J. Cent. South Univ. Technol. (2011) 18: 1211-1216
DOI: 10.1007/s11771-011-0824-1
Knowledge mining collaborative DESVM correction method in short-term load forecasting
NIU Dong-xiao(牛东晓), WANG Jian-jun(王建军), LIU Jin-peng(刘金朋)
School of Economics and Management, North China Electric Power University, Beijing 102206, China
? Central South University Press and Springer-Verlag Berlin Heidelberg 2011
Abstract: Short-term forecasting is a difficult problem because of the influence of non-linear factors and irregular events. A novel short-term forecasting method named TIK was proposed, in which ARMA forecasting model was used to consider the load time series trend forecasting, intelligence forecasting DESVR model was applied to estimate the non-linear influence, and knowledge mining methods were applied to correct the errors caused by irregular events. In order to prove the effectiveness of the proposed model, an application of the daily maximum load forecasting was evaluated. The experimental results show that the DESVR model improves the mean absolute percentage error (MAPE) from 2.82% to 2.55%, and the knowledge rules can improve the MAPE from 2.55% to 2.30%. Compared with the single ARMA forecasting method and ARMA combined SVR forecasting method, it can be proved that TIK method gains the best performance in short-term load forecasting.
Key words: load forecasting; support vector regression; knowledge mining; ARMA; differential evolution
1 Introduction
Load forecasting is an important process in power systems. Short-term load forecasting (STLF) refers to several hours ahead or several days ahead load forecasting, and it has great significance for power market transactions, power flow optimization, units scheduling optimization and so on. Both electric utilities and its customers want to improve short-term forecasting accuracy in order to help them to get a good position in competition.
Many techniques have been proposed for short-term load forecasting in the last few decades. It can be divided into two classes: one is the traditional forecasting methods, and the other is the intelligence forecasting methods. The former mainly contains regression [1] and time series [2-4] forecasting method. However, traditional forecasting method ignores non-linear influences of other factors for STLF. It is difficult to improve the accuracy of STLF further.
Recently, with the artificial intelligence development, such as artificial neural networks (ANNs) [5-7], support vector machine (SVM) [8-11] and expert systems [12-13] methods have been successfully applied to STLF. The experimental results show that the performances of intelligence method are better than those of traditional methods. In the artificial intelligence forecasting method, ANN is the most favorite method in STLF. The reason is that ANN can consider other factors besides history load data. A three-layer ANN model can easily fit any nonlinear mapping between input variables and output variables, and it does not need too much prior knowledge of STLF. Furthermore, with support vector regression (SVR) method proposed by VAPNIK in 1998, SVR becomes a novel method for load forecasting, and it has been proved to achieve better performance than ANNs in related studies [8-11].
However, the STLF is a complex problem, because the influencing factors not only contain the economic or weather variables, but also contain social and human activities. The irregular changes of load are caused by non-parameter influences. How to further improve the forecasting accuracy is attracting the forecasting researchers.
In this work, a novel methodology integrated time series forecasting model, intelligence technology and knowledge (TIK) mining is proposed. This method can consider time series trend, non-linear parameters influence and irregularity influence integratedly, and its load forecasting accuracy is evaluated.
2 TIK method for short-term load forecasting
The frame of TIK method applied for STLF is shown in Fig.1. It can be divided into three modules: time series forecasting module, intelligence technology non-linear error correction module and knowledge influence rules module. The time series forecasting model forecasts time series trends, the intelligence part fits the errors caused by nonlinear behavior, and the knowledge mining technique corrects the errors caused by irregularity and infrequency events. Integrating above three modules can form a model for STLF problem. The details of each module are introduced as follows.
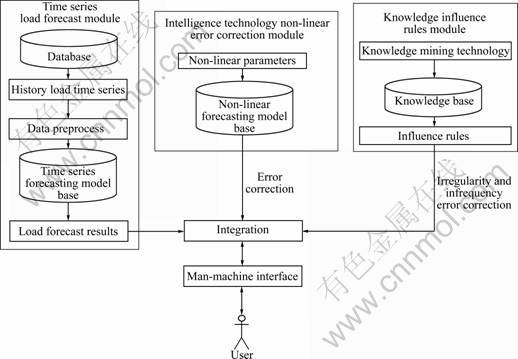
Fig.1 TIK method frame
2.1 Time series forecasting module
As seen in Fig.1, this module includes four processing procedures. Firstly, the history load time series are picked up from a database. Secondly, the data preprocess technologies are used to check the bad data and adjust the time series, and it also examines and removes the linear trend and seasons trend. Thirdly, the time series models are used in the load series forecasting. Finally, the primacy load forecasting result can be obtained for further analysis.
