基于改进蚁群算法优化参数的LSSVM短期负荷预测
龙文1, 2,梁昔明2,龙祖强3,李朝辉2
(1. 贵州财经学院 贵州省经济系统仿真重点实验室,贵州 贵阳,550004;
2. 中南大学 信息科学与工程学院,湖南 长沙,410083;
3. 衡阳师范学院 物理与电子信息科学系,湖南 衡阳,421008)
摘要:提出一种自动优选最小二乘支持向量机(LSSVM)模型参数的改进蚁群(MACO)算法。该算法将LSSVM模型的参数作为蚂蚁的位置向量,然后采用动态随机抽取的方法来确定目标个体引导蚁群进行全局搜索,同时在最优蚂蚁邻域内进行小步长局部搜索,找到模型的最优参数,得到基于MACO算法优化的LSSVM(MACO-LSSVM)预测模型。将优化后的LSSVM模型应用于短期电力负荷预测问题,选择湖南某地区日期为2009-08-01至 2009-08-30各小时点的数据进行分析,对2009-08-31该日24 h的负荷进行预测,并与BP神经网络和SVM模型进行比较。研究结果表明: 本文方法得到的均方根相对误差为1.71%,比用BP神经网络和SVM模型得到的均方根相对误差分别低1.61%和1.05%。
关键词:最小二乘支持向量机;蚁群优化算法;参数优化;短期负荷预测
中图分类号:TP273 文献标志码:A 文章编号:1672-7207(2011)11-3408-07
Parameters selection for LSSVM based on modified ant colony optimization in short-term load forecasting
LONG Wen1,2, LIANG Xi-ming2, LONG Zu-qiang3, LI Zhao-hui2
(1. Guizhou Key Laboratory of Economics System Simulation, Guizhou College of Finance and Economics,
Guiyang 550004, China;
2. School of Information Science and Engineering, Central South University, Changsha 410083, China;
3. Department of Physics and Electronic Information Science, Hengyang Normal College, Hengyang 421008, China)
Abstract: An optimization method based on the modified ant colony optimization (MACO) algorithm was used to select the two parameters of least square support vector machine (LSSVM) model. In this method, the parameters of LSSVM model were considered the position vector of ants. Target individuals which lead the ant colony to do global rapid search were determined by dynamic and stochastic extraction, and the optimal ant of this generation searched in small step nearly. The optimal parameter value was obtained by MACO and modified ant colony optimization-least square support vector machine (MACO-LSSVM) forecasting model was obtained. The proposed model is applied to the short-term electrical power load forecasting problem. Every hour’s load from 2009-08-01 to 2009-08-30 of area in Hunan province was taken as the sample data to be analyzed. The results indicate that the root-mean-square relative error of the proposed method is only 1.71%, which is less than those of BP and SVM model by 1.61% and 1.05%, respectively.
