DOI: 10.11817/j.issn.1672-7207.2016.05.020
基于改进BOF算法的图像识别和分类
李康顺1, 2,王福滨1,张丽霞1,李伟1, 2
(1. 华南农业大学 信息学院,广东 广州,510642;
2. 江西理工大学 信息工程学院,江西 赣州,341000)
摘要:对bag of features(BOF)算法进行研究与改进,并将其应用到图像识别和分类中。针对传统BOF算法执行效率低以及分类精度不够高等缺陷,提出一种结合SURF(speeded up robust feature)与空间金字塔匹配原理的优化方法相结合的图像识别与分类算法。SURF算法可提高执行效率,而空间金字塔匹配原理的优化方法可提高分类精度。首先对分类图像应用SURF算法提取特征描述符并生成视觉词典,该算法提取的视觉词典能更有效地表示图像特征,且能应对多变的尺度;然后应用空间金字塔匹配原理对图像采用视觉词典的直方图表示,进一步提高分类的准确度;最后利用LIBSVM分类器进行分类。在Graz,Caltech-256和Pascal VOC 2012这3个数据集中进行实验测试。研究结果表明:该方法与传统的BOF算法相比提高了执行效率和分类精度。在数据实验中通过与近几年一些相关研究工作在分类准确率方面进行对比,该方法具有很大的优越性。
关键词:bag of features算法;图像识别分类;SURF;空间金字塔匹配
中图分类号:TP391 文献标志码:A 文章编号:1672-7207(2016)05-1599-07
Image recognition and classification based on improved BOF algorithm
LI Kangshun1, 2, WANG Fubin1, ZHANG Lixia1, LI Wei1, 2
(1. College of Information, South China Agricultural University, Guangzhou 510642, China;
2. School of Information Engineering, Jiangxi University of Science and Technology, Ganzhou 341000, China)
Abstract: Improved bag of features (BOF) algorithm was applied to image recognition and classification. In view of the low efficiency and low classification accuracy of the traditional BOF algorithm, a new recognition and classification algorithm combined SURF (speeded up robust feature) with spatial pyramid matching principle was proposed. SURF algorithm can improve the efficiency, and spatial pyramid matching principle can improve the classification accuracy. Firstly, the image feature was extracted by SURF algorithm and the codebook was generated using the features which were able to respond the changing