In this study, The ARMA method is applied in time series model forecasting procedure. ARMA model can be expressed by
(1)
where yt expresses the actual value at time t, B is the backward shift operator, and et is the random error, which is independently and identically distributed with a mean of zero and a constant variance of σ2.
f(B) and θ(B) can be calculated as
(2)
(3)
where p and q are integers, and the model is generally called ARMA(p, q). The values of p and q can be estimated by the difference time series characteristics of an autocorrelation function (ACF) and a partial autocorrelation function (PACF).
2.2 Intelligence technology non-linear error correction module
As mentioned above, time series load forecasting module can fit the load time series trend well, but it neglects the non-linear influence. Therefore, it is necessary to use a non-linear model technology in order to fit the non-linear components of load time series. The artificial intelligence technologies such as ANN and SVM method are competent for the problem. In this work, the support vector regression (SVR) in SVM method is used to capture the non-linear functions in order to correct the errors of ARMA.
The support vector machines (SVM) were proposed by VAPNIK, and the standard form of support vector regression (SVR) was proposed also by VAPNIK in 1998. The basic concept of the SVR is to map nonlinearly the original data x into a higher dimensional feature space. The standard form of SVR can be expressed as Eq.(4), and the dual is expressed as Eq.(5) [14]:
(4)
(5)
The Lagrange multipliers in Eq.(5) satisfy
0, and
are calculated and an optimal desired weight vector of the regression hyperplane is
(6)
Hence, the regression function is
(7)
where K(xi, xj) is called the kernel function. The value of the feature space ψ(xi) and ψ(xj), K(xi, xj)=ψ(xi)ψ(xj). In this work, the Gaussian RBF kernel function K(xi, x)= exp(-||xi-x||2/2σ2) is used as the kernel function.
From above description, using SVR models needs to determine three parameters C, v and σ. C controls the empirical risk degree of SVR, v controls the width of the fraction of errors and σ controls the Gaussian function width of the kernel function. Most of the researchers select three parameters by experience or some optimization algorithm such as SA [8] and GA [9]. In this work, differential evolution (DE) is used for determining the parameters of SVR forecasting method. A flowchart of DE algorithm for parameters selection of SVR model (DESVR) is shown in Fig.2.
Step 1: Initialization parameters. The populations (N), Chromosome length (D), the mutation factor (F) and the crossover rate (R) of DE algorithm should be determined at first, and a chromosome consists of three parameters C, v and σ, which should be determined in SVR. Therefore, the Chromosome length D=3. In this work, N=200, F=0.9 and R=0.9.
Step 2: Evolution starting. Set g=0 and employ Eq.(8) to generate the population randomly:
(8)
Step 3: Preliminary calculations. Input the generated population into SVR for load forecasting, then according to the load forecasting results, calculate and record the fitness function values. The fitness function is employed in the mean absolute percentage error function (MAPE, EMAP) which is common employed in load forecasting:
(9)
where A(i) is the actual value, F(i) is the forecasting value and n is the total numbers.
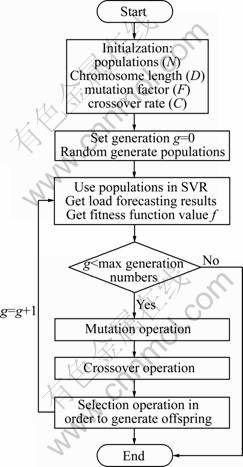
Fig.2 Flowchart of DESVR model
Step 4: Offspring generation. Employ Eq.(10), Eq.(11) and Eq.(12) to generate offspring, then input the offspring into SVR for load forecasting and calculate the fitness value again. Set g=g+1.
(10)
(11)
(12)
Step 5: Circulation until the stop criterion satisfied. If g equals the max generation number, show the best solution chromosome which is the best parameter of the SVR model, otherwise, go back to Step 3.
2.3 Knowledge influence rules module
The key to improve the load forecasting accuracy is to correct the irregular influence of the load time series. The above two modules can only fit the linear and non-linear influences of the factors. They cannot consider other influences by predictable irregular events, for example, both the special days and the special weather have the special load series patterns. It is necessary to extract some influence rules or knowledge by text mining technology or expert’s experience. In this work, the decision trees classification technology of data mining is used to form the IF-THEN rules which are used to correct the irregular errors of the forecasting results.