Key words: least square support vector machine (LSSVM); ant colony optimization (ACO) algorithm; parameter optimization; short-term load forecasting
电力系统负荷预测是指从电力负荷历史数据及其相关因素等资料出发,运用某种方法去合理推测未来某一时段的电力负荷需求情况。短期负荷预测是电力负荷预测的重要组成部分,准确的短期负荷预测可以维持电网安全运行,降低发电成本,提高电力系统的经济效益和社会效益[1]。传统的短期负荷预测方法有回归分析法、趋势外推法、灰色系统法和时间序列法等[2-3]。然而,影响短期电力负荷的因素大都具有不确定性和复杂性,并具有很强的非线性,传统的拟线性预测方法存在着局限性。
近年来,人工神经网络(ANN)在电力短期负荷预测中取得了一定的研究成果[4-5]。但ANN存在网络结构难以确定、过学习及易陷入局部极小值等缺点。基于统计理论的支持向量机(SVM)方法能够较好地解决小样本、非线性等问题,在电力负荷预测中被证明了优于人工神经网络和其他方法[6]。最小二乘支持向量机(LSSVM)在SVM的基础上用等式约束替代不等式约束,避免了求解耗时的二次规划问题[7]。LSSVM可以以任意精度逼近非线性系统,是非线性系统建模与预测的有力工具[8-9]。
由于LSSVM模型的推广预测能力很大程度上依赖于惩罚因子和核函数参数。因此,确定合适的参数是利用LSSVM进行建模和预测所要解决的关键问题。Chappelle等[10]提出用梯度下降法来优化参数,虽然在算法效率上有了明显的改善,但对初始点要求较高,且易陷入局部最优;张春晓等[11]采用遗传算法来优化LSSVM参数,虽然遗传算法不依赖于问题的数学模型,但其遗传操作比较复杂。由于人们对LSSVM模型参数优化的研究和方法不是很多,到目前为止,还没有得到指导LSSVM参数选择的好方法,也没有什么系统的规律可循。在实际应用中大多凭经验确定其参数,这可能导致由于参数选择不恰当而使其预测精度不高。鉴于此,本文作者通过改进的蚁群算法对最小二乘支持向量机模型的参数进行自动搜索和确定,然后建立改进蚁群算法优化参数的LSSVM (MACO-LSSVM)预测模型。最后将MACO-LSSVM模型应用到短期电力负荷预测中,取得了比较满意的 结果。
1 最小二乘支持向量机回归理论
支持向量机(SVM)的基本思想是通过一个非线性映射,把输入空间的数据映射到一个高维特征空间,将实际问题转化为一个带不等式约束的二次规划问题。LSSVM是SVM的一种扩展,将不等式约束替代等式约束,把误差平方和损失函数作为训练集的经验损失,从而把问题转化为一个线性矩阵求解问题。其具体原理如下:
给定一组样本数据集
,其中
是输入向
量,
,
是对应的输出,
。m为样本集大小。通过一个非线性函数φ将样本映射到高维空间,然后进行线性回归,回归函数为:
φ
(1)
其中:w为权值向量;b为偏差。利用LSSVM进行函数回归时优化目标为:
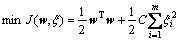
φ
(2)
其中:C为误差惩罚函数;
为松弛变量。构造拉格朗日函数L为:
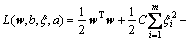
φ
(3)
其中:ai为拉格朗日乘子,根据KKT条件有:
(4)
消去w和
,可得出:
(5)
其中:Q=[1, …, 1]T;A=[a1, a2, …, am]T;Y=[y1, y2, …, ym]T。根据Mercer条件可以确定核函数为:
φ
φ
(6)
则LSSVM的函数估计为:
(7)
本文采用径向基函数(RBF)作为核函数:
(8)
其中:
为核函数宽度。
根据LSSVM回归理论可知:它的主要参数是核函数参数
和惩罚参数
,这2个参数对LSSVM的学习和泛化能力影响很大。本文采用改进的蚁群算法来优化选择2个参数
和C,在一定程度上减少了主观经验选择的盲目性。
2 基于MACO的LSSVM参数优化
2.1 改进的蚁群(MACO)算法
蚁群算法最初是针对离散优化问题而提出的一种智能算法[12]。因此,利用它求解连续优化问题时必须对算法的一些细节进行改进。假设考虑如下连续优化问题:
(9)
基本蚁群算法中蚂蚁的信息素体现在各个离散点之间的路径上。本文算法中蚁群是根据某个区域的信息素对该蚂蚁的影响来选择下一步行进方式,信息素依附于种群的个体上,表示该个体对蚂蚁的吸引程度,蚂蚁在引导个体寻优后将在该个体上释放信息素。
2.1.1 初始化蚁群位置和信息素
设蚁群规模为N,将蚁群随机分布在解空间,然后根据蚂蚁所在初始位置的分布情况,按照寻优问题的不同来确定蚂蚁i的初始信息素大小:
(10)
对于蚂蚁初始位置
,i=1, 2, …, N,当
≥0时,由式(10)可知:
,当
无限大时,信息素浓度将无限接近零,因此,应对适应度
进行修正[13]:
(11)
其中:avg为
的平均值,
;
为修正前的适应度值;
为修正后的值。
2.1.2 蚂蚁构建解
蚂蚁在完成一次搜索后,会根据相应的移动规则进行下一次搜索。本文算法的移动规则分为2个部 分[14]:一是通过动态随机抽取的方法选定目标个体,将上一次迭代中除最优蚂蚁以外的其他蚂蚁向目标个体移动,称其为全局大步长搜索;二是借鉴模式搜索中“探测”思想,让最优蚂蚁在邻域内进行小步长的局部精细搜索,以便找到更好的解。具体描述如下[14]:
移动规则1:首先随机抽取种群中p个体。其中:
,N为种群规模,r为动态变化的抽取比率,
,
为最大迭代次数,iter为当前迭代次数。接着计算出所抽取的个体中信息素浓度最大的个体作为目标个体
。
(12)
其中:Xbest为上次迭代中获得的最优解。这样做是由于依附于个体的信息素浓度越大,表示该个体对其他蚂蚁的吸引程度也就越大。蚂蚁在向目标个体移动的过程中,可能会找到更好的解,蚂蚁i按式(13)向目标蚂蚁位置移动:
(13)
蚂蚁按规则1进行移动使算法在迭代前期增大搜索的随机性,后期加快收敛速度[14]。
移动规则2:对上次迭代中获得最优解的蚂蚁Xbest,在其邻域内进行精细的局部搜索,搜索算法如式(14)和(15)所示。
(14)
(15)
其中:
,式中加减号的确定借鉴模式搜索中探测的思想,由下式进行判断:
(16)
若
≤
,则取“+”,否则取“-”。
h为动态搜索步长,按下式更新:
(17)
式中:hmax和hmin为初始设定的常数,一般取hmin= 0.1hmax。使用动态搜索步长的目的是随着搜索的进行,使搜索过程越来越细致,有利于提高解的精度[14]。
2.1.3 信息素更新策略
在完成全局搜索和局部搜索后,对蚂蚁i处的信息素
进行更新,更新规则为
(18)
其中:
为信息素挥发系数,
。
2.2 基于MACO的LSSVM参数优化
定义目标函数为:
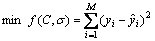
(19)
其中:yi为第i个已知样本的输出值;
为第i个样本的模型预测输出值,可由式(7)计算得到。
LSSVM参数优化的思想是通过迭代算法搜索一组参数
,使目标函数(19)达到最小。本文利用2.1节中所描述的改进蚁群算法(MACO)来进行优化,将定义域内一组参数序列