scales. Secondly, the spatial pyramid matching principle was applied to the image histogram’s codebook which can improve the accuracy of the classification. Finally, the image histogram’s codebook was used to be the input of LIBSVM classifier. The experiments were carried out based on Graz, Caltech-256 and Pascal VOC 2012. The results show that the proposed method is better than the traditional method in the efficiency and classification accuracy. In addition, the proposed method is compared with some related research work in classification accuracy, and the proposed method has obvious advantages.
Key words: bag of features algorithm; image recognition and classification; SURF; spatial pyramid matching
随着多媒体技术的不断发展,图像逐渐成为一种主流的视觉信息载体。在图像处理和应用领域,图像识别和分类一直是研究的热点。而随着网络全球化的发展以及涌现出来的各种门户网站,使得图像数量大量激增,这给图像识别和分类带来了巨大的困难。如何在浩瀚的数据中准确地获取用户所需的信息并进行处理,成为计算机视觉领域中亟待解决的问题之一。目前已经有很多比较成熟的图像识别和分类算法,BOF(bag of features)算法就是其中之一。BOF算法的思想来自于BOW(bag of words)[1-2]算法,BOW算法最初用于文档匹配。近年来,由CSURKA等[3]将该算法的思想移植到图像处理领域[4],通过对图像采用SIFT等相关的特征提取算法将图像转换为特征描述符,得到大量特征用来表示图像的关键词;然后采用K-Meams聚类方法对得到的大量单词进行聚类,将聚类的中心定义为视觉单词,K-Meams算法得出所有的聚类中心构成视觉词典;最后将分类图像进行统一处理,通过SVM分类器对其进行分类。有部分研究者将该算法应用到行为识别[5]中,提高了识别率。还有部分研究人员创新地将该算法应用到糖尿病患者的食品识别系统中[6],大大地提高了系统的鲁棒性。近年来,BOF算法在医疗领域中也取得了重要成果[7-8],为医疗事业做出了不少的贡献。然而,BOF算法在图像识别和分类问题的应用中存在一定的问题,执行速度不够快,分类精度不够高,因此,需对其进行优化。传统的BOF算法采用SIFT(scale-invariant feature transform)[9]特征描述符。K-Means聚类方法和SVM (Support Vector Machine)分类器来实现。由于SIFT算法对复杂背景的特征提取鲁棒性不足,为了提高算法的鲁棒性,文献[10]提出了一种基于部分模型的方法,但该方法复杂度比较高。简单的应用K-Means聚类方法和SVM分类器实现的BOF算法拟合度和分类的精度都不够高,文献[11] 通过使用概率学的思想,通过最大期望(Expectation Maximization,EM)算法对BOF模型进行拟合,虽然在一定程度上提高了精度,但对于复杂背景的图像仍然存在着效率低以及分类精度不高的不足。