DOI: 10.11817/j.issn.1672-7207.2017.08.017
一种改进的FLS-SVM分类辨识模型及其应用
左红艳1, 2,王涛生2
(1. 中南大学 资源与安全工程学院,湖南 长沙,410083;
2.湖南涉外经济学院 商学院,湖南 长沙,410205)
摘要:采用三角形函数隶属度法确定模糊最小二乘支持向量机(fuzzy least squares support vector machine, FLS-SVM)输入参数隶属度,采用自适应变尺度混沌免疫算法优化FLS-SVM的参数,从而构建改进模糊最小二乘支持向量机(improved fuzzy least squares support vector machines, IFLS-SVM)分类辨识模型, 用Ripley数据集、MONK数据集和PIMA数据集进行仿真实验,并用于地下金属矿山采场信号分类辨识与中国国际贸易安全分类辨识。研究结果表明:与LS-SVM分类辨识模型和FLS-SVM分类辨识模型相比,IFLS-SVM分类辨识模型能有效提高带噪声点和异常点数据集的分类精度,且分类辨识精度相对误差较小。
关键词:混沌免疫算法;模糊最小二乘支持向量机;分类辨识
中图分类号:TP183 文献标志码:A 文章编号:1672-7207(2017)08-2097-08
An improved FLS-SVM classification identification model and its application
ZUO Hongyan1, 2, WANG Taosheng2
(1. School of Resources and Safety Engineering, Central South University, Changsha 410083, China;
2. School of Business, Hunan International Economics University, Changsha 410205, China)
Abstract: A classification and identification model was developed based on improved fuzzy least squares support vector machines(FLS-SVM),in which the fuzzy membership function was set by using triangle function method and its parameters were optimized by an adaptive mutative scale chaos immune algorithm, and an improved fuzzy least squares support vector machines(IFLS-SVM) was constructed. The simulation experiments were conducted on three benchmarking datasets such as Ripley datasets, MONK datasets and PIMA datasets for testing the generalization performance of the classification and identification model, signals from underground metal mines stope wall rock and international trade data in China were diagnosed by the IFLS-SVM classification and identification model. The results show that compared with LS-SVM classification identification model and FLS-SVM classification identification model, the IFLS-SVM classification identification model is valid for improving the analysis accuracy of the data with noises or outliers and IFLS-SVM classification identification model has small relative error.