作为MACO中蚂蚁的位置向量,各蚂蚁的适应度函数取式(19)。算法具体步骤如下:
Step1 根据收集到的历史数据,建立训练样本集和测试样本集。
Step2 设置参数,初始化蚂蚁位置,每个位置值对应于LSSVM模型的一组参数
,由参数和样本训练建立如式(7)所示的LSSVM预测模型。
Step3 由式(19)计算每个蚂蚁个体的适应度值。再通过式(10)计算各只蚂蚁的信息素浓度。
Step4在种群中随机抽取p只蚂蚁,根据每只蚂蚁所在位置的信息素浓度大小,找出最优蚂蚁的位置设为Xbest,把它作为目标个体Xobj。
Step5 种群中非最优蚂蚁按式(13)向目标蚂蚁位置移动进行全局搜索。
Step6 最优蚂蚁按2.1.2节中的移动规则2在其邻域内进行局部搜索。
Step7 按式(18)更新每只蚂蚁的信息素浓度。
Step8 判断是否满足迭代终止条件,若满足,则迭代结束输出最优参数
;否则,返回step4。
Step9 利用最优参数
和训练样本训练建立MACO-LSSVM预测模型。
3 基于MACO-LSSVM的短期负荷预测
首先选取3个标准测试函数对MACO算法的性能进行测试,函数具体表达式见文献[13]和文献[14]。为了方便比较,本文算法参数和文献中相关参数设置一样,蚁群规模N=20,最大迭代数均为1 000次。对每个测试函数均独立运行10次。表1给出了几种算法对3个函数的实验结果比较。当寻优结果达到收敛精度时,认为寻优成功,收敛精度取1.0×10-4。表1中达优率表示满足收敛精度的寻优结果占实验所有结果的比例。
从表1可知:对于函数f1和f2,MACO的求解精度要优化对比的算法,且函数估值次数相对较少。而对于函数f3,本文算法在达优率和收敛速度上有一定的优势。因此,与文献中算法相比,MACO有较快的收敛速度和优良的全局寻优能力,且求解精度有了很大的提高。
为了验证MACO-LSSVM模型的预测性能,本文选取湖南省某地区电网从2009-08-01至2009-08-30各日0~24点的负荷数据作为基础数据,利用本文提出的模型对该地区2009-08-31该日24个整点时刻的负荷进行仿真预测。并与BP神经网络模型和SVM模型的预测结果进行比较。为了减少负荷预测的波动性,提高预测精度,有必要将历史负荷数据按工作日和周末日分成工作日基础数据和周末日基础数据。同时考虑到短期负荷的日周期性,对预测日的每个时刻单独建模,所以,该日的预测是重复24次建模完成的。在对每个时刻的建模过程中,利用负荷数据序列中最靠近预测日该时刻前4 d的同一时刻值作为LSSVM模型的输入向量,预测日同一时刻的实际值作为LSSVM模型的输出,这样就构成了LSSVM建模的输入输出数据样本对。
表1 几种算法对3个测试函数结果比较
Table 1 Results of different algorithms on three functions
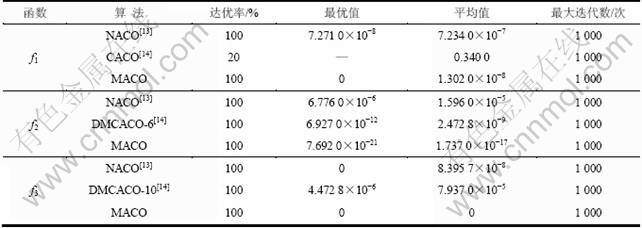
本文方法中的参数设置为: 蚁群规模为N=10,最大迭代次数为iter max=100,惩罚误差
,核函数参数
。BP神经网络的结构采用4-5-1,学习因子
,最大允许误差为
。SVM模型的惩罚误差C=100,核参数
。通过MACO优化第10个整点时刻负荷LSSVM模型的2个参数
和
。图1所示为利用MACO对第10个整点时刻负荷LSSVM预测模型参数寻优的曲线。
本文利用相对误差ER和均方根相对误差RMSRE作为评价指标[15]:
(20)
(21)
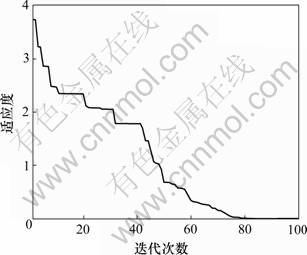