本文作者针对BOF算法的缺点以及现有研究的不足,采用一种SIFT的改进算法SURF(speeded up robust feature)[12]算法来提取图像特征描述符,以降低BOF算法的复杂度、提高运行效率以及解决存储要求过高的问题;引入空间金字塔匹配原理并采用基于视觉词典的直方图表示,提高BOF算法分类的精度;为了提高分类的效率,采用快速有效的LIBSVM[13]分类器。将SURF算法和空间金字塔匹配原理结合用到BOF算法中,在Graz,Caltech-256以及Pascal VOC 2012这3个数据集下都可以达到较好的分类效果。
1 改进的BOF算法
本文应用BOF算法有3个步骤:特征提取和特征描述符的转化、视觉词典的构建、识别和分类。图1所示为BOF算法的基本流程。
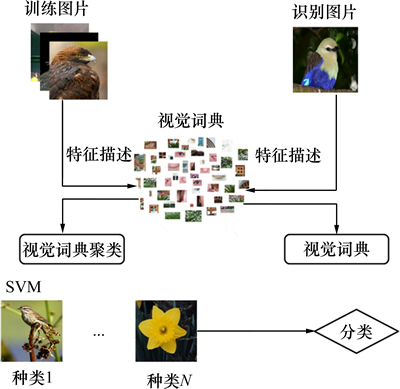
图1 BOF算法的基本流程
Fig. 1 Basic flow of BOF algorithm
根据图1,应用BOF算法的实现过程可以归纳如下。
首先,本文采用SURF算法代替传统的SIFT算法,利用Hessian矩阵来检测特征点,每个特征用64维的向量进行表示,从图像中抽取出具有代表性的全局特征和局部特征作为图像的描述符。然后,采用K-Meams聚类方法,本文并没有指定聚类中心,而是通过随机选取聚类中心,通过有限次数的迭代,最终收敛出聚类中心即词典。传统的方法将图像表示成为统计直方图的形式,如图2所示。而本文引用了空间金字塔匹配方案,提高了分类的准确度。最后使用LIBSVM分类器对其进行分类。
1.1 SURF算法
在BOF算法中进行特征提取时,常用的特征描述符的算法有SIFT,GLOH(gradient location-orientation histogram)[14]和PCA-SIFT等[15]。SIFT算法是不变性特征检测技术的一种基于尺度空间,对图像缩放、旋转、光照变化甚至仿射变换保持不变性的图像局部特征描述方法。但是该算法计算数据量大,时间复杂度高,算法耗时较长。针对上述缺点,本文提出一种基于SURF算法的特征描述符方法,以提高BOF算法的运算速度和性能。
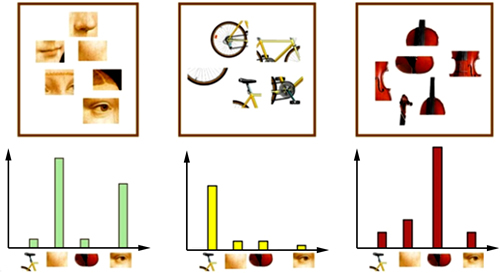
图2 基于视觉单词的直方图
Fig. 2 Histogram based on visual word
SURF算法的基本流程主要包括特征点检测(构建Hessian矩阵,构建尺度空间,精确定位特征点),主方向确定以及生成特征点描述符。
1.1.1 特征点检测
在图像I中
处的点,在尺度
上的Hessian矩阵定义为
(1)
式中:
为高斯滤波二阶导数
和
的卷积,
;
和
的含义与
的含义类似。
本文采用方框滤波(box filters)近似代替二阶高斯滤波,采用计算量与模板大小无关的积分图像[16]加速卷积以提高计算速度。如图3所示,用一个9×9的方框滤波模板近似最低尺度
的高斯二阶导数。
,
和
为方框滤波和图像卷积后的值,用于分别代替
,
和
,矩阵区域中的权值使用经验值0.9,进一步求得Hessian矩阵的det表达 式为
(2)
要实现特征点的尺度不变性,本文采用图像金字塔来实现尺度空间,通过图像与不同尺度下的高斯函数卷积,并对初始图像采样处理,得到更高阶的图像金字塔,通过不同尺度下的滤波模板对原始图像处理,使得原始图像保持不变。
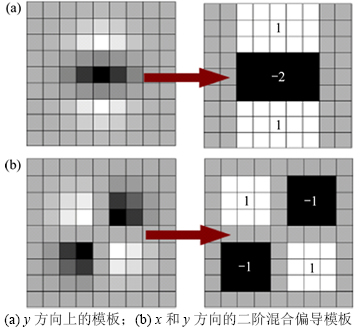
图3 方框滤波对二阶偏导的近似