key words: chaos immune algorithm; fuzzy support vector machines; classification identification
对于小样本条件下的高维模式分类辨识和非线性回归问题,建立在统计学习理论的VC维理论和结构风险最小原理基础上的支持向量机(support vector machine,SVM)[1-3]比神经网络分类、决策树分类和模糊分类具有更多的优势,它可以根据有限的样本信息在模型的复杂性和学习能力之间寻求最佳折中,以求获得最强的推广能力,但其抗噪声能力弱,过拟合和多类分类精度低等,这成为制约其广泛应用于模式识别、信号分类处理和时间序列预测等领域[4-7]的瓶颈。考虑到模糊理论具有非线性映射能力强、可有效表示模糊信息以及不确定性等特点,人们提出模糊支持向量机(fuzzy support vector machines, FSVM)[8-9]和模糊最小二乘支持向量机(fuzzy least squares support vector machine, FLS-SVM)[10-11]等改进型支持向量机,以消除样本中噪声的影响,使改进型支持向量机结构更透明和具有更强的推理能力。由于模糊支持向量机和模糊最小二乘支持向量机[12-13]等改进型支持向量机要求隶属度函数必须能客观、准确地反映系统中样本存在的不确定性,且其正则化参数和核参数优化对其分类精度有很大影响[14-15],因此,如何有效构造隶属度函数来区分样本集中噪声或野值点和采用有效的优化算法对正则化参数和核参数进行优化十分重要[16-17]。考虑到三角形隶属度函数法能客观、准确地反映系统中样本存在的不确定性[18],本文作者采用三角形隶属度函数法确定FLS-SVM(fuzzy least squares support vector machine)的隶属度,同时采用自适应变尺度混沌免疫算法优化FLS-SVM的参数,建立改进模糊最小二乘支持向量机(improved fuzzy least squares support vector machines, IFLS-SVM)分类辨识模型,进行数据集分类仿真实验及实例应用。
1 改进FLS-SVM分类辨识模型构建
由于影响FLS-SVM分类精度的因素主要包括正则化参数和核参数是否优化、隶属度函数是否客观和准确地反映样本存在的不确定性,为此,采用三角形隶属度函数法确定FLS-SVM的隶属度,采用自适应变尺度混沌免疫算法优化FLS-SVM参数,从而建立IFLS-SVM分类辨识模型。
1.1 模糊最小二乘支持向量机
一般地,令模糊最小二乘支持向量机的输入模糊样本为
(x1, y1, μ(x1)),…,(xk, yk, μ(xk)),…,
(xl, yl, μ(xl)),k=1,2,…,l。
式中:xk为输入变量;yk为输出变量;μ(xk)为隶属度,0≤μ(xk)≤1。模糊最小二乘支持向量机的最优分类面为式(1)所示的目标函数的最优解[15]:
(1)
s.t. yi= wT·φ(xi)+b+εk ;εk>0;k=1,2,…,l
式中:εk为松弛变量;C为惩罚因子;b为阈值。
相应的拉格朗日函数为
(2)
式中:ai为拉格朗日系数。则模糊最小二乘支持向量机优化问题转化为求解线性方程:
(3)
式中:y=[y1,…,yk,…,yl]T;E=[1,…,1,…,1,l]T;a=[a1,…,ak,…,al]T;Ωij=φ(xk)·φ(xt)=K(xk, xt);t=1,2,…,l。
FLS-SVM分类辨识模型如图1所示,则该分类辨识模型为
(4)
式中:x=[x1,…,xk,…,xl];K(xk, x)=exp{-|xk-x|2/σ2};σ为核参数。
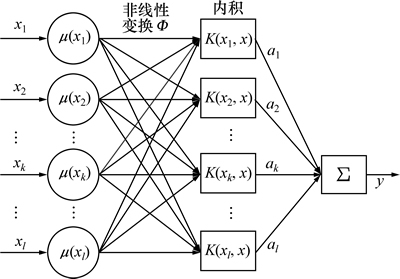
图1 FLS-SVM分类辨识模型
Fig. 1 FLS-SVM classification and identification model