图1 MACO对LSSVM参数寻优曲线
Fig.1 Optimization curve for LSSVM parameters by MACO
图2和表2给出了本文方法(MACO-LSSVM)、BP神经网络和SVM模型对2009-08-31该日24个整点负荷预测的仿真结果。图3所示为不同模型的相对误差。表中的负荷实际值(Actual values)和预测值(Forecasting values)分别用AV和FV表示。
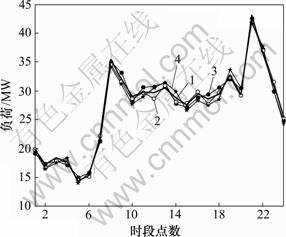
图2 不同模型的预测结果
Fig.2 Forecasting results with different models
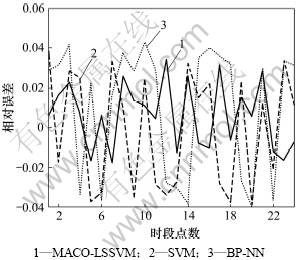
图3 不同模型的相对误差
Fig.3 Relative errors with different models
从图2,3和表2可知:MACO-LSSVM预测模型24个时段点中有22个时段点的相对误差的绝对值小于3%,另外2个时段点的相对误差均接近3%,这充分说明MACO-LSSVM模型具有较高的预测精度。对于SVM预测模型,有9个时段点的相对误差的绝对值在3%以上,而其他15个时段点中,时段点2,8,15,21和24的相对误差绝对值低于2%,其余时段点的均在2%~3%之间。而BP神经网络模型的预测结果中,只有7个时段点的相对误差绝对值在3%以内,这说明BP神经网络模型的预测精度较低。
另外,从表2可以看出:MACO-LSSVM方法得到的均方根相对误差仅为1.71%,比用SVM模型和BP神经网络模型得到的均方根相对误差分别低1.05%和1.61%。基于以上分析可知:本文提出的MACO-LSSVM预测模型比SVM模型和BP神经网络具有更高的预测精度。
表2 仿真预测结果及相对误差
Table 2 Simulation forecasting results and relative errors
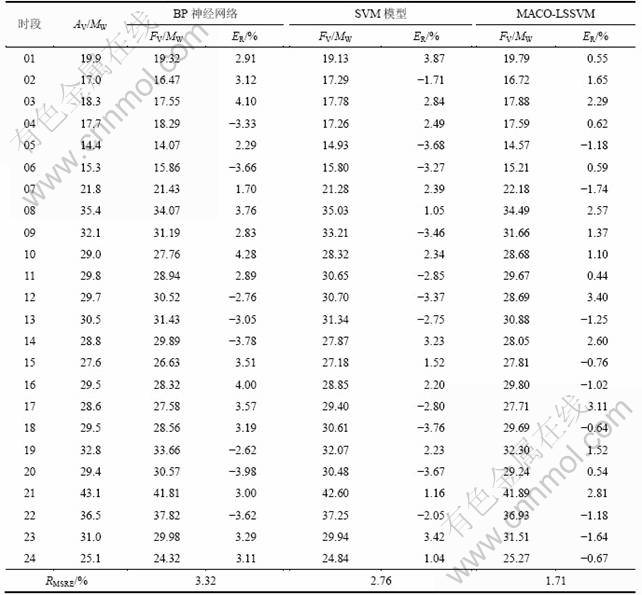
4 结论
(1) 在利用最小二乘支持向量机进行预测时,参数的选取很重要,若参数选取不合理,则直接影响其预测精度。
(2) 本文提出利用改进蚁群算法对最小二乘支持向量机的参数进行寻优,以得到使LSSVM预测精度最高的一组最优参数。
(3) 通过对某地区的短期负荷预测应用结果表明,本文所提出的方法具有较好的预测效果。
参考文献:
[1] 康重庆, 夏清, 刘梅. 电力系统负荷预测[M]. 北京: 中国电力出版社, 2007: 1-20.
KONG Chong-qing, XIA Qing, LIU Mei. Power system load forecasting[M]. Beijing: China Electric Power Press, 2007: 1-20.
[2] 张俊芳, 吴伊昂, 吴军基. 基于灰色系统理论负荷预测的应用研究[J]. 电力自动化设备, 2004, 24(5): 24-27.
ZHANG Jun-fang, WU Yi-ang, WU Jun-ji. Application of grey system theory in load forecasting[J]. Electric Power Automation Equipment, 2004, 24(5): 24-27.
[3] 李钷, 李敏, 刘涤尘. 基于改进回归法的电力负荷预测[J]. 电网技术, 2006, 30(1): 99-104.