Fig. 3 Box filter approximations for the second order partial derivative
1.1.2 主方向确定
为了保证图像具有旋转不变性,对于每个特征点,以该特征点为中心,画出6s(s为特征点的尺度)为半径的圆领域,并计算半径内所有特征点x和y方向上的Haar小波响应,根据特征点与中心点的距离赋权值,越近权值较大;然后将60°范围内响应的特征点形成新的矢量,遍历整个圆形区域后,选择最长矢量方向作为该特征点的主方向。
1.1.3 生成特征点描述符
确定了主方向之后,需要生成特征描述符。以特征点为中心,将坐标轴x方向旋转到主方向,按主方向选择1个20s×20s的正方形区域,并将该窗口区域划分成4×4的子区域,每个子区域为5s×5s,计算每个子区域的Haar小波响应dx和dy (dx和dy分别为相对于主方向水平和垂直方向的响应);然后对区域中的响应和响应的绝对值dx,dy,
和
求和,得到4维矢量
。这样,对每一个特征点,形成了4×(4×4)=64维的特征向量,相对于SIFT算法的128维,减少了一半,如图4所示。SURF算法的特征点的个数要比SIFT算法的个数少,减少了存储的容量。本文还对SURF算法提取的特征向量进行归一化处理,提高了算法的鲁棒性。
1.2 空间金字塔匹配下的BOF算法表示
空间金字塔匹配[17]主要包含金字塔匹配核以及空间金字塔在BOF算法下的匹配方案[18]。金字塔匹配的核心思想是将特征集映射到高分辨率空间进行匹配,在匹配的过程中,随着层次划分的密集性增加,分配的权值会变大。
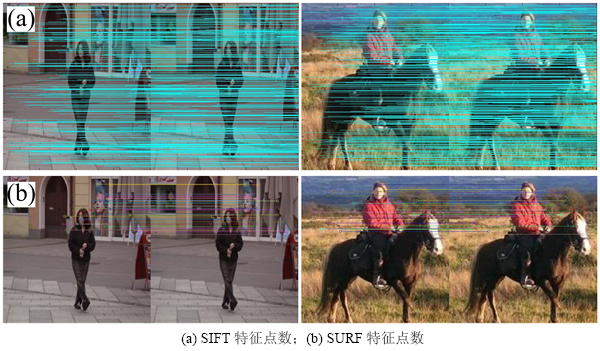
图4 SIFT与SURF特征点数的对比
Fig. 4 Contrast SIFT and SURF feature points
1.2.1 金字塔匹配核
假设X和Y分别表示d维特征空间中的向量集,本文构造网格分辨率的序号为0,…, L,其中
,以
划分每一维时得到
个子集,在d维的特征空间中总的维数
。令
和
分别表示X和Y的直方图,
和
分别表示X和Y中落入第i个子区域的点的个数。在l层上给出的直方图交叉函数匹配的数为
(3)
简称
为
。通过观察,当分层为
时,l层和
层之间的差别匹配数可以表示为
,其中
。l层的权值为
。综上所述,金字塔匹配核的定义如下:
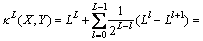
(4)
1.2.2 空间金字塔在BOF算法下的匹配方案
本文应用空间金字塔的匹配方案,量化特征向量为M个离散的类型,即表示视觉单词的数量,采用K-Means聚类将图像的特征聚类到视觉单词类别中,再将视觉单词采用直方图的形式表示。本文方法极大简化了匹配的计算,只需要比较2个视觉单词是否一致。最终的核函数总和定义为
(5)
空间金字塔的BOF表示如图5所示。
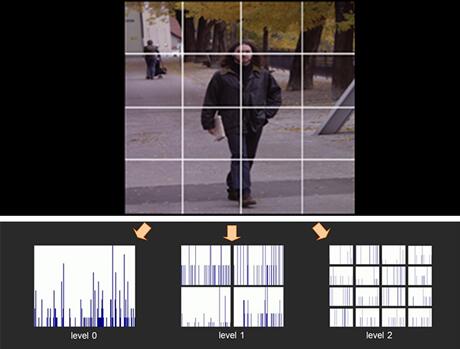
图5 空间金字塔的BOF表示
Fig. 5 BOF spatial pyramid representation
通过对图像进行不同层级的划分,并且进行基于视觉单词的直方图表示,根据式(4)计算每个视觉单词的金字塔匹配核之后,利用式(5)对所有的视觉单词求和,最终利用SVM分类器对其分类。从图5可以看出:当L=0时,就是标准的BOF算法。对于具有L层和M个视觉单词时,结果集的向量维数如下:
(6)
本文将数据集中的图像统一归一化为200×200像素,当L增大时,维数会呈指数级增长,因此,要控制L的大小。本文通过大量测试,发现当L=3时,在兼顾效率的同时分类准确率较高,因此,本文的所有实验都采用L=3。
1.3 LIBSVM软件包