1.2 FLS-SVM的隶属度确定
采用如图2所示的三角形隶属度函数法确定分类指标的隶属度,其计算公式为[19]
(5)
式中:zij为第i个分类指标的第j次实际值;ui为第i个分类指标实际值的均值;mi为第i个分类指标的模糊子集的边界,是用来确定第i个分类指标隶属度的必要条件,可以根据实际应用情况进行自适应调整,一般取mi=2σi;σi为第i个分类指标的相应实际值的方差,
(6)
T为总样本数。
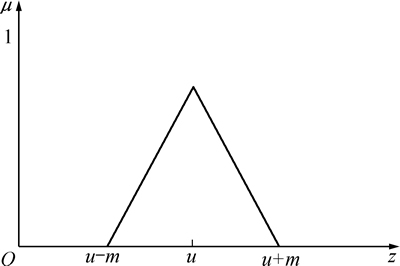
图2 三角形隶属函数
Fig. 2 Triangle subjection function
1.3 FLS-SVM的参数优化
应用FLS-SVM分类辨识模型进行分类辨识时,正则化参数C和核参数σ的选择是一个重要问题。为此,采用自适应变尺度混沌免疫算法对FLS-SVM的正则化参数C和核参数σ进行优化时,可由下式确定自适应变尺度混沌免疫算法的适应度函数:
(7)
式中:yi为期望输出;f(xi)为实际输出;e为1个很小的实数,其作用是防止分母出现0的情况,此处e为10-3。定义误差函数EMS作为FLS-SVM泛化性能的评价指标:
(8)
式中:f(xi)为实际输出;yi为期望输出。
自适应变尺度混沌免疫算法优化FLS-SVM参数的具体步骤如下。
Step 1 将x=[x1,…,xk,…,xl]作为抗原{Ag}输入,并进行标准化处理。选择sn+1=4sn(1-sn)作为混沌模型在目标函数定义域内随机产生N个初始化抗体{Ab}的混沌变量。
Step 2 对每个抗原Agi操作如下。
Step 2.1 利用式(9)分别计算每个抗体Abiz与抗原Agjz的亲和力βij,
(9)
Step 2.2 选择m个亲和力最强的抗体作为网络细胞,并对其进行克隆操作,得到相应的克隆数Nc。
Step 2.3 对第z次克隆后的细胞应用方程Cij(z+1)=Cijz-α(Cijz-Xijz)进行变异操作(其中,Cijz为第z次克隆抗体细胞数,Xijz为第z次克隆抗原细胞数,α为变异率)。
Step 2.4 计算第z次变异操作后的克隆抗体细胞数Cij(z+1)和第z-1次变异操作后的克隆抗体细胞数Cijz的亲和力。
(10)
Step 2.5 选择亲和力最强的25%的克隆抗体细胞作为记忆细胞数据集Mp。
Step 2.6 利用式(11)计算每个抗体Abi同抗体Abj间相似度λij,淘汰记忆细胞数据集Mp中相似度λij大于阈值σs的个体。
(11)
Step 3 将淘汰完毕的记忆细胞数据集Mp合并到记忆数据集M中。
Step 4 对较优个体进行混沌搜索。
选择记忆库中适应值较大的15%的个体进行混沌细搜索。设较优个体为X=(X1,…,Xt,…,XT),混沌变量搜索区间的缩小表示为
(12)
式中:
为收缩因子,φ∈(0, 0.4)。为保证新范围不越界,进行如下处理:若
<at,则
=at;若
>bt,则
=bt。因此,Xt在新区间[
,
]上进行还原处理后的向量Yt由下式确定:
(13)
将Yt与Xt的非线性组合作为新的混沌变量,用此混沌变量进行搜索。
(14)
式中:δt为自适应调节系数,0<δt<1。自适应调节系数δt采用下式进行自适应确定:
(15)
式中:K为进化代数。淘汰记忆库中适应值较大的8%的个体相似度大于σs的个体。
Step 5 选择sn+1=4sn(1-sn)作为产生N ′个(0, 1)间的个体,替换亲和力差的个体,与上次免疫计算得到的记忆数据集M作为下一代免疫计算的抗体,并返回Step 2,直到达到网络收敛为止。
Step 6 用适应度函数评价Xt*,计算相应的f(Xt*)。若f(Xt*)>f(X),则f(X)=f(Xt*),否则放弃Xt*。
Step 7 若满足截止判据EMS<10-5,则截止搜索,输出最优解X,否则返回Step 1。
1.4 IFLS-SVM分类辨识效果仿真验证
为验证IFLS-SVM分类辨识模型的分类辨识效果,用3个常用标准测试(UCI)数据集进行实验,并与LS-SVM分类辨识模型和FLS-SVM分类辨识模型相比较。
1) Ripley数据集。采用第2类Ripley数据集,其中训练集含300个样本(其中正负类各150个),测试集1 000个样本(正负类各500个)。