3 Application and analysis
The application load data are chosen from the daily maximum load from 2005-01-01 to 2007-12-31 of Jiangmen City, Guangdong Province, China. The total points are 1 095 noted by Yt as shown in Fig.3.
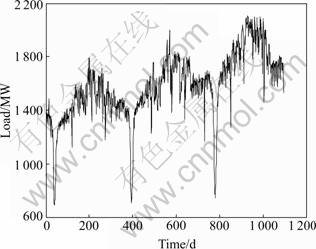
Fig.3 Time series form Jan 1st 2005 to Dec 31st 2007
The environment of experiment includes Matlab 2009a, libsvm 2.8.8 and data mining tools Weka 3.6. The computer with the Intel(R) Core(TM)2 T5600 1.8 GHz CPU, 1 G RAM and Windows XP professional system is used as the calculation environment. According to TIK method mentioned above, the experiment steps are as follows.
Step 1: Data preprocess. It can be clearly seen that the load time series shows the seasonal and the linear trend. At first, the 365 days’ seasonal trend of load time series is removed, and the linear trend is also removed by the least square linear regression method.
Step 2: ARMA forecasting. An ARMA(1,1) forecasting model of which parameters p and q are determined by AIC criterion is used to forecast the load time series trend preliminary.
Step 3: Using DESVR method to correct the nonlinear influence. According to forecasting result
by ARMA method, the error series Et can be obtained by
Then, the DESVR model with Et-1, Et-2, Et-3, Et-365, totally four input variables are used to fit the nonlinear influence. The data are divided into two parts: one is the training data set form Jan 1st, 2006 to Dec 31st, 2006, and the other is the test set from Jan 1st, 2007 to Dec 31st, 2007. Finally, the corrected errors series Et can be calculated.
Step 4: Irregular influence knowledge rules analysis. Combining the ARMA model result
and DESVR correction model result Et, the advanced forecasting result
can be calculated by
Then, the error ratio series αt can be obtained by
(13)
According to the previous description by knowledge influence rules module, the factors in Table 1 are prepared for forming the IF-THEN rules, and the αt processing strategies are shown in Table 2.
Table 1 Attributes used for knowledge mining
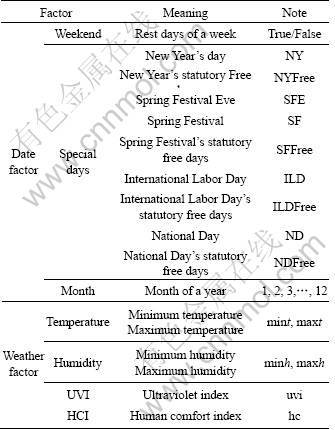
After a decision tree is formed by Weka software [15], the IF-THEN rules can be extracted as listed in Table 3.
Step 5: Integrate ARMA results, DESVR results and use IF-THEN rules to adjust the forecast results by Eq.(14):
(14)
In order to compare the result, the mean absolute percentage error (MAPE, EMAP) in Eq.(9) is used, and the result comparison is shown in Table 4.
Table 2 Preprocessing strategy of knowledge mining
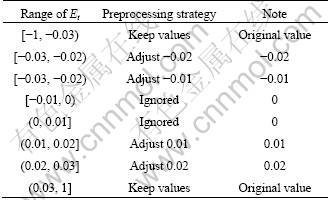
Table 3 Corrective IF-THEN rules
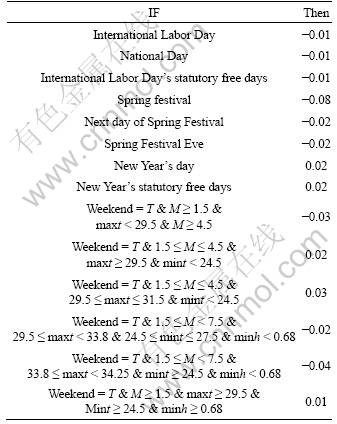
Table 4 MAPE values of three methods
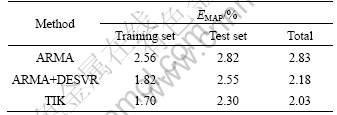
It can be clearly seen that the corrected forecasting results by DESVR is better than ARMA results, and the best performance forecasting result is obtained by the integrated TIK method, which has the lowest MAPE of all data set in the three methods. The main reason is that DESVR method adds the non-linear influence to the ARMA forecasting result, and the error correction can effectively improve the forecasting performance. Finally, the IF-THEN rules amend several larger error forecasting points, which makes the load forecasting result performance better. Figure 4 shows the results (total 61 points) corrected by IF-THEN rules in test set.