LIBSVM是由LIN开发设计的一个简单、易于使用且快速有效的SVM模式识别和回归的软件包[13],LIBSVM所涉及的参数调节相对较少,提供了很多的默认参数,而且运行速度比较快,完全可以解决本文的识别和分类问题。
LIBSVM沿用SVM分类器的思想,SVM的核心思想是寻找最优的分类面,对空间中不同的数据集进行分类。SVM可以描述为如下最优化问题[19]:
(7)
约束条件为:
≥
;
≥0;i=1, …, n。其中:
;
,表示数据点所属的类别;w为与最优分类面垂直的向量;C为惩罚因子;
为稀疏变量。
使用LIBSVM软件包代替传统的SVM,不仅减少了代码量,而且提高了效率。
2 实验结果及分析
本文采用识别分类中最著名的3个数据集即Graz,Caltech-256和Pascal VOC 2012。这3个数据集具有图像数量庞大,图像种类繁多,对象类内变化多样等特点,用来测试识别和分类算法很具有代表性。其中Pascal VOC中具有较多的噪声,分类的难度比较大,最具挑战性。采用图像数据的种类包括Bike,Car,Person,Ketch,Office,Outdoor,Running,Shooting,Watch,Biking,HorseRiding,PlayingGuitar,Bird,Cat,Flowers,Horse,Cake,Grasshopper和Helicopter。
为了突出本文方法的优越性,将在人脸识别中识别率很高的Adaboost分类器与LIBSVM分类器进行对比。
2.1 实验结果
本文每类都统一使用40幅图像作为训练样本,使用20幅图像作为测试样本,保证了数据量的充足。图6所示为节选的数据集的一部分。
表1所示为Graz,Caltech-256和Pascal VOC 2012这3个数据集中传统的BOF算法与本文方法的比较 结果。
表2所示为在Pascal VOC 2012数据集中使用本文方法采用Adaboost分类器与LIBSVM分类器的对比结果。
表3所示为本文方法与其他相关方法在Caltech-256数据集中的对比结果。
为了突出本文算法的效率,节选部分图像中SIFT算法与SURF算法效率的对比。表4所示为这2种算法的平均特征点数与运行时间的比对结果。
2.2 实验分析
从表1可以看出:应用SURF提取特征描述符以及对图像进行空间金字塔匹配,与传统的BOF算法相比,在匹配准确度方面得到了极大提高。因为SURF算法产生的视觉单词更具有代表性,而且本文通过归一化处理,在一定程度上避免了背景信息和位置变化等带来的干扰和影响,通过空间金字塔的划分细化,极大地提高了算法的鲁棒性。因此,本文算法在背景比较复杂、噪声比较多的Pascal VOC 2012数据集中也具有优越性。
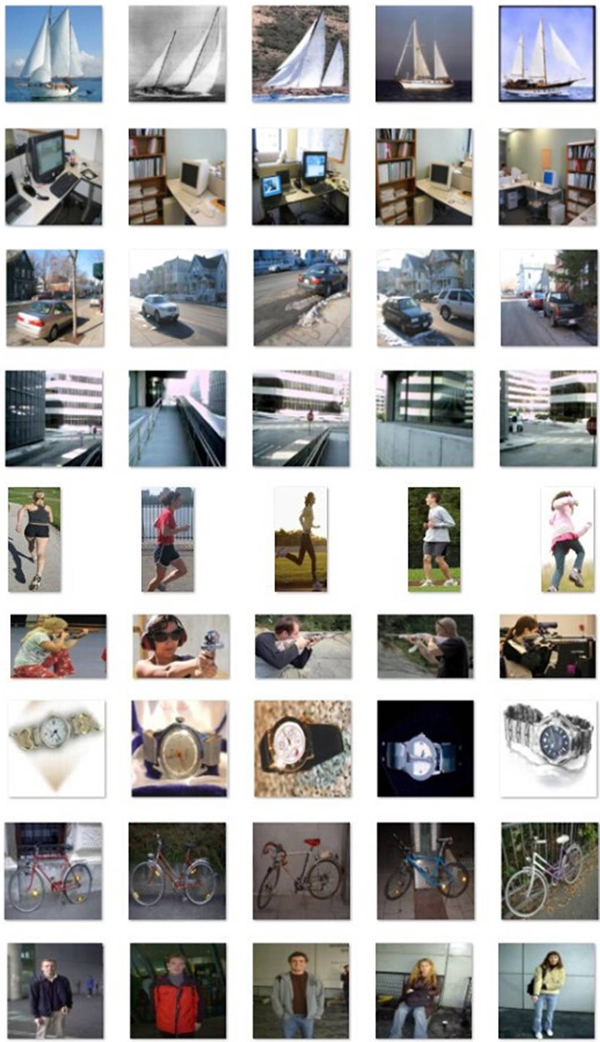
图6 所选数据集的部分图像
Fig. 6 Part of image of selected dataset
表1 本文方法与传统方法在3大数据集下的对比
Table 1 Compare this method with traditional method at three datasets
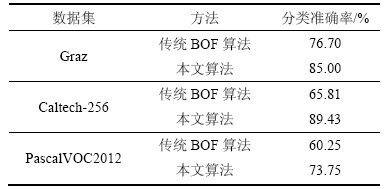
表2 本文方法在不同分类器下的对比
Table 2 Contrast to this method at different classifiers
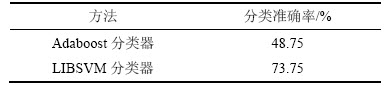
表3 本文方法与其他相关方法的对比
Table 3 Compare this method with other related methods
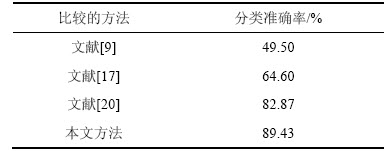
表4 SIFT算法与SURF算法效率对比