2) MONK数据集。采用含有随机添加的噪声点的第3类MONK数据集,训练集含130个样本(其中正、负类分别为65个和65个),测试集440个样本(其中、正负类分别为230个和210个)。
3) PIMA数据集。PIMA数据集总样本为800个(其中正、负类分别为500个和300个)。在数据集文件中随机选取600个样本训练,剩余200个样本测试。
对3个常用标准测试(UCI)数据集中数据进行归一化处理,并采用LS-SVM分类辨识模型、FLS-SVM分类辨识模型和本文中提出的IFLS-SVM分类辨识模型对其进行辨识分类,这3种方法得到的最优测试精度及达到最优测试精度时所对应的参数分别见表1和表2。从表1可以看出:本文提出的IFLS-SVM分类辨识模型能够有效提高带噪声点和异常点数据集数据的分类辨识精度。
表1 最优辨析精度
Table 1 Optimal prediction accuracy %
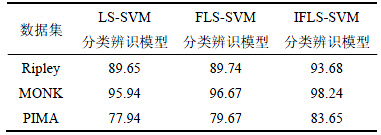
表2 最优分类辨识精度时对应参数
Table 2 Corresponding parameters when analysis accuracy is optimal
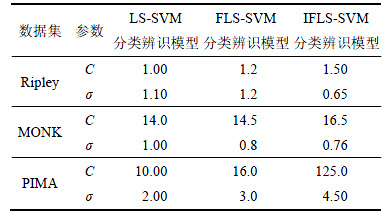
基于CPU的3种分类辨识模型计算耗时见表3。由表3可知:LS-SVM分类辨识模型和FLS-SVM分类辨识模型的CPU计算耗时较多,而IFLS-SVM分类辨识模型的CPU计算耗时最少。
表3 3种分类器的CPU计算耗时对比
Table 3 Comparison of consuming time of three kinds of classification models s
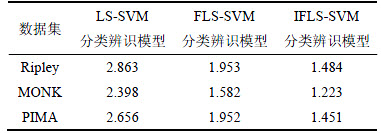
2 IFLS-SVM分类辨识模型的实际应用
2.1 在地下金属矿山采场信号分类辨识中的应用
图3所示为南方某地下金属矿山开采过程中采集的机械振动、爆破信号和采场围岩声发射信号测试结果,且各为600组。
从地下金属矿山采场围岩声发射信号及干扰信号数据中取150个有效样本数据,75个为训练集(岩石破裂信号25个、机械振动信号25个和声发射信号25个),75个为样本测试集(岩石破裂信号25个、机械振动信号25个和声发射信号25个),分别采用LS-SVM分类辨识模型、FLS-SVM分类辨识模型和IFLS-SVM分类辨识模型对地下金属矿山采场围岩声发射信号及干扰信号测试数据进行分类,其结果如表4所示。从表4可知:采用LS-SVM分类辨识模型、FLS-SVM分类辨识模型和IFLS-SVM分类辨识模型的分类精度分别为82.67%,86.67%和90.67%。可见IFLS-SVM分类辨识模型由于在构造适应度函数时,将模糊分类有效性指标和模糊分类正确样本数为适应度函数的子目标,从而使得不同的样本有不同的贡献,在较大程度上减弱了噪声和孤立点对分类的影响,使学习算法在代价敏感数据或含噪声数据的情况下更加具有稳健性。
2.2 在中国国际贸易安全分类辨识中的应用
2.2.1 中国国际贸易安全评价指标
针对中国国际贸易实际情况,选择易于获取、操作性强并且最能客观反映中国国际贸易安全现状的指标,即固定资产投资总额x1、实际利用外资金额x2、城乡居民储蓄存款年底余额x3、外汇储备x4、人民币对美元汇率x5和货币供应量x6。
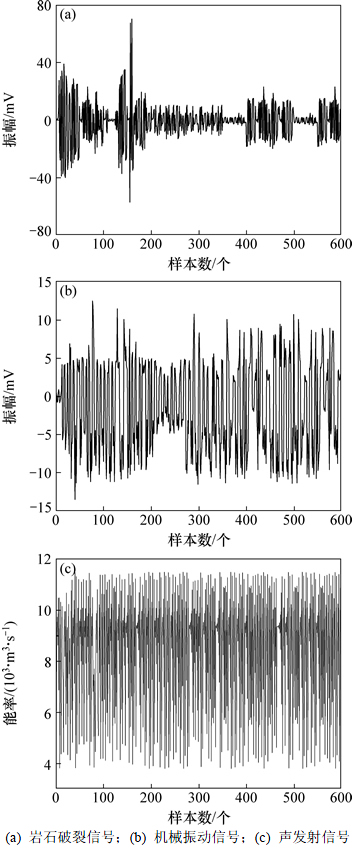
图3 地下金属矿山采场围岩声发射信号及干扰信号
Fig. 3 Acoustic emission signals from underground metal mines stope wall rock and its interference signals
表4 测试数据分类结果
Table 4 Classified results of measurement data
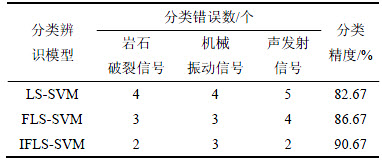
2.2.2 中国国际贸易安全性等级划分