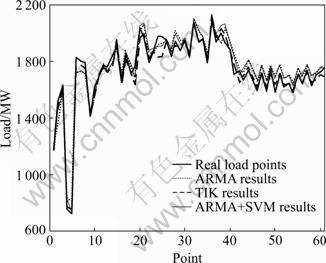
Fig.4 Results of ARMA results, ARMA combined DESVM method and the proposed TIK method
4 Conclusions
1) TIK method integrates the time series forecasting model, the non-linear intelligence forecasting model and knowledge mining method. It considers the load time series trend, non-linear influence and irregular influence together. It can improve the load forecasting performance.
2) The experimental results verify the effectiveness of the proposed method. The application results show that the non-linear intelligence SVR method produces a significant improvement in daily maximum load forecasting by correcting the ARMA result, and the knowledge rules adjustment can further improve the results outstanding. The TIK method has the best forecasting accuracy.
References
[1] GOIA A, MAY C, FUSAI G. Functional clustering and linear regression for peak load forecasting [J]. International Journal of Forecasting, 2010, 26(4): 700-711.
[2] PAPPAS S S, EKONOMOU L, KRAMOUSANTAS D C, CHATZARAKIS G E, KATSIKAS S K, LIATSIS P. Electricity demand loads modeling using auto regressive moving average (ARMA) models[J]. Energy, 2008, 33(9): 1353-1360.
[3] NOWICKA-ZAGRAJEK J, WERON R. Modeling electricity loads in California: ARMA models with hyperbolic noise [J]. Signal Processing, 2002, 82(12): 1993-1915.
[4] WANG Bo, TAI Neng-ling, ZHAI Hai-qing, YE Jian, ZHU Jia-dong, QI Liang-bo. A new ARMAX model based on evolutionary algorithm and particle swarm optimization for short-term load forecasting [J]. Electric Power Systems Research, 2009, 78(10): 1679-1685.
[5] KALAITZAKIS K, STAVRAKAKIS G S, ANAGNOSTAKIS E M. Short-term load forecasting based on artificial neural networks parallel implementation [J]. Electric Power Systems Research, 2002, 63(3): 185-196
[6] HSU Che-Chiang, CHEN Chia-Yon. Regional load forecasting in Taiwan—Applications of artificial neural networks [J]. Energy Conversion and Management, 2003, 44(12): 1941-1949.
[7] LI Cun-bin, WANG Ke-cheng. A new grey forecasting model based on BP neural network and Markov chain [J]. Journal of Central South University of Technology, 2007, 14(5): 713-718.
[8] PAI Ping-feng, HONG Wei-Chiang. Support vector machines with simulated annealing algorithms in electricity load forecasting [J]. Energy Conversion and Management, 2005, 46(17): 2669-2688.
[9] PAI Ping-Feng, HONG Wei-Chiang. Forecasting regional electricity load based on recurrent support vector machines with genetic algorithms [J]. Electric Power Systems Research, 2005, 74(3): 417-425.
[10] YANG Shu-xia. Study and application of time series forecasting based on rough set and Kernel method [J]. Journal of Central South University of Technology, 2008, 15(s2): 336-340.
[11] LI Yan-bin, ZHANG Ning, LI Cun-bin. Support vector machine forecasting method improved by chaotic particle swarm optimization and its application [J]. Journal of Central South University of Technology, 2009, 16(3): 336-340.
[12] KANDIL M S, EL-DEBEIKY S M, HASANIEN N E. The implementation of long-term forecasting strategies using a knowledge-based expert system (Part II) [J]. Electric Power Systems Research, 2001, 58(1): 19-25.
[13] MAMLOOK R, BADRAN O, ABDULHADI E. A fuzzy inference model for short-term load forecasting [J]. Energy Policy, 2008, 37(4): 1239-1248.
[14] HONG Wei-Chiang. Electric load forecasting by support vector model [J]. Applied Mathematical Modelling, 2009, 33(5): 2444- 2454.
[15] WITTEN I H, FRANK E. Data Mining: Practical machine learning tools and techniques [M]. San Francisco: Morgan Kaufmann, 2006.
(Edited by YANG Bing)
Foundation item: Projects(70671039, 71071052) supported by the National Natural Science Foundation of China; Projects(10QX44, 09QX68) supported by the Fundamental Research Funds for the Central Universities in China
Received date: 2010-04-09; Accepted date: 2010-11-20
Corresponding author: NIU Dong-xiao, Professor, PhD; Tel: +86-10-80796652; E-mail: niudx@126.com