Table 4 Compare efficiency of SURF and algorithm SIFT algorithm
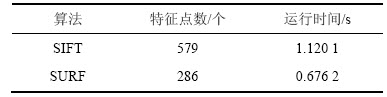
图7所示为Pascal VOC 2012这个数据集中的实验结果(横坐标和纵坐标代表图像的类别,对角线深色部分代表分类的准确率,其他格子代表误差)。从图7可见:虽然在这个最有挑战性的数据集中有些分类结果不是很好,但整体上,本文的方法可以适应复杂的图像,并且具有良好的效果。
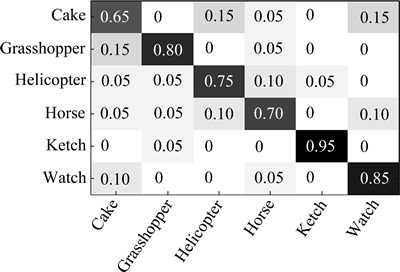
图7 在Pascal VOC 2012下的实验结果
Fig. 7 Result of Pascal VOC 2012 dataset
从表2可以看出:在人脸识别中具有极好表现的Adaboost分类器,应用于BOF算法中的效果远远没有LIBSVM分类器的效果突出,LIBSVM不仅在分类的准确性上超越了Adaboost,在执行效率方面也有明显的优势。
从表3可以看出:本文与当前一些相关研究工作对比,本文算法的分类准确率较高。
从表4可以看出:经过改良的算法其执行效率提高了将近1倍,这对于图片量比较大时进行分类与识别具有重大意义。
3 结论
1) 将SURF算法和空间金字塔相结合,应用到BOF算法中进行图像识别与分类。SURF算法用特征描述符生成视觉词典更能代表所要分类图像的特征,比传统方法更具鲁棒性,并且在一定程度上适应了多边尺度的图像。
2) 基于空间金字塔匹配原理,通过对图像进行划分,充分利用图像的空间信息,使得匹配精确度得到极大提高。通过实验结果对比,本文算法达到了良好的分类效果,并且与近几年来的相关算法相比具有一定的优越性。
参考文献:
[1] WU Lei, HOI S C H, YU Nenghai. Semantics-preserving bag-of-words models and applications[J]. IEEE Transactions on Image Processing, 2010, 19(7): 1908-1920.
[2] ZHENG Zhenzhu, YAN Luxin. Global and local explotation for saliency using bag-of-words[J]. IET Computer Vision, 2014, 8(4): 299-304.
[3] CSURKA G, DANCE C, FAN L, et al. Visual categorization with bags of keypoints[C]// Proceedings of IEEE Conference on Workshop on Statistical Learning in Computer Vision. Centre Europe, 2004: 1-22.
[4] SIVIC J, LAB D, ECOLE N, et al. Efficient visual search of videos cast as text retrieval[J]. IEEE Transactions on Pattern Analysis and Machine Intelligence, 2009, 31(4): 591-606.
[5] YU J, JEON M, PEDRYCZ W. Weighted feature trajectories and concatenated bag-of-features for action recognition[J]. Neurocomputing, 2014, 131(2): 200-207.