IFLS-SVM分类辨识模型输出参数为中国国际贸易安全性等级,分为5级,IFLS-SVM分类辨识模型期望输出的5级的值依次为:A级(极不安全),R1=(l,0,0,0,0);B级(较不安全), R2=(0,1,0,0,0);C级(一般安全),R3=(0,0,l,0,0);D级(较安全),R4=(0,0,0,l,0);E级(极安全),R5=(0,0,0,0,1)。因此,IFLS-SVM分类辨识模型输出参数为中国国际贸易安全性等级值Ri(i=1,2,3,4,5)。
2.2.3 中国国际贸易安全性分类辨识
1980—2014年中国国际贸易安全性分类指标参数如表5所示[20]。以1980—2006年参数作为训练样本集,以2007—2014年参数作为测试样本,进行中国国际贸易安全性分类辨识。由于历史资料不够全面及不够准确,给专家判断中国国际贸易安全性Ri分类带来一定影响,因此,专家只能根据历史进出口贸易总值给出1980—2014年中国国际贸易安全性Ri的模糊判断,如表5所示。
为验证IFLS-SVM分类辨识模型(用模型F2表示)的有效性,用表5所示数据进行仿真实验,并与文献[10]中的分类器(用模型F1表示)分类结果进行比较。
采用式(5)和式(6)求得表5中中国国际贸易安全性指标,即x1,x2,x3,x4,x5和x6的隶属度,以中国国际贸易安全性Ri(i=1,2,…,5)作为IFLS-SVM分类辨识模型的输出,以1980—2006年的x1,x2,x3,x4,x5和x6作为模糊最小二乘支持向量机的输入,借助于建立的IFLS-SVM分类辨识模型,可得1980—2006年中国国际贸易安全性的辨析值和实际值,如表6所示。从表6可见:模型F2的辨析值与实际值的相对误差小于0.70%,具有较高的预测精度。
以中国机电产品出口贸易风险Ri(i=1,2,…,5)作为IFLS-SVM分类辨识模型的输出,以2007—2014年的x1,x2,x3,x4,x5和x6作为IFLS-SVM分类辨识模型的输入,借助于已经训练好的中国机电产品出口贸易风险智能预警分析模型,可得到2007—2014年的中国机电产品出口贸易风险的智能预警分析值和实际值如表7所示,模型F2的辨析值和实际值相对误差小于0.90%,表明IFLS-SVM具有较高的预测精度。
以IFLS-SVM分类辨识模型输出对输入分量的偏导数γi作为影响因子对中国国际贸易安全性影响程度的判据,偏导数愈大,则说明该因子的影响作用愈显著,各指标参数对中国国际贸易安全性的影响因子计算结果如图4所示。由图4可知:固定资产投资总额的影响因子γ1>货币供应量影响因子γ6>人民币对美元汇率影响因子γ5>实际利用外资金额影响因子γ2>外汇储备影响因子γ4>城乡居民储蓄存款年底余额影响因子γ3。可见,对中国国际贸易安全性影响程度由大至小依次是x1,x6,x5,x2,x4和x3。其原因可能是:固定资产投资总额的增长能较大程度地促进中国国际贸易的发展,国内固定资产投资总额越大,越能刺激出口贸易增长,对中国国际贸易安全的影响也就越大。货币供应量可有效促进国内市场需求,从而在一定程度上促进进出口贸易增长,也会对中国国际贸易安全带来较大影响。人民币对美元汇率的影响不如固定资产投资总额x1、货币供应量x6的影响显著,其主要原因是人民币对美元汇率的变化会导致出口需求的变化,即对出口市场需重新选择,对美元升值可能会导致对贬值地区的出口量增加,因此,对中国国际贸易安全的变化影响不显著。
表5 中国国际贸易安全性指标参数xi
Table 5 Capability index parameters xi(i=1, 2, …, 5) for international trade safety in China
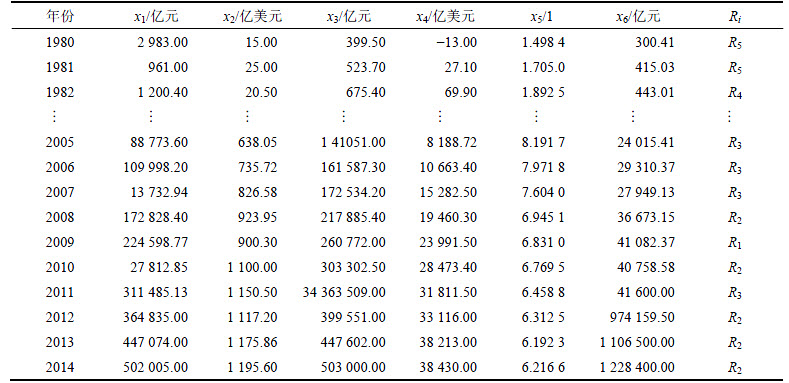
表6 基于中国国家贸易参数的IFLS-SVM分类辨识模型训练结果
Table 6 Training results of IFLS-SVM classification and identification model based on China international trade safety
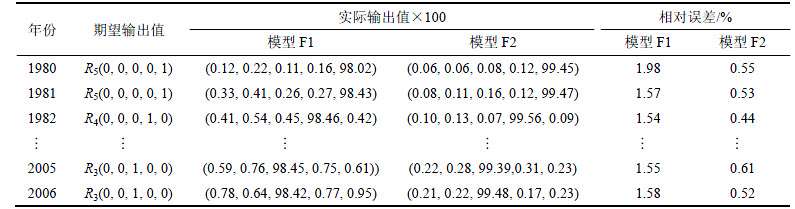
表7 训练后IFLS-SVM分类辨识模型测试结果
Table 7 Test results of IFLS-SVM classification and identification model after training
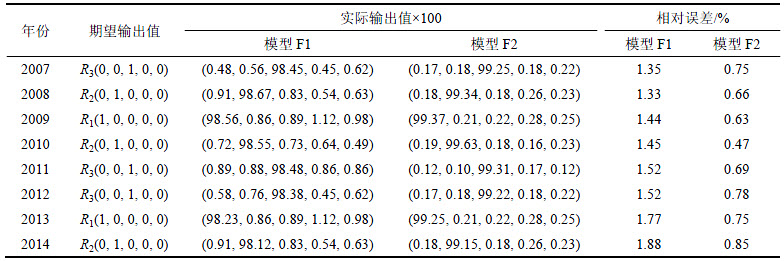
