis
HE Xue-wen1,2, ZHAO Hai-ming1
(1.School of Mechanical and Electrical Engineering, Central South University, Changsha 410083, China;
2.School of Mechanical and Electrical Engineering, Jiangxi University of Science and Technology, Ganzhou 341000, China)
Abstract: Aiming at the difficulty in getting adequate fault samples, extracting eigenvectors and acquiring diagnosis knowledge in machinery fault diagnosis, a novel method for machinery fault diagnosis based on support vector machine(SVM) was put forward, in which wavelet packet analysis and signal energy decomposition were used for the feature extraction machinery faults. According to the method, the energy of different frequency bands after wavelet packet decomposition constitutes the input vectors of support vector machine as eigenvectors, and these eigenvectors were input into multiple fault classifiers to identify faults. The new method, by which multiple faults can be diagnosed, only requires a small quantity of fault samples and it doesn’t need the field knowledge of fault diagnosis. The experimental results show that different SVM classifiers, in which different kernel functions and their parameters are adopted, will influence the precision of fault classifiers. Under the circumstances that samples don’t include noise signal and samples include 15% noise signal, the classification precision of SVM classifiers is higher than that of BP artificial neural networks. Thus SVM classifiers show better classification performance.
Key words: support vector machine; wavelet packet analysis; feature extraction; fault diagnosis; multiple fault classifiers
在机械故障诊断领域中,人工神经网络作为解决复杂非线性问题的有效工具得到广泛应用。但由于这项技术缺乏坚实的理论基础,而且神经网络存[CM(22] 在一些诸如收敛速度慢、局部极小点、过学习与欠学习以及需要大量的故障数据典型样本等不足,制约了人工神经网络在智能故障诊断中进一步应用和发展。近年来支持向量机(SVM)受到广泛关注,它以统计学习理论为基础,具有简洁的数学形式,能进行直观的几何解释,并具有良好的泛化能力,避免了局部最优解,有效地克服了“维数灾难”,且需要人为设定的参数少,便于使用,为小样本机器学习提供了一种新方法,已应用于模式识别、回归分析和函数逼近等领域[1-4]。在此,作者将支持向量机方法应用于机械故障诊断领域,建立了基于支持向量机的多故障分类器,并与BP神经网络分类器进行比较。
1 支持向量机原理
统计学习理论(SLT)是V.VAPNIK在20世纪70年代末提出的一种有限样本统计理论,是针对小样本统计问题而建立的一套新的理论体系[5]。统计学习理论给出了结构风险最小化原理和VC维(Vapnik-Chervonenkis Dimension)的概念,指出经验风险最小不能保证期望风险最小,提出为了最小化期望风险,必须同时最小化经验风险和VC维[5]。与传统统计学相比,统计学习理论着重研究有限样本情况下的统计规律和学习方法,在这种体系下的统计推理规则不仅考虑了对渐进性能的要求,而且追求得到现有信息条件下的最优解,并在此基础上发展了一种新的通用学习算法,即支持向量机。支持向量机是一种建立在统计学习理论和结构风险最小化原理基础上的新型学习机器,它根据有限的样本信息在模型的复杂性(即对特定训练样本的精度)与学习能力(即无错误地识别任意样本的能力)之间寻求最佳折中,以获得最强的推广能力[5-7]。
支持向量机的研究最初是针对模式识别中的两类线性可分问题。设样本集(xi,yi),x∈Rd,yi∈{+1,-1},i=1,2,…,n。其中:n为训练样本个数;d为每个训练样本向量的维数;yi为分类类别(其值等于+1时为一类,等于-1时为另一类),其最优分类函数为

式中:sgn(·)为符号函数;α*i和w*0分别为最优的Lagrange乘子值和阀值。
对非线性情形,SVM的基本思想是将样本通过非线性映射函数由低维空间映射到高维特征空间,使其线性可分,再在高维特征空间求最优分类面。这种非线性映射函数称为核函数。根据泛函的有关理论,只要核函数K(xi,xj)满足Mercer条件,它就对应某一变换空间的内积,即

这样,在高维特征空间建立的优化超平面为:

于是,原样本空间的两类分类问题可表示为:

考虑到有些样本有可能不能被式(3)分开,引入非负的松弛变量ξi,作为在确定最优分类面时折中考虑最大分类间隔和最小错分样本,常数C控制着对错分样本惩罚的程度,这样,优化方程改写为:
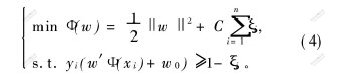
其中:i=1,2,…,n。利用Lagrange乘子法使原问题转化为简单的对偶问题进行求解,即
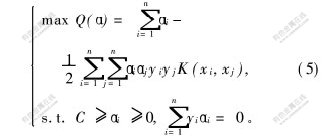
根据Kuhn-Tucker条件,优化系数αi必须满足:

因此,只有一小部分αi不为零,它们对应的训练样本即为支持向量。通过式(5)和(6)求出α*i和w*0后,对于给定的测试样本x,支持向量机分类函数的一般形式为:

因此,可据分类函数的正负判别样本x所属的类别。
目前,在模式识别方面,常用的核函数主要有[8]:多项式核函数K(xi,x)=[(xi·x)+1]p,高斯径向基核函数K(xi,x)=exp[-|x-xi|2/(2σ2)]和双曲正切核函数K(xi,x)=tanh[a(xi·x)+b]。
2 小波包故障特征提取算法
小波分析是一种时频分析方法,对非平稳瞬态信号具有宽频响应的特点,具有良好的时频域定位特性,在低频处有较高的频率分辨率,在高频处有较高的时间分辨率[9,10]。而小波包方法是在多分辨分析基础上构成的一种更精细的正交分解方法,在全频带对信号进行多层次的频带划分。它继承了小波变换所具有的时频局部化的优点,又继续对小波变换没有再分解的高频频带进行进一步分解,从而提高了频率分辨率。因此,小波包具有更广泛的应用价值。
小波包信号分解是将包括正弦信号在内的任意信号划归到相应的频带。这些分解频带信号都具有一定的能量,因此,可以用每个频带里信号能量作为特征向量来表征设备的运行状态。一方面,可以根据感兴趣的信号频率范围,把信号在一定的尺度上进行分解,从而提取相应频带内的信息。另一方面,可以对频带内的信号进行统计分析,形成反映信号特征的特征向量。若分析各频带内的信号能量,则称为小波频带能量分析。
由于采用的小波包分解是正交分解,各频带的信号相互独立,它们无冗余,不泄漏,遵守能量守恒定律,所以,小波包频带分析技术和Fourier频谱分析技术一样,它的理论依据也是Parseval能量积分等式。小波变换中,原始信号f(x)在L2(R)上的信号2范数定义为[11,12]:

因此,小波变换中信号2范数的平方等价于原始信号在时域的能量。f(x)的小波变换为:

其中:ψ(x)为基小波。据式(8)和式(9)以及Parseval能量积分等式,有

由式(10)可知,小波变换系数Ckj具有能量的量纲,可以用于故障诊断的能量特征提取。设小波包分解后第k层第j个频带的重构信号Skj对应的信号能量为Ekj,则有

其中:N表示数据长度;k表示小波包分解层次;j=0,1,2,…,2k-1,表示分解频带的序号,p=2k-1;xjm表示重构信号Skj的离散点的幅值。信号总能量Ek等于各频带能量之和,即

对式(12)的特征因子进行能量归一化后可得到小波包分解提取的故障特征向量T:

3 基于SVM的故障诊断方法
3.1 样本数据及特征向量提取
以某铝业公司关键设备煤气排送机为诊断对象。该排送机型号为D350-2.5/1.0208,出口流量为350 m3/min,进口压力为0.1 MPa,介质进口密度为0.95138 kg/m3,出口压力为0.249 MPa,风机转速为6627 r/min。用台湾研华公司生产的模拟量数据采集卡PCI-1713采集数据,选用美国本特利330500压电式速度传感器, 该传感器中集成有积分电路,可以把原始的加速度信号转变成速度信号。数据采集采用整周期同步采样,每周32点,采集32个周期,共计1024点。根据以前采用恩泰克PAC 1500便携式数据采集器采集的典型数据、目前在线监测系统采集到的数据和现场故障诊断的经验,按照机组正常和4种常见故障(不平衡,不对中,基础松动和油膜涡动)共5种工作状态在频域的特点来建立多故障分类器。每种工作状态选40组样本组成训练样本集,其中每个样本含3个传感器(水平、垂直、轴向)的振动数据。特征向量的提取步骤为:
a. 将每个传感器测得的振动信号用N=20的Daubechies小波进行4层小波包分解。
b. 对小波包系数进行重构,提取各频带范围的信号。
c. 由式(11)和(12)求各频带信号的总能量E4j(j=0,1,2,…,15)和总能量E4。
d. 以各分解频带的信号能量E4j占总能量的百分比为元素来构造特征向量。
这样,构成了一个16×3=48维的特征向量,最后将这40×5=200组48维特征向量作为训练样本输入到由多个支持向量机构成的多故障分类器中进行故障识别和分类。图1所示为油膜涡动时测得的水平方向振动波形、频谱以及进行4层小波包分解后的波形示意图,其中S140~S14f为小波包分解后各频带信号重构波形。水平方向信号小波包分解后16个频带的能量形成了一个16维向量,规一化后为: [CM(22][0.7119 0.2149 0.0031 0.0129 0.0012[CM)]
[CM(22]0.0018 0.0023 0.0016 0.0029 0.0022[CM)]
[CM(22]0.0020 0.0029 0.0034 0.0054 0.0124[CM)]
0.0188]。从规一化后的特征向量中可以看出,0.5倍频(55.2 Hz)位于第1频带(0~62.5Hz),1倍频(110.5 Hz)位于第2频带(62.5~125.0 Hz),这2个频带能量较大,符合油膜涡动的振动特征[13]。
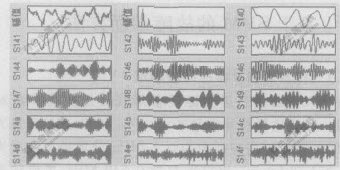
图 1 油膜涡动水平方向振动波形、频谱和
小波包分解示意图
Fig. 1 Graph of waveform, frequency
spectrum and wavelet packet decomposition
of oil film turbulence in horizontality
3.2 多故障分类器的建立
以上述5种状态数据样本集合200个作为SVM训练样本,将其中的某一种状态样本(40个)作为一类,标识为+1,将其余的4种状态样本160个作为另一类,标识为-1。在求出α*i和w*0后,按式(7)分别建立了对应5种工作状态的4个两类分类器SVM1~SVM4,分别用于将某一种故障和其他故障分开,然后将4个分类器按图2所示的树结构进行组合,形成一个可以判断5种工作状态的多故障分类器。在分类测试中,先将训练样本输入SVM1,若式(7)中f(x)的值为1,则认为是不平衡故障,否则进入SVM2继续进行分类判别,依此类推直至进入SVM4分类测试为止。
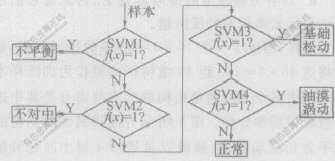
图 2 基于SVM的多故障分类器
Fig. 2 Multiple fault classifiers based on SVM
3.3 结果分析
为了检验SVM多故障分类器的分类效果,取测得振动信号的另外180组数据作为测试样本进行测试。在进行对比试验时,一方面选用高斯径向基核函数(σ=0.01)和双曲正切核函数(a=2,b=1);另一方面,为了对比支持向量机与神经网络的分类效果,还设计了网络结构为48-18-5的BP神经网络,训练目标误差β分别取0.010,0.005和0.001,采用与SVM多故障分类器相同的训练样本和测试样本进行训练和测试。此外,为了说明噪声对分类器性能的影响,在训练和测试时对样本数据加入占信号幅值15%的白噪声信号。支持向量机与BP神经网络分类结果见表1。
从表1可以看出,不论有没有加入噪声,针对相同数量样本,SVM的分类准确率均高于BP神经网络的分类准确率,从而表现出更好的分类性能。样本数据加入白噪声后,分类器的分类性能有所下降。
表 1 支持向量机与BP神经网络分类结果
Table 1 Results of classification of SVM
and BP artificial neural network
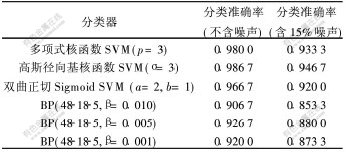
另一方面,选用不同核函数及其参数的SVM分类器对分类准确率有影响。BP神经网络的分类准确率也没有因为提高分类精度而明显提高,而且出现了过学习现象。由此可见,SVM比BP神经网络具有更好的鲁棒性和更强的分类能力。
4 结 论
a. 提出了基于SVM的故障诊断方法,该方法只需少量训练样本,而且不必预先知道故障分类的先验知识,故障分类知识隐式地体现在支持向量及其相应的Lagrange系数上,即仅用支持向量就决定了分类器的性能,表明SVM具有较强的推广能力,有利于设备故障诊断。
b. 对小波包分析和信号频带能量分解算法进行了研究,指出将该算法用于机械故障特征提取是可行的。
c. 选用不同核函数及其参数的SVM分类器对分类准确率有影响。将样本数据加入白噪声后,分类器的分类性能有所下降。与常用的BP神经网络相比,基于SVM的多故障分类器具有更强的分类能力和鲁棒性,且具有算法简单、重复训练样本少等优点。
d. 对某排送机实测振动信号进行故障诊断,达到了预期的效果,表明该方法的正确性和有效性,为设备故障智能诊断提供了一种新的方法。
参考文献:
[1]POYHONEN S, NEGREA M, ARKKIO A. Fault Diagnostics of an Electrical Machine with Multiple Support Vector Classifiers[A]. Proceedings of 2002 IEEE International Symposium on Intelligent Control[C]. Vancouver, 2002.
[2]GUYON I, WESTON J, BARNHILL S. Gene Selection for Cancer Classification Using Support Vector Machines[J]. Machine Learning, 2002, 46(1-3):389-422.
[3]GUNN S R. Support Vector Machines for Classification and Regression[R]. Southampton: Department of Electronics and Computer Science, University of Southampton, 1998.
[4]MA Yong, DING Xiao-qing. Face Detection Based on Cost-sensitive Support Vector Machines[A]. LEE Seong-whan, VERRI A. Pattern Recognition with Support Vector Machines[C]. Niagara Falls, Berlin: Springer Press, 2002: 260-267.
[5]VAPNIK V. The Nature of Statistical Learning Theory[M]. New York: Spring-Verlag, 1995.
[6]张学工.关于统计学习理论与支持向量机[J].自动化学报,2000,26(1):32-42.
ZHANG Xue-gong. Introduction to Statistical Learning Theory and Support Vector Machines[J]. Acta Automatica Sinica, 2000, 26(1): 32-42.
[7]BURGES J C C. A Tutorial on Support Vector Machine for Pattern Recognition[J]. Data Mining and Knowledge Discovery, 1998, 2(2):121-167.
[8]边肇祺,张学工.模式识别(第2版)[M].北京:清华大学出版社,2000.
BIAN Shao-qi, ZHANG Xue-gong. Pattern Recognition (2nd edition)[M]. Beijing: Tsinghua University Press, 2000.
[9]黄名辉,李子萌.小波神经网络在齿轮箱故障诊断中的应用[J].中南工业大学学报(自然科学版),1998,29(5):490-493.
HUANG Ming-hui, LI Zi-ming. The Application of Wavelet Neural Network for Faults Diagnosis of Gearbox[J]. Journal of Central South University of Technology (Science and Technology), 1998,29(5):490-493.
[10]秦宣云,卜英勇.基于小波变换的故障信号检测[J].中南工业大学学报(自然科学版),2002,33(4):434-437.
QIN Xuan-yun, BU Ying-yong. Fault Signal Detection Based on Wavelet Transform[J]. Journal of Central South University of Technology (Science and Technology), 2002,33(4): 434-437.
[11]周小凯,严谱强.用小波分析铁路车辆滚动轴承诊断方法[J].清华大学学报(自然科学版),1996,36(8):29-33.
ZHOU Xiao-kai, YAN Pu-qiang. New Method Diagnosis of Railway Roller Bearing Based on the Wavelet Analysis[J]. Journal of Tsinghua University (Science and Technology), 1996, 36(8): 29-33.
[12]邢双喜,冷军发,李臻.基于小波包分析的机械故障特征提取方法研究[J].煤矿机械,2003,(3):92-94.
XING Shuang-xi, LENG Jun-fa, LI Zhen. Study on the Extraction Method of Mechanical Fault Symptom Based on Wavelet Packet Analysis[J]. Coal Mine Machinery, 2003,(3):92-94.
[13]刘朝山,张继华.油膜涡动和油膜振荡的特征频率[J].风机技术,2001,(6):52-54.
LIU Chao-shan, ZHANG Ji-hua. Characteristic Frequency of Oil Film Turbulence and Oscillation[J]. Compressor Blower and Fan Technology, 2001,(6):52-54.
收稿日期:2004-06-21
作者简介:何学文(1971-),男,江西赣州人,博士研究生,从事分布式故障诊断和机器学习研究
论文联系人: 何学文,男,博士研究生;电话:0731-8836819(O); E-mail: hxw993@vip.163.com