LI Po, LI Min, LIU Di-chen. Power load forecasting based on improved regression[J]. Power System Technology, 2006, 30(1): 99-104.
[4] 李彦斌, 李存斌, 宋晓华. 改进的人工智能神经网络预测模型及其应用[J]. 中南大学学报: 自然科学版, 2008, 39(5): 1054-1058.
LI Yan-bin, LI Cun-bin, SONG Xiao-hua. Prediction model of improved artificial neural network and its application[J]. Journal of Central South University: Science and Technology, 2008, 39(5): 1054-1058.
[5] Kandil N, Wamkeue R, Saad M. An efficient approach for short term load forecasting using artificial neural networks[J]. International Journal of Electrical Power and Energy Systems, 2006, 28: 525-530.
[6] Chen B J, Chang M W, Lin C J. Load forecasting using support vector machine: a study on EUNITE competition 2001[J]. IEEE Transactions on Power Systems, 2004, 19(4): 1821-1830.
[7] Suykens J A K, Vandewalle J. Least square support machine classifier[J]. Neural Processing Letters, 1999, 9(3): 293-300.
[8] Esen H, Ozgen F, Esen M. Modeling of a new solar air heater through least square support vector machine[J]. Expert Systems with Applications, 2009, 36(7): 10673-10682.
[9] 耿艳, 韩学山, 韩力. 基于最小二乘支持向量机的短期负荷预测[J]. 电网技术, 2008, 32(18): 72-76.
GENG Yan, HAN Xue-shan, HAN Li. Short-term load forecasting based on least squares support vector machine[J]. Power System Technology, 2008, 32(18): 72-76.
[10] Chappelle O, Vapnik V, Bousquet O. Choosing multiple parameters for support vector machines[J]. Machine Learning, 2002, 46(1): 131-160.
[11] 张春晓, 张涛. 基于最小二乘支持向量机和遗传算法的热式油水两相流含油率建模[J]. 化工学报, 2009, 60(7): 1651-1655.
ZHANG Chun-xiao, ZHANG Tao. Oil holdup modeling of oil-water two-phase flow using thermal method based on LSSVM and GA[J]. CIESC Journal, 2009, 60(7): 1651-1655.
[12] Dorigo M, Maniezzo V, Colorni A. Ant system: Optimization by a colony of cooperating agents[J]. IEEE Transaction on Systems, Man, and Cybernetics, 1996, 26(1): 29-41.
[13] 马卫, 朱庆保. 求解函数优化问题的快速连续蚁群算法[J]. 电子学报, 2008, 36(11): 2120-2124.
MA Wei, ZHU Qing-bao. Fast continuous ant colony optimization algorithm for solving function optimization problems[J]. Acta Electronica Sinica, 2008, 36(11): 2120-2124.
[14] 梁昔明, 李朝辉, 龙文, 等. 含维变异算子的连续域蚁群算法[J]. 计算机应用, 2010, 30(12): 3204-3206.
LIANG Xi-ming, LI Zhao-hui, LONG Wen, et al. Continuous domains ant colony algorithm with dimension mutation operator[J]. Journal of Computer Applications, 2010, 30(12): 3204-3206.
[15] 吴景龙, 杨淑霞, 刘承水. 基于遗传算法优化参数的支持向量机短期负荷预测方法[J]. 中南大学学报: 自然科学版, 2009, 40(1): 180-184.
WU Jing-long, YANG Shu-xia, LIU Cheng-shui. Parameter selection for support vector machines based on genetic algorithms to short-term power load forecasting[J]. Journal of Central South University: Science and Technology, 2009, 40(1): 180-184.
(编辑 陈爱华)
收稿日期:2010-07-12;修回日期:2010-12-06
基金项目:国家自然科学基金资助项目(60874070,61074069);贵州财经学院博士科研启动基金资助项目
通信作者:龙文(1977-),男,湖南隆回人,博士研究生,从事建模与智能优化研究;电话:13639086822;E-mail: lw084601012@gmail.com