从1980—2014年中国国际贸易性辨析仿真分析实例可看出IFLS-SVM分类辨识模型具有很大的优势,为中国国际贸易安全性准确辨析提供了一种有效的解决方法。
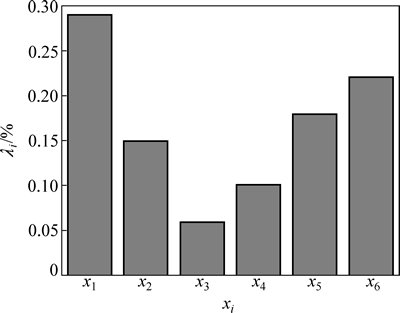
图4 中国国际贸易安全性指标参数影响因子
Fig. 4 Influence factors of capability index parameters about international trade safety in China
3 结论
1) 针对分类辨识数据的模糊特性,采用三角形隶属度函数法确定模糊最小二乘支持向量机隶属度,采用自适应变尺度混沌免疫算法优化FLS-SVM的参数,从而建立了IFLS-SVM分类辨识模型。IFLS-SVM分类辨识模型能够有效提高带噪声点和异常点数据集数据的辨析精度,其智能辨析相对误差小于0.8%,具有较高的预测精度。
2) LS-SVM分类辨识模型、FLS-SVM分类辨识模型和IFLS-SVM分类辨识模型的分类精度分别为82.67%,86.67%和90.67%。
3) 对中国国际贸易安全性影响由大至小依次是固定资产投资总额x1、货币供应量x6、人民币对美元汇率x5、实际利用外资金额x2、外汇储备x4和城乡居民储蓄存款年底余额x3。
参考文献:
[1] MONTEIRO R V A,
G C, MOURA F A M, et al. Estimating photovoltaic power generation: performance analysis of artificial neural networks, support vector machine and Kalman filter[J]. Electric Power Systems Research, 2017, 143: 643-656.
[2] COUELLAN N, WANG Wenjuan. Uncertainty-safe large scale support vector machines[J]. Computational Statistics & Data Analysis, 2017, 109: 215-230.
[3] ABD A M, ABD S M. Modelling the strength of lightweight foamed concrete using support vector machine(SVM)[J]. Case Studies in Construction Materials, 2017, 6: 8-15.
[4] LIU Chuan, WANG Wenyong, WANG Meng, et al. An efficient instance selection algorithm to reconstruct training set for support vector machine[J]. Knowledge-Based Systems, 2017, 116: 58-73.
[5] ZHANG Jun, OU Jianping, ZHAN Ronghui. Automatic target recognition of moving target based on empirical mode decomposition and genetic algorithm support vector[J]. Journal of Central South University, 2015, 22(4): 1389-1396.
[6] CARRIZOSA E,
A, MORALES D R. Clustering categories in support vector machines[J]. Omega, 2017, 66(Part A): 28-37.
[7] WANG Di, ZHANG Xiaoqin, FAN Mingyu, et al. Hierarchical mixing linear support vector machines for nonlinear classification[J]. Pattern Recognition, 2016, 59: 255-267.