[6] ANTHIMOPOULOS M M, GIANOLA L, SCAMATD L, et al. A food recognition system for diabetic patients based on an optimized bag-of-fetures model[J]. IEEE Journal of Biomedical and Health Informatics, 2014, 18(4): 1261-1271.
[7] SHEN Linlin, LIN Jiaming, WU Shengyin, et al. HEp-2 image classification using intensity order pooling based features and bag of words[J]. Pattern Recognition, 2013, 47(7): 2419-242.
[8] PIRES R, HELINEK H F, WAINER J, et al. Advancing bag-of-visual-words representations for lesion classification in retinal images[J]. Plos One, 2014, 9(6): e96814.
[9] LOWE D G. Distinctive image features from scale-invariant key points[J]. International Journal of Computer Vision, 2004, 60(2): 91-110.
[10] LI Feifei, FERGUS R, PERONS P. Learning generative visual models from few training examples: an incremental Bayesian approach tested on 101 object categories[J]. Special Issue on Generative Model Based Vision, 2007, 106(1): 59-70.
[11] LEUNG T, MAILIK J. Representing and recognizing the visual appearance of materials using three-dimensional textons[J]. International Journal of Compute Vision, 2001, 43(1): 29-44.
[12] BAY H, TUYTELAARS T, VAN GOOL L. SURF: speeded up robust features[C]// Computer Vision-ECCV 2006. Graz: Springer Berlin Heidelberg, 2006: 404-417.
[13] CHANG C C, LIN C J. LIBSVM: a library for support vector machines[J]. ACM Transactions on Intelligent Systems and Technology, 2011, 2(3): 27-53.
[14] HUANG L, CHEN C, SHEN H, et al. Adaptive registration algorithm of color images based on SURF[J]. Measurement, 2015, 66: 118-124.
[15] 唐建鑫. 基于Bag of Features的图像分类和检索研究[D]. 西安: 西安电子科技大学信息学院. 2012: 1-70.
TANG Jinxin. Research on image classification and retrieval based-on Bag of Features[D]. Xi’an: Xidian University. College of Information, 2012: 1-70.
[16] VIOLA P, JONES M J. Rapid object detection using a boosted cascade of simple features[C]// Conference on Computer Vision and Pattern Recognition. USA, 2001: 511-518.
[17] LAZEBNIK S, SCHMID C, PONCE J. Beyond bags of features: spatial pyramid matching for recognizing natural scene categories[J]. IEEE Computer Society on Computer Vision and Pattern Recognition, 2006, 2: 2169-2178.
[18] HUANG M, MU Z, ZENG H. Efficient image classification via sparse coding spatial pyramid matching representation of SIFT-WCS-LTP feature[J]. Iet Image Processing, 2016, 10(1): 61-67.
[19] 徐渊, 许晓亮, 李才年, 等. 结合SVM分类器与HOG特征提取的行人检测[J]. 计算机工程, 2016, 42(1): 56-60.
XU Yuan, XU Xiaoliang, LI Cainian, et al. Pedestrian detection combining with SVM classifier and HOG feature extraction[J]. Computer Engineering, 2016, 42(1): 56-60.
[20] 赵春晖, 王莹. 一种基于词袋模型的图像优化分类方法[J]. 电子与信息学报, 2012, 34(9): 2064-2070.
ZHAO Chunhun, WANG Ying. An optimized method for image classification based on bag of words model[J]. Electronics & Information Technology, 2012, 34(9): 2064-2070.
(编辑 杨幼平)
收稿日期:2015-05-26;修回日期:2015-07-12
基金项目(Foundation item):国家自然科学资金资助项目(70971043);广东省自然科学基金资助项目(2015A030313408);江西理工大学科研基金资助项目(NSFJ2015-K13) (Project(70971043) supported by the National Natural Science Foundation of China; Project(2015A030313408) supported by the Natural Science Foundation of Guangdong Province of China; Project(NSFJ2015-K13) supported by the Science Foundation of Jiangxi University of Science and Technology)
通信作者:李康顺,教授,博士生导师,从事图像处理研究;E-mail: likangshun@sina.com