[8] HANG Jun, ZHANG Jianzhong, CHENG Ming. Application of multi-class fuzzy support vector machine classifier for fault diagnosis of wind turbine[J]. Fuzzy Sets and Systems, 2016, 297: 128-140.
[9] ABE S. Fuzzy support vector machines for multilabel classification[J]. Pattern Recognition,2015, 48(6): 2110-2117.
[10]
S, et al. A new medical decision making system: Least square support vector machine (LSSVM) with fuzzy weighting pre-processing[J]. Expert Systems with Applications, 2007, 32(2): 409-414
[11] WANG Chunpeng, WANG Xingyuan, ZHANG Chuan, et al. Geometric correction based color image watermarking using fuzzy least squares support vector machine and Bessel K form distribution[J]. Signal Processing, 2017, 134: 197-208.
[12] 王志强, 李立君, 黄雁, 等. 基于模糊最小二乘支持向量机的火灾信号辨识[J]. 中南大学学报(自然科学版), 2013, 44(1): 202-207.
WANG Zhiqiang, LI Lijun, HUANG Yan, et al. Fire disaster signal recognition based on fuzzy least squares support vector machines[J]. Journal of Central SouthUniversity (Science andTechnology), 2013, 44(1): 202-207.
[13] 李岩波, 张超, 郭新辰. 基于直觉模糊最小二乘支持向量机的变压器故障诊断[J]. 吉林大学学报(理学版), 2014, 52(2): 313-318.
LI Yanbo, ZHANG Chao, GUO Xinchen. Transformer fault diagnosis based on intuitionistic fuzzy least squares support vector machine[J]. Journal of Jilin University (Science Edition), 2014, 52(2): 313-318.
[14] ZUO Hongyan, LUO Zhouquan, GUAN Jialin, et al. Identification on rock and soil parameters for vibration drilling rock in metal mine based on fuzzy least square support vector machine[J]. Journal of Central South University, 2014, 21(3): 1085-1090.
[15] WANG Taosheng, ZUO Hongyan. Fuzzy least squares support vector machines soft measurement model based on adaptive mutative scale chaos immune algorithm[J]. Journal of Central South University, 2014, 21(2): 593-599.
[16] VAPNIK V. Statistical learning theory[M]. New York: Wiley, 1998: 30-55.
[17] 鄂加强. 智能故障诊断及其应用[M]. 长沙: 湖南大学出版社, 2006: 100-145.
E Jiaqiang. Intelligent fault diagnosis and its application[M]. Changsha: Hunan University Press, 2006: 100-145.
[18] 彭海仔. 基于T-S模糊模型的太阳位置算法[J]. 上海海事大学学报, 2014, 35(2): 81-84.
PENG Haizai. Sun position algorithm based on T-S fuzzy model[J]. Journal of Shanghai Maritime University, 2014, 35(2): 81-84.
[19] 罗周全, 左红艳, 王益伟. 人-机-环境系统安全性的模糊熵评价方法[J]. 模糊系统与数学, 2011, 25(6): 169-174.
LUO Zhouquan, ZUO Hongyan, WANG Yiwei. Fuzzy entropy evaluation method of the safety for man-machine-environment system[J]. Fuzzy Systems and Mathematics, 2011, 25(6): 169-174.
[20] 左红艳. 机电产品出口贸易复杂性分析及其风险预警预报研究[M]. 长沙: 中南大学出版社, 2015: 140-141.
ZUO Hongyan. Study on the complexity of the export trade of electromechanical products and its risk prediction[M]. Changsha: Central SouthUniversity Press, 2015: 140-141.
(编辑 陈灿华)
收稿日期:2016-12-18;修回日期:2017-02-21
基金项目(Foundation item):国家自然科学基金资助项目(71573082);湖南省自然科学基金资助项目(2017JJ2134);湖南省高校创新平台开放基金资助项目(14K055)(Project(71573082) supported by the National Natural Science Foundation of China; Project(2017JJ2134) supported by the Natural Science Foundation of Hunan Province; Project(14K055) supported by the Innovation Platform Open Fund of Hunan Province)
通信作者:左红艳,博士(后),讲师,从事人工智能和非线性科学融合理论及其在国际贸易中的研究;E-mail:zuohongyan